Open Access
ARTICLE
A Low Complexity ML-Based Methods for Malware Classification
1 Cybersecurity Department, Al-Zaytoonah University of Jordan, Amman, 11733, Jordan
2 Department of Computer Science and Information Technology, Applied College, Princess Nourah Bint Abdulrahman University, P.O. Box 84428, Riyadh, 11671, Saudi Arabia
3 Faculty of Information Technology, Applied Science Private University, Amman, 11931, Jordan
4 Computer Systems Program-Electrical Engineering Department, Faculty of Engineering-Shoubra, Benha University, Cairo, 11629, Egypt
5 Research Center for Artificial Intelligence and Cybersecurity, Electronics and Informatics Organization, National Research and Innovation Agency (BRIN), KST Samaun Samadikun, Bandung, 40135, Republic of Indonesia
6 Jadara Research Center, Jadara University, Irbid, 21110, Jordan
7 MEU Research Unit, Middle East University, Amman, 11831, Jordan
* Corresponding Authors: Mahmoud E. Farfoura. Email: ; Deema Mohammed Alsekait. Email:
(This article belongs to the Special Issue: Applications of Artificial Intelligence for Information Security)
Computers, Materials & Continua 2024, 80(3), 4833-4857. https://doi.org/10.32604/cmc.2024.054849
Received 09 June 2024; Accepted 19 August 2024; Issue published 12 September 2024
Abstract
The article describes a new method for malware classification, based on a Machine Learning (ML) model architecture specifically designed for malware detection, enabling real-time and accurate malware identification. Using an innovative feature dimensionality reduction technique called the Interpolation-based Feature Dimensionality Reduction Technique (IFDRT), the authors have significantly reduced the feature space while retaining critical information necessary for malware classification. This technique optimizes the model’s performance and reduces computational requirements. The proposed method is demonstrated by applying it to the BODMAS malware dataset, which contains 57,293 malware samples and 77,142 benign samples, each with a 2381-feature vector. Through the IFDRT method, the dataset is transformed, reducing the number of features while maintaining essential data for accurate classification. The evaluation results show outstanding performance, with an F1 score of 0.984 and a high accuracy of 98.5% using only two reduced features. This demonstrates the method’s ability to classify malware samples accurately while minimizing processing time. The method allows for improving computational efficiency by reducing the feature space, which decreases the memory and time requirements for training and prediction. The new method’s effectiveness is confirmed by the calculations, which indicate significant improvements in malware classification accuracy and efficiency. The research results enhance existing malware detection techniques and can be applied in various cybersecurity applications, including real-time malware detection on resource-constrained devices. Novelty and scientific contribution lie in the development of the IFDRT method, which provides a robust and efficient solution for feature reduction in ML-based malware classification, paving the way for more effective and scalable cybersecurity measures.Keywords
Cite This Article
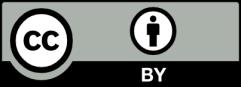
This work is licensed under a Creative Commons Attribution 4.0 International License , which permits unrestricted use, distribution, and reproduction in any medium, provided the original work is properly cited.