Open Access
ARTICLE
Knowledge-Driven Possibilistic Clustering with Automatic Cluster Elimination
1 The School of Computer Science and Engineering, Southeast University, Nanjing, 211189, China
2 The School of Computer and Information, Hefei University of Technology, Hefei, 230601, China
3 The Department of Electrical and Computer Engineering, University of Alberta, Edmonton, AB T6R 2V4, Canada
4 The Systems Research Institute, Polish Academy of Sciences, Warsaw, 00-901, Poland
5 The School of Information Engineering, Nanjing University of Finance and Economics, Nanjing, 210023, China
* Corresponding Authors: Jiuchuan Jiang. Email: ; Yichuan Jiang. Email:
Computers, Materials & Continua 2024, 80(3), 4917-4945. https://doi.org/10.32604/cmc.2024.054775
Received 06 June 2024; Accepted 14 August 2024; Issue published 12 September 2024
Abstract
Traditional Fuzzy C-Means (FCM) and Possibilistic C-Means (PCM) clustering algorithms are data-driven, and their objective function minimization process is based on the available numeric data. Recently, knowledge hints have been introduced to form knowledge-driven clustering algorithms, which reveal a data structure that considers not only the relationships between data but also the compatibility with knowledge hints. However, these algorithms cannot produce the optimal number of clusters by the clustering algorithm itself; they require the assistance of evaluation indices. Moreover, knowledge hints are usually used as part of the data structure (directly replacing some clustering centers), which severely limits the flexibility of the algorithm and can lead to knowledge misguidance. To solve this problem, this study designs a new knowledge-driven clustering algorithm called the PCM clustering with High-density Points (HP-PCM), in which domain knowledge is represented in the form of so-called high-density points. First, a new data density calculation function is proposed. The Density Knowledge Points Extraction (DKPE) method is established to filter out high-density points from the dataset to form knowledge hints. Then, these hints are incorporated into the PCM objective function so that the clustering algorithm is guided by high-density points to discover the natural data structure. Finally, the initial number of clusters is set to be greater than the true one based on the number of knowledge hints. Then, the HP-PCM algorithm automatically determines the final number of clusters during the clustering process by considering the cluster elimination mechanism. Through experimental studies, including some comparative analyses, the results highlight the effectiveness of the proposed algorithm, such as the increased success rate in clustering, the ability to determine the optimal cluster number, and the faster convergence speed.Keywords
Cite This Article
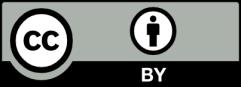
This work is licensed under a Creative Commons Attribution 4.0 International License , which permits unrestricted use, distribution, and reproduction in any medium, provided the original work is properly cited.