Open Access
ARTICLE
Improving Multiple Sclerosis Disease Prediction Using Hybrid Deep Learning Model
1 Department of Electrical and Computer Engineering, College of Engineering, Anderson University, Anderson, SC 29621, USA
2 Faculty of Computing and Information, Al-Baha University, Al-Baha, 65528, Saudi Arabia
3 ReDCAD Laboratory, University of Sfax, Sfax, 3038, Tunisia
4 Department of Information Technology, College of Computer & Information Science, Princess Nourah Bint Abdul Rahman University, Riyadh, 11564, Saudi Arabia
5 Department of Management Information Systems, College of Business and Economics, Qassim University, P.O. Box 6640, Buraidah, 51452, Saudi Arabia
6 School of Management and Information Technology, De La Salle-College of Saint Benilde, Manila, 1004, Philippines
7 Department of Information Management and Business Systems, Faculty of Management, Comenius University Bratislava, Bratislava 25, 82005, Slovakia
* Corresponding Authors: Moez Krichen. Email: ; Jaroslava Kniezova. Email:
Computers, Materials & Continua 2024, 81(1), 643-661. https://doi.org/10.32604/cmc.2024.052147
Received 25 March 2024; Accepted 31 July 2024; Issue published 15 October 2024
Abstract
Myelin damage and a wide range of symptoms are caused by the immune system targeting the central nervous system in Multiple Sclerosis (MS), a chronic autoimmune neurological condition. It disrupts signals between the brain and body, causing symptoms including tiredness, muscle weakness, and difficulty with memory and balance. Traditional methods for detecting MS are less precise and time-consuming, which is a major gap in addressing this problem. This gap has motivated the investigation of new methods to improve MS detection consistency and accuracy. This paper proposed a novel approach named FAD consisting of Deep Neural Network (DNN) fused with an Artificial Neural Network (ANN) to detect MS with more efficiency and accuracy, utilizing regularization and combat over-fitting. We use gene expression data for MS research in the GEO GSE17048 dataset. The dataset is preprocessed by performing encoding, standardization using min-max-scaler, and feature selection using Recursive Feature Elimination with Cross-Validation (RFECV) to optimize and refine the dataset. Meanwhile, for experimenting with the dataset, another deep-learning hybrid model is integrated with different ML models, including Random Forest (RF), Gradient Boosting (GB), XGBoost (XGB), K-Nearest Neighbors (KNN) and Decision Tree (DT). Results reveal that FAD performed exceptionally well on the dataset, which was evident with an accuracy of 96.55% and an F1-score of 96.71%. The use of the proposed FAD approach helps in achieving remarkable results with better accuracy than previous studies.Keywords
Cite This Article
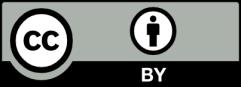
This work is licensed under a Creative Commons Attribution 4.0 International License , which permits unrestricted use, distribution, and reproduction in any medium, provided the original work is properly cited.