Open Access
ARTICLE
Phenotypic Image Recognition of Asparagus Stem Blight Based on Improved YOLOv8
College of Information Science and Engineering, Shandong Agricultural University, Tai’an, 271000, China
* Corresponding Author: Chao Zhang. Email:
Computers, Materials & Continua 2024, 80(3), 4017-4029. https://doi.org/10.32604/cmc.2024.055038
Received 14 June 2024; Accepted 19 July 2024; Issue published 12 September 2024
Abstract
Asparagus stem blight, also known as “asparagus cancer”, is a serious plant disease with a regional distribution. The widespread occurrence of the disease has had a negative impact on the yield and quality of asparagus and has become one of the main problems threatening asparagus production. To improve the ability to accurately identify and localize phenotypic lesions of stem blight in asparagus and to enhance the accuracy of the test, a YOLOv8-CBAM detection algorithm for asparagus stem blight based on YOLOv8 was proposed. The algorithm aims to achieve rapid detection of phenotypic images of asparagus stem blight and to provide effective assistance in the control of asparagus stem blight. To enhance the model’s capacity to capture subtle lesion features, the Convolutional Block Attention Module (CBAM) is added after C2f in the head. Simultaneously, the original CIoU loss function in YOLOv8 was replaced with the Focal-EIoU loss function, ensuring that the updated loss function emphasizes higher-quality bounding boxes. The YOLOv8-CBAM algorithm can effectively detect asparagus stem blight phenotypic images with a mean average precision (mAP) of 95.51%, which is 0.22%, 14.99%, 1.77%, and 5.71% higher than the YOLOv5, YOLOv7, YOLOv8, and Mask R-CNN models, respectively. This greatly enhances the efficiency of asparagus growers in identifying asparagus stem blight, aids in improving the prevention and control of asparagus stem blight, and is crucial for the application of computer vision in agriculture.Keywords
Cite This Article
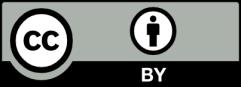
This work is licensed under a Creative Commons Attribution 4.0 International License , which permits unrestricted use, distribution, and reproduction in any medium, provided the original work is properly cited.