Open Access
ARTICLE
YOLO-RLC: An Advanced Target-Detection Algorithm for Surface Defects of Printed Circuit Boards Based on YOLOv5
1 School of Computer and Software Engineering, Huaiyin Institute of Technology, Huai’an, 223003, China
2 Huaiyin Institute of Technology, Laboratory for Internet of Things and Mobile Internet Technology of Jiangsu Province, Huai’an, 223001, China
3 Jiangsu Kesheng Xuanyi Technology Co., Ltd., Huai’an, 223300, China
* Corresponding Author: Yuanyuan Wang. Email:
Computers, Materials & Continua 2024, 80(3), 4973-4995. https://doi.org/10.32604/cmc.2024.055839
Received 08 July 2024; Accepted 22 August 2024; Issue published 12 September 2024
Abstract
Printed circuit boards (PCBs) provide stable connections between electronic components. However, defective printed circuit boards may cause the entire equipment system to malfunction, resulting in incalculable losses. Therefore, it is crucial to detect defective printed circuit boards during the generation process. Traditional detection methods have low accuracy in detecting subtle defects in complex background environments. In order to improve the detection accuracy of surface defects on industrial printed circuit boards, this paper proposes a residual large kernel network based on YOLOv5 (You Only Look Once version 5) for PCBs surface defect detection, called YOLO-RLC (You Only Look Once-Residual Large Kernel). Build a deep large kernel backbone to expand the effective field of view, capture global information more efficiently, and use 1 1 convolutions to balance the depth of the model, improving feature extraction efficiency through reparameterization methods. The neck network introduces a bidirectional weighted feature fusion network, combined with a brand-new noise filter and feature enhancement extractor, to eliminate noise information generated by information fusion and recalibrate information from different channels to improve the quality of deep features. Simplify the aspect ratio of the bounding box to alleviate the issue of specificity values. After training and testing on the PCB defect dataset, our method achieved an average accuracy of 97.3% (mAP50) after multiple experiments, which is 4.1% higher than YOLOv5-S, with an average accuracy of 97.6% and an Frames Per Second of 76.7. The comparative analysis also proves the superior performance and feasibility of YOLO-RLC in PCB defect detection.Keywords
Cite This Article
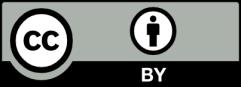
This work is licensed under a Creative Commons Attribution 4.0 International License , which permits unrestricted use, distribution, and reproduction in any medium, provided the original work is properly cited.