Open Access
REVIEW
Survey on Video Security: Examining Threats, Challenges, and Future Trends
1 Department of Artificial Intelligence, Faculty of Computing, The Islamia University of Bahawalpur, Bahawalpur, 63100, Pakistan
2 School of Computer Science, College of Science and Engineering, University of Galway, Galway, H91 TK33, Ireland
* Corresponding Author: Mamoona Naveed Asghar. Email:
# Ali Asghar and Amna Shifa contributed equally to this work
Computers, Materials & Continua 2024, 80(3), 3591-3635. https://doi.org/10.32604/cmc.2024.054654
Received 04 June 2024; Accepted 20 August 2024; Issue published 12 September 2024
Abstract
Videos represent the most prevailing form of digital media for communication, information dissemination, and monitoring. However, their widespread use has increased the risks of unauthorised access and manipulation, posing significant challenges. In response, various protection approaches have been developed to secure, authenticate, and ensure the integrity of digital videos. This study provides a comprehensive survey of the challenges associated with maintaining the confidentiality, integrity, and availability of video content, and examining how it can be manipulated. It then investigates current developments in the field of video security by exploring two critical research questions. First, it examine the techniques used by adversaries to compromise video data and evaluate their impact. Understanding these attack methodologies is crucial for developing effective defense mechanisms. Second, it explores the various security approaches that can be employed to protect video data, enhancing its transparency, integrity, and trustworthiness. It compares the effectiveness of these approaches across different use cases, including surveillance, video on demand (VoD), and medical videos related to disease diagnostics. Finally, it identifies potential research opportunities to enhance video data protection in response to the evolving threat landscape. Through this investigation, this study aims to contribute to the ongoing efforts in securing video data, providing insights that are vital for researchers, practitioners, and policymakers dedicated to enhancing the safety and reliability of video content in our digital world.Keywords
To provide end users with an immersive and interactive experience, multimedia applications are frequently used to create, deliver, store, and manipulate digital media content [1]. However, in today’s fast-paced technological era, videos have emerged as the most informative medium among all types of multimedia, serving various users, including business and home users. They serve various practical purposes, including education, communication, entertainment, analytics, dynamic scientific visualization/analytics, surveillance, advertising, and video conferencing [2]. Platforms like Google and YouTube offer solutions to various queries and problems, with video tutorials becoming preferred over lengthy textual explanations due to time constraints. Additionally, watching videos allows for multitasking, enabling users to engage in other activities while enjoying content. A report reveals that 66% of consumers prefer to see video content [3]. According to Cisco, video traffic accounted for 82% of all internet traffic in 2021 and is projected to reach 91% by 2026 [4]. This increasing reliance on video content underscores the urgent need for secure video transmission, as these are multicast to subscribers, and personal videos are exchanged among internet users. The risks of unauthorised access and manipulation of video data through complex and frequent attacks are significant, and the inherent openness of network channels makes them vulnerable to attacks and communication errors [5]. This poses a pressing need for robust security measures when disseminating confidential or sensitive information, including military, financial, or private videos. Furthermore, increasing trends in cloud computing have made the protection of distributed content even more important. In cloud computing, all the multimedia contents are stored on a central storage device known as a cloud. All the contents shared by the users on social media applications (such as Facebook, Twitter, and Instagram) are stored on the central server of those applications. In such cases, there is a possibility that an unauthorised person who is connected to the same network may view or access someone else’s confidential data. Hence, it is necessary to provide proper security measures to the user before distributing the contents of that particular user to provide them with basic information security services.
