Open Access
ARTICLE
PSMFNet: Lightweight Partial Separation and Multiscale Fusion Network for Image Super-Resolution
1 Institute of Intelligent Manufacturing, GDAS, Guangdong Key Laboratory of Modern Control Technology, Guangzhou, 510030, China
2 School of Mechanical & Automotive Engineering, South China University of Technology, Guangzhou, 511442, China
3 Faculty of Information Engineering and Automation, Kunming University of Science and Technology , Kunming, 650500, China
4 School of Faculty of Intelligent Manufacturing, Wuyi University, Jiangmen, 529020, China
* Corresponding Author: Jianan Liang. Email:
Computers, Materials & Continua 2024, 81(1), 1491-1509. https://doi.org/10.32604/cmc.2024.049314
Received 03 January 2024; Accepted 07 April 2024; Issue published 15 October 2024
Abstract
The employment of deep convolutional neural networks has recently contributed to significant progress in single image super-resolution (SISR) research. However, the high computational demands of most SR techniques hinder their applicability to edge devices, despite their satisfactory reconstruction performance. These methods commonly use standard convolutions, which increase the convolutional operation cost of the model. In this paper, a lightweight Partial Separation and Multiscale Fusion Network (PSMFNet) is proposed to alleviate this problem. Specifically, this paper introduces partial convolution (PConv), which reduces the redundant convolution operations throughout the model by separating some of the features of an image while retaining features useful for image reconstruction. Additionally, it is worth noting that the existing methods have not fully utilized the rich feature information, leading to information loss, which reduces the ability to learn feature representations. Inspired by self-attention, this paper develops a multiscale feature fusion block (MFFB), which can better utilize the non-local features of an image. MFFB can learn long-range dependencies from the spatial dimension and extract features from the channel dimension, thereby obtaining more comprehensive and rich feature information. As the role of the MFFB is to capture rich global features, this paper further introduces an efficient inverted residual block (EIRB) to supplement the local feature extraction ability of PSMFNet. A comprehensive analysis of the experimental results shows that PSMFNet maintains a better performance with fewer parameters than the state-of-the-art models.Keywords
Cite This Article
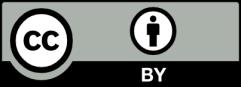
This work is licensed under a Creative Commons Attribution 4.0 International License , which permits unrestricted use, distribution, and reproduction in any medium, provided the original work is properly cited.