Open Access
ARTICLE
Sports Events Recognition Using Multi Features and Deep Belief Network
1 Department of Information Systems, College of Computer and Information Sciences, Princess Nourah bint Abdulrahman University, P.O. Box 84428, Riyadh, 11671, Saudi Arabia
2 Department of Computer Science, Air University, Islamabad, 44000, Pakistan
3 Department of Computer Science, Applied College, King Khalid University, Abha, 61421, Saudi Arabia
4 Department of Computer Science, College of Computer Science and Information System, Najran University, Najran, 55461, Saudi Arabia
5 Department of Computer Sciences, Faculty of Computing and Information Technology, Northern Border University, Rafha, 91911, Saudi Arabia
6 Department of Computer Engineering, Korea Polytechnic University, Siheung-si, Gyeonggi-do, 237, Republic of Korea
* Corresponding Author: Jeongmin Park. Email:
(This article belongs to the Special Issue: Metaheuristics, Soft Computing, and Machine Learning in Image Processing and Computer Vision)
Computers, Materials & Continua 2024, 81(1), 309-326. https://doi.org/10.32604/cmc.2024.053538
Received 03 May 2024; Accepted 24 July 2024; Issue published 15 October 2024
Abstract
In the modern era of a growing population, it is arduous for humans to monitor every aspect of sports, events occurring around us, and scenarios or conditions. This recognition of different types of sports and events has increasingly incorporated the use of machine learning and artificial intelligence. This research focuses on detecting and recognizing events in sequential photos characterized by several factors, including the size, location, and position of people’s body parts in those pictures, and the influence around those people. Common approaches utilized, here are feature descriptors such as MSER (Maximally Stable Extremal Regions), SIFT (Scale-Invariant Feature Transform), and DOF (degree of freedom) between the joint points are applied to the skeleton points. Moreover, for the same purposes, other features such as BRISK (Binary Robust Invariant Scalable Keypoints), ORB (Oriented FAST and Rotated BRIEF), and HOG (Histogram of Oriented Gradients) are applied on full body or silhouettes. The integration of these techniques increases the discriminative nature of characteristics retrieved in the identification process of the event, hence improving the efficiency and reliability of the entire procedure. These extracted features are passed to the early fusion and DBscan for feature fusion and optimization. Then deep belief, network is employed for recognition. Experimental results demonstrate a separate experiment’s detection average recognition rate of 87% in the HMDB51 video database and 89% in the YouTube database, showing a better perspective than the current methods in sports and event identification.Keywords
Cite This Article
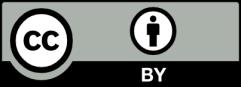
This work is licensed under a Creative Commons Attribution 4.0 International License , which permits unrestricted use, distribution, and reproduction in any medium, provided the original work is properly cited.