Moreover, the rapid increase in video camera monitoring, recording, and archiving of videos of individuals in public or private settings has heightened ethical and privacy risks [6]. Recently, during the Russia-Ukraine war, Russian hackers hacked two surveillance cameras in Kyiv ahead of missile attacks [7]. To prevent further misuse, the Ukraine agency blocked 10,000 surveillance cameras. Furthermore, the government advised the people against using live video or images, as such footage can provide intelligence on the country’s defenses or military movements. However, it is not feasible for any government to impose large-scale restrictions on people. Illegal users can employ sophisticated attacks and video manipulation techniques such as object addition, removal, or insertion to alter critical video footage or defame individuals, as seen in deepfake videos [8]. Additionally, increasing trends in cloud computing have made protecting video data even more important, as the central server provides all processing, computations, and storage. In such cases, an unauthorised person connected to the same network may view or access someone else’s confidential data.
To address security and privacy concerns, video content producers ensure their work remains unaltered, fostering a reliable and trustworthy online environment. A major hosting service provider, YouTube, has taken proactive steps by introducing a pixelation tool in its YouTube Creator Studio [9]. On the other hand, encryption is pivotal in safeguarding digital videos, especially in an era where video dissemination is crucial for information sharing. Encryption can be performed on either full video content, known as Naïve Encryption (NE), or half of the contents of the video, also known as Selective Encryption (SE). However, the evolving landscape of video technology has outpaced conventional encryption methods [10], with high-definition (HD) videos now incorporating advanced features such as enhanced color depth, color correction, filtering, re-encoding, and redundancies. Moreover, attacks are increasing in complexity and frequency, making current countermeasures insufficient to prevent them. Fortunately, technological advancements also offer promising avenues, with increasingly advanced approaches being developed, such as deoxyribonucleic acid (DNA) based security, blockchain technology, watermarking, digital rights management (DRM), machine learning (ML), and more. These solutions help mitigate risks such as manipulation, illegal redistribution, and intellectual property theft, providing a robust defense against threats for organisations, individuals, and content providers. Many such techniques will be explored in subsequent sections. Considering the current developments, this paper aims to investigate the following two research questions from different perspectives:
RQ1: What techniques do adversaries use to compromise, falsify, misuse, and damage the integrity, confidentiality, or authenticity of video data? (Attacks).
RQ2: What technologies can be used to safeguard video data and improve its transparency, integrity, and trustworthiness? (Countermeasures).
Although a significant number of security techniques are currently under development to counter complex video manipulation methods and security attacks, despite these efforts, a notable gap persists in understanding the evolving landscape of security techniques for video data. This paper aims to bridge that gap by offering an extensive review of video data security, focusing on attack analysis and eleven distinct security approaches while considering recent technological advancements. While existing reviews tend to be outdated [11,12], fail to incorporate recent developments, or focus primarily on specific use cases [13–16]. This article is crafted to be understandable by any reader, irrespective of their technical expertise. It aims to consolidate existing knowledge, provide insights, and guide the development of new solutions in the field of video data security. The findings of this survey will provide reference to researchers seeking in-depth insights into security strategies, as well as key challenges and future trends unique to video data security in their ongoing research.
The primary contributions of this paper are outlined as follows:
1. Analysis of various manipulation techniques that can be used to alter video footage. Additionally, we examine attacks targeting security services such as integrity, authenticity, and confidentiality of video content, as well as disruptions to video delivery services.
2. Research indicates a notable gap in the state-of-the-art security techniques adopted against attacks on all types of video data over the past decade. This paper addresses this gap by presenting a detailed analysis of current trends in safeguarding video data.
3. The paper highlights the open research challenges that need to be addressed to enhance video security. Additionally, the paper emphasises the importance of developing efficient, scalable, and transparent security technologies to keep pace with the evolving landscape of video manipulation and attacks.
The survey structure, as illustrated in Fig. 1, comprises the following sections: Section 2 discusses the background of the study and the associated challenges in securing video data. Section 3 explores how videos can be manipulated, followed by an explanation of several attacks on videos and their impacts in Section 4. Section 5, divided into twelve subsections, examines different security techniques for safeguarding videos. The pros and cons are discussed in Section 6, followed by future trends in Section 7. Finally, Section 8 concludes the paper.
