Open Access
ARTICLE
Q-Learning-Assisted Meta-Heuristics for Scheduling Distributed Hybrid Flow Shop Problems
1 Institute of Systems Engineering, Macau University of Science and Technology, Macau, 99078, China
2 Department of Electronics and Computer Science, Koszalin University of Technology, Koszalin, 75-453, Poland
* Corresponding Author: Kaizhou Gao. Email:
(This article belongs to the Special Issue: Recent Advances in Ensemble Framework of Meta-heuristics and Machine Learning: Methods and Applications)
Computers, Materials & Continua 2024, 80(3), 3573-3589. https://doi.org/10.32604/cmc.2024.055244
Received 21 June 2024; Accepted 13 August 2024; Issue published 12 September 2024
Abstract
The flow shop scheduling problem is important for the manufacturing industry. Effective flow shop scheduling can bring great benefits to the industry. However, there are few types of research on Distributed Hybrid Flow Shop Problems (DHFSP) by learning assisted meta-heuristics. This work addresses a DHFSP with minimizing the maximum completion time (Makespan). First, a mathematical model is developed for the concerned DHFSP. Second, four Q-learning-assisted meta-heuristics, e.g., genetic algorithm (GA), artificial bee colony algorithm (ABC), particle swarm optimization (PSO), and differential evolution (DE), are proposed. According to the nature of DHFSP, six local search operations are designed for finding high-quality solutions in local space. Instead of random selection, Q-learning assists meta-heuristics in choosing the appropriate local search operations during iterations. Finally, based on 60 cases, comprehensive numerical experiments are conducted to assess the effectiveness of the proposed algorithms. The experimental results and discussions prove that using Q-learning to select appropriate local search operations is more effective than the random strategy. To verify the competitiveness of the Q-learning assistedmeta-heuristics, they are compared with the improved iterated greedy algorithm (IIG), which is also for solving DHFSP. The Friedman test is executed on the results by five algorithms. It is concluded that the performance of four Q-learning-assisted meta-heuristics are better than IIG, and the Q-learning-assisted PSO shows the best competitiveness.Keywords
Cite This Article
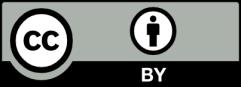
This work is licensed under a Creative Commons Attribution 4.0 International License , which permits unrestricted use, distribution, and reproduction in any medium, provided the original work is properly cited.