Open Access
ARTICLE
HWD-YOLO: A New Vision-Based Helmet Wearing Detection Method
1 College of Information Science and Technology, Beijing University of Technology, Beijing, 100124, China
2 Chinese Institute of Coal Science, Beijing, 100013, China
3 State Key Laboratory for Intelligent Coal Mining and Strata Control, Beijing, 100013, China
4 Engineering Research Center of Digital Community of Ministry of Education, Beijing, 100124, China
* Corresponding Author: Liang Wang. Email:
Computers, Materials & Continua 2024, 80(3), 4543-4560. https://doi.org/10.32604/cmc.2024.055115
Received 17 June 2024; Accepted 12 August 2024; Issue published 12 September 2024
Abstract
It is crucial to ensure workers wear safety helmets when working at a workplace with a high risk of safety accidents, such as construction sites and mine tunnels. Although existing methods can achieve helmet detection in images, their accuracy and speed still need improvements since complex, cluttered, and large-scale scenes of real workplaces cause server occlusion, illumination change, scale variation, and perspective distortion. So, a new safety helmet-wearing detection method based on deep learning is proposed. Firstly, a new multi-scale contextual aggregation module is proposed to aggregate multi-scale feature information globally and highlight the details of concerned objects in the backbone part of the deep neural network. Secondly, a new detection block combining the dilate convolution and attention mechanism is proposed and introduced into the prediction part. This block can effectively extract deep features while retaining information on fine-grained details, such as edges and small objects. Moreover, some newly emerged modules are incorporated into the proposed network to improve safety helmet-wearing detection performance further. Extensive experiments on open dataset validate the proposed method. It reaches better performance on helmet-wearing detection and even outperforms the state-of-the-art method. To be more specific, the mAP increases by 3.4%, and the speed increases from 17 to 33 fps in comparison with the baseline, You Only Look Once (YOLO) version 5X, and the mean average precision increases by 1.0% and the speed increases by 7 fps in comparison with the YOLO version 7. The generalization ability and portability experiment results show that the proposed improvements could serve as a springboard for deep neural network design to improve object detection performance in complex scenarios.Keywords
Cite This Article
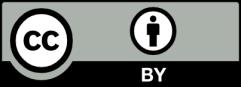
This work is licensed under a Creative Commons Attribution 4.0 International License , which permits unrestricted use, distribution, and reproduction in any medium, provided the original work is properly cited.