Open Access
ARTICLE
Enhancing Early Detection of Lung Cancer through Advanced Image Processing Techniques and Deep Learning Architectures for CT Scans
1 Computers and Systems Department, Electronics Research Institute, El Nozha, Huckstep Cairo, 12622, Egypt
2 Department of Electronics and Electrical Communications Engineering, Faculty of Electronic Engineering, Menoufia University, Menouf, 32952, Egypt
3 Security Engineering Lab, Computer Science Department, Prince Sultan University, Riyadh, 11586, Saudi Arabia
4 Department of Information Technology, College of Computer and Information Sciences, Princess Nourah bint Abdulrahman University, Riyadh, 84428, Saudi Arabia
* Corresponding Author: Nahed Tawfik. Email:
(This article belongs to the Special Issue: Data and Image Processing in Intelligent Information Systems)
Computers, Materials & Continua 2024, 81(1), 271-307. https://doi.org/10.32604/cmc.2024.052404
Received 01 April 2024; Accepted 09 August 2024; Issue published 15 October 2024
Abstract
Lung cancer remains a major concern in modern oncology due to its high mortality rates and multifaceted origins, including hereditary factors and various clinical changes. It stands as the deadliest type of cancer and a significant cause of cancer-related deaths globally. Early diagnosis enables healthcare providers to administer appropriate treatment measures promptly and accurately, leading to improved prognosis and higher survival rates. The significant increase in both the incidence and mortality rates of lung cancer, particularly its ranking as the second most prevalent cancer among women worldwide, underscores the need for comprehensive research into efficient screening methods. Advances in diagnostic techniques, particularly the use of computed tomography (CT) scans, have revolutionized the identification of lung cancer. CT scans are renowned for their ability to provide high-resolution images and are particularly effective in detecting small, calcified areas, crucial for identifying early-stage lung cancer. Consequently, there is growing interest in enhancing computer-aided detection (CAD) systems. These algorithms assist radiologists by reducing false-positive interpretations and improving the accuracy of early cancer diagnosis. This study aims to enhance the effectiveness of CAD systems through various methods. Initially, the Contrast Limited Adaptive Histogram Equalization (CLAHE) algorithm is employed to preprocess CT scan images, thereby improving their visual quality. Further refinement is achieved by integrating different optimization strategies with the CLAHE method. The CutMix data augmentation technique is applied to boost the performance of the proposed model. A comparative analysis is conducted using deep learning architectures such as Inception-V3, ResNet101, Xception, and EfficientNet. The study evaluates the performance of these architectures in image classification tasks, both with and without the implementation of the CLAHE algorithm. The empirical findings of the study demonstrate a significant reduction in the false positive rate (FPR) and an overall enhancement in diagnostic accuracy. This research not only contributes to the field of medical imaging but also holds significant implications for the early detection and treatment of lung cancer, ultimately aiming to reduce its mortality rates.Keywords
Cite This Article
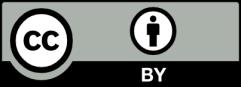
This work is licensed under a Creative Commons Attribution 4.0 International License , which permits unrestricted use, distribution, and reproduction in any medium, provided the original work is properly cited.