Figure 1: Structure of the paper (all sections represent the discussion on videos)
Videos are generally classified into two types: continuous and non-continuous videos, as shown in Fig. 2. In Continuous video, frames are captured continuously without interruption to record an event, commonly utilised in surveillance systems where real-time monitoring or viewing is necessary. On the other hand, non-continuous video involves periodic examination of video feeds, which are reviewed during playback rather than in real-time (e.g., Video on Demand (VoD)). Regardless of the type of video, these videos are composed of sequences of highly correlated images known as frames [17]. Each time these frames are delivered to an end user, they undergo several processing stages, such as encoding, compression, digitisation, quantisation, decompression, and decoding, and they pass through various platforms and communication channels and are then stored. As a result, they are more susceptible to manipulation and cyberattacks. However, effectively safeguarding video data against these threats and ensuring confidentiality, integrity, availability, and accountability during all phases of video data remains challenging. Some of the key challenges include:
1. Large Volume and Complexity: Video data is typically larger and more complex than other data forms. This complexity arises from factors such as high-resolution imagery, audio components, and multiple streams. Securing such large volumes of data presents technological challenges due to significant bandwidth, storage, and processing requirements. Bandwidth limitations can lead to latency issues and reduced video quality while applying encryption to large volumes of video data can be costly and resource-intensive [18].
2. Computational Overhead: Ensuring integrity, authenticity, and confidentiality in real-time video streams demands significant computational resources and sophisticated algorithms. However, measures such as encryption like AES (Advanced Encryption Standard) and digital signatures or watermarking can introduce a substantial computational overhead, which in turn can impact the performance and user experience. It is important to note that the financial cost of implementing and maintaining robust security measures can be significant, adding another layer of complexity to the video data security landscape.
3. Privacy Concerns: Videos often contain sensitive information, such as face recognition and behavior information, that may be required for analytics and business insights. Balancing security with the ability to analyse or search video content for legitimate purposes is an open research question.
4. Interoperability Issues: Video data often involves different formats, codecs, and standards that may not seamlessly interact with each other across various hardware and software platforms. Ensuring interoperability between different systems and devices while protecting the video is hard.
5. Vulnerability to Cyberattacks: Video data faces security risks throughout its lifecycle, including transmission, storage, and processing. It is susceptible to various cyber threats, such as unauthorised access, data breaches, and manipulation. Malicious actors may exploit vulnerabilities in video management software, cameras, or transmission protocols to compromise the security and integrity of video content [19]. Securing data at each stage demands different measures.
6. Advanced Video Manipulation Techniques: As video technologies evolve, so do the methods employed by attackers. The emergence of advanced threats using Artificial Intelligence (AI), such as deepfake, AI-morphed videos, spoofing, manipulating video metadata, or using sophisticated AI-generated manipulation tools to alter the context or content of the video, poses risks to the integrity, authenticity, and possibility of misuse of the information. Even people without prior knowledge of technology can now easily manipulate or fabricate videos using applications such as DeepFaceLab and FaceSwap. However, developing reliable methods to detect deepfakes and other AI-generated manipulations is a constant challenge and requires continuous adaptation and enhanced security measures.
7. Sophisticated Attacks Surface: With the rise of AI and ML, attacks are becoming more sophisticated. Failing to secure the data can damage trust in video data organisations. Therefore, security measures must constantly adapt to new threats to protect video data from cyberattacks effectively.
Figure 2: Classification of videos
This section presents an overview of some manipulation techniques that are commonly used for video data. The various manipulation techniques are discussed in detail below:
1. Inter-Frame Tampering: This fraudulent approach adds, deletes, or alters frames, distorting, falsifying, or exhibiting other irregularities. Frames are altered or manipulated to disrupt a video sequence’s chronological order and consistency [20]. Inter-frame manipulation can be used to construct narratives, conceal important information, or make events appear more plausible than they are.
Frame Insertion: Intentionally adding frames to a video sequence to introduce new visual information or alter the temporal flow is known as frame insertion [21]. Frame insertion can be used to manipulate the timing of crucial moments in a video or fabricate evidence, leading to erroneous inferences or interpretations.
