Open Access
ARTICLE
Service Function Chain Deployment Algorithm Based on Multi-Agent Deep Reinforcement Learning
1 College of Software Engineering, Zhengzhou University of Light Industry, Zhengzhou, 450007, China
2 Henan Jiuyu Tenglong Information engineering Co., Ltd., Zhengzhou, 450052, China
3 Henan Xin’an Communication Technology Co., Ltd., Zhengzhou, 450007, China
* Corresponding Author: Wanwei Huang. Email:
Computers, Materials & Continua 2024, 80(3), 4875-4893. https://doi.org/10.32604/cmc.2024.055622
Received 02 July 2024; Accepted 12 August 2024; Issue published 12 September 2024
Abstract
Aiming at the rapid growth of network services, which leads to the problems of long service request processing time and high deployment cost in the deployment of network function virtualization service function chain (SFC) under 5G networks, this paper proposes a multi-agent deep deterministic policy gradient optimization algorithm for SFC deployment (MADDPG-SD). Initially, an optimization model is devised to enhance the request acceptance rate, minimizing the latency and deploying the cost SFC is constructed for the network resource-constrained case. Subsequently, we model the dynamic problem as a Markov decision process (MDP), facilitating adaptation to the evolving states of network resources. Finally, by allocating SFCs to different agents and adopting a collaborative deployment strategy, each agent aims to maximize the request acceptance rate or minimize latency and costs. These agents learn strategies from historical data of virtual network functions in SFCs to guide server node selection, and achieve approximately optimal SFC deployment strategies through a cooperative framework of centralized training and distributed execution. Experimental simulation results indicate that the proposed method, while simultaneously meeting performance requirements and resource capacity constraints, has effectively increased the acceptance rate of requests compared to the comparative algorithms, reducing the end-to-end latency by 4.942% and the deployment cost by 8.045%.Keywords
Cite This Article
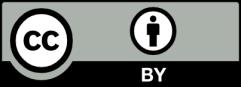
This work is licensed under a Creative Commons Attribution 4.0 International License , which permits unrestricted use, distribution, and reproduction in any medium, provided the original work is properly cited.