Open Access
ARTICLE
Machine Fault Diagnosis Using Audio Sensors Data and Explainable AI Techniques-LIME and SHAP
1 School of Data and Sciences, Brac University, Dhaka, 1212, Bangladesh
2 JW KIM College of Future Studies, Endicott College, Woosong University, Daejeon, 300-718, Republic of Korea
3 AI and Big Data Department, Endicott College, Woosong University, Daejeon, 300-718, Republic of Korea
* Corresponding Author: Jia Uddin. Email:
Computers, Materials & Continua 2024, 80(3), 3463-3484. https://doi.org/10.32604/cmc.2024.054886
Received 10 June 2024; Accepted 14 August 2024; Issue published 12 September 2024
Abstract
Machine fault diagnostics are essential for industrial operations, and advancements in machine learning have significantly advanced these systems by providing accurate predictions and expedited solutions. Machine learning models, especially those utilizing complex algorithms like deep learning, have demonstrated major potential in extracting important information from large operational datasets. Despite their efficiency, machine learning models face challenges, making Explainable AI (XAI) crucial for improving their understandability and fine-tuning. The importance of feature contribution and selection using XAI in the diagnosis of machine faults is examined in this study. The technique is applied to evaluate different machine-learning algorithms. Extreme Gradient Boosting, Support Vector Machine, Gaussian Naive Bayes, and Random Forest classifiers are used alongside Logistic Regression (LR) as a baseline model because their efficacy and simplicity are evaluated thoroughly with empirical analysis. The XAI is used as a targeted feature selection technique to select among 29 features of the time and frequency domain. The XAI approach is lightweight, trained with only targeted features, and achieved similar results as the traditional approach. The accuracy without XAI on baseline LR is 79.57%, whereas the approach with XAI on LR is 80.28%.Keywords
Cite This Article
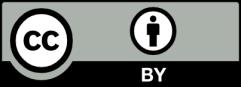
This work is licensed under a Creative Commons Attribution 4.0 International License , which permits unrestricted use, distribution, and reproduction in any medium, provided the original work is properly cited.