Open Access
ARTICLE
EfficientNetB1 Deep Learning Model for Microscopic Lung Cancer Lesion Detection and Classification Using Histopathological Images
1 Department of Computer Science, Lahore College for Women University, Lahore, 54000, Pakistan
2 Artificial Intelligence & Data Analytics Lab., CCIS Prince Sultan University, Riyadh, 11586, Saudi Arabia
3 Department of Information Systems, College of Computer and Information Sciences, Princess Nourah bint Abdulrahman University, P.O. Box 84428, Riyadh, 11671, Saudi Arabia
4 Department of Computer Science, College of Computer and Information Sciences, King Saud University, Riyadh, 145111, Saudi Arabia
* Corresponding Author: Tahani Jaser Alahmadi. Email:
(This article belongs to the Special Issue: Medical Imaging Based Disease Diagnosis Using AI)
Computers, Materials & Continua 2024, 81(1), 809-825. https://doi.org/10.32604/cmc.2024.052755
Received 14 April 2024; Accepted 23 August 2024; Issue published 15 October 2024
Abstract
Cancer poses a significant threat due to its aggressive nature, potential for widespread metastasis, and inherent heterogeneity, which often leads to resistance to chemotherapy. Lung cancer ranks among the most prevalent forms of cancer worldwide, affecting individuals of all genders. Timely and accurate lung cancer detection is critical for improving cancer patients’ treatment outcomes and survival rates. Screening examinations for lung cancer detection, however, frequently fall short of detecting small polyps and cancers. To address these limitations, computer-aided techniques for lung cancer detection prove to be invaluable resources for both healthcare practitioners and patients alike. This research implements an enhanced EfficientNetB1 deep learning model for accurate detection and classification using histopathological images. The proposed technique accurately classifies the histopathological images into three distinct classes: (1) no cancer (benign), (2) adenocarcinomas, and (3) squamous cell carcinomas. We evaluated the performance of the proposed technique using the histopathological (LC25000) lung dataset. The preprocessing steps, such as image resizing and augmentation, are followed by loading a pretrained model and applying transfer learning. The dataset is then split into training and validation sets, with fine-tuning and retraining performed on the training dataset. The model’s performance is evaluated on the validation dataset, and the results of lung cancer detection and classification into three classes are obtained. The study’s findings show that an enhanced model achieves exceptional classification accuracy of 99.8%.Keywords
Cite This Article
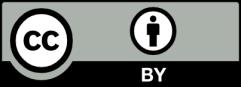
This work is licensed under a Creative Commons Attribution 4.0 International License , which permits unrestricted use, distribution, and reproduction in any medium, provided the original work is properly cited.