Open Access
ARTICLE
Diabetic Retinopathy Detection: A Hybrid Intelligent Approach
1 Department of Computer Science (CS), College of Computer Science and Information Technology (CCSIT), Imam Abdulrahman Bin Faisal University (IAU), P.O. Box 1982, Dammam, 31441, Saudi Arabia
2 Department of Computer Engineering (CE), College of Computer Science and Information Technology (CCSIT), Imam Abdulrahman Bin Faisal University (IAU), P.O. Box 1982, Dammam, 31441, Saudi Arabia
* Corresponding Author: Atta Rahman. Email:
(This article belongs to the Special Issue: Deep Learning in Medical Imaging-Disease Segmentation and Classification)
Computers, Materials & Continua 2024, 80(3), 4561-4576. https://doi.org/10.32604/cmc.2024.055106
Received 17 June 2024; Accepted 09 August 2024; Issue published 12 September 2024
Abstract
Diabetes is a serious health condition that can cause several issues in human body organs such as the heart and kidney as well as a serious eye disease called diabetic retinopathy (DR). Early detection and treatment are crucial to prevent complete blindness or partial vision loss. Traditional detection methods, which involve ophthalmologists examining retinal fundus images, are subjective, expensive, and time-consuming. Therefore, this study employs artificial intelligence (AI) technology to perform faster and more accurate binary classifications and determine the presence of DR. In this regard, we employed three promising machine learning models namely, support vector machine (SVM), k-nearest neighbors (KNN), and Histogram Gradient Boosting (HGB), after carefully selecting features using transfer learning on the fundus images of the Asia Pacific Tele-Ophthalmology Society (APTOS) (a standard dataset), which includes 3662 images and originally categorized DR into five levels, now simplified to a binary format: No DR and DR (Classes 1–4). The results demonstrate that the SVM model outperformed the other approaches in the literature with the same dataset, achieving an excellent accuracy of 96.9%, compared to 95.6% for both the KNN and HGB models. This approach is evaluated by medical health professionals and offers a valuable pathway for the early detection of DR and can be successfully employed as a clinical decision support system.Keywords
Cite This Article
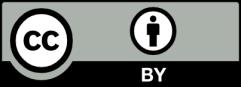
This work is licensed under a Creative Commons Attribution 4.0 International License , which permits unrestricted use, distribution, and reproduction in any medium, provided the original work is properly cited.