2. Intra-Frame Tampering: Videos that have been altered or manipulated on a frame-by-frame basis without disrupting the overall chronological order of the sequence are referred to as intra-frame tampering [22]. Using this method, targeted changes can be made within a single frame. Intra-frame tampering can be used to fabricate false information within a single frame or perform retouching to diminish visual quality.
i) Object Addition: It involves intentionally including new components or objects that were not present during the recording [23]. This type of modification is often used for deceptive or distracting purposes.
ii) Object Removal: Object removal involves deliberately deleting specific objects or features from the frame that were present during recording [24]. This type of alteration is often used to reduce visual clarity, introduce distractions, or achieve nefarious goals. It may also be employed to mislead viewers or conceal important details within the video sequence.
3. Resampling: The process of enhancing or altering visual elements to rectify flaws or alter the narrative is known as resampling [25]. It involves adjusting the video’s aspect ratio, frame rate, or resolution to meet specific display requirements or artistic objectives. Attackers can use these techniques to mislead the viewer about the video’s content.
4. Style-and-Motion Transfer: Style-and-motion transfer is the sophisticated technique of combining motion transfer, which adds a video’s motion characteristics to another, with style transfer [22]. Dishonest actors can manipulate video appearance and motion to fabricate events, distort reality, or deceive viewers. For instance, criminals may use style transfer to mimic the visuals of reputable news sources to disseminate fake information or false legitimacy. Additionally, motion transfer can overlay individuals’ actions onto unrelated situations, falsely implicating them in activities in which they were not involved.
5. Deepfake: Deepfake or AI-forged video technology involves altering original video content to create highly realistic but fake frames using AI and neural networks, particularly generative adversarial networks (GANs) tools [26]. Hence, it violates the video’s integrity by introducing elements that are not part of the original footage. Threat actors utilise deepfake for deception, fraud, and social engineering. Additionally, fabricating realistic but false video content can deceive viewers into believing false events or statements, undermining trust in video data and causing viewers to question the authenticity of even legitimate videos.
6. Spoofing: Spoofing refers to deceiving or tricking a system into accepting false data as genuine. Spoofing in the video can be utilised in various ways to compromise the authenticity, integrity, and confidentiality of video data, including altering identities, content, sources, timestamps, frames, and network transmissions [27]. Attackers can create compelling yet false video content. For example, network spoofing can intercept and alter video data as it is being streamed, making real-time changes that go undetected by the recipient. This can include adding fake events, altering backgrounds, or inserting false information. Additionally, attackers can spoof the source of a video feed, making it appear that the video is coming from a trusted source when it is not. This can involve hijacking live feeds or tampering with metadata to disguise the origin of the video.
Fig. 3 illustrates some of the data manipulation and privacy protection techniques.
Figure 3: Video data manipulation and protection methods
4 Attacks on Video Data Security
This section explores various malicious activities aimed at compromising the integrity, authenticity, or confidentiality of videos. Fig. 4 illustrates the major security threats targeting video data and its processing.
Figure 4: Attacks that can compromise the videos
4.1 Attacks on Confidentiality of Videos
Confidentiality is a critical security principle, particularly in the context of video, as it primarily ensures the secrecy of data. Below are the attacks that can compromise the confidentiality of video data.
Interception Attack: In an interception attack, an unauthorised individual attempts to gain access to valuable information transmitted between two parties [28]. The hacker may intercept sensitive data by eavesdropping on the transmission channel, using specialised software to capture data, physically tapping into network cables, or analysing network traffic. In [29], the authors proposed the PriSE method, which restricts access only to authorized users, thereby ensuring video protection. Furthermore, vulnerability assessments aid in detecting and preventing interception attacks.
Eavesdropping Attack: In such an attack, hackers tap into the network cables to eavesdrop on confidential data transmitted over private communication channels without the knowledge or consent of the parties involved [30]. To address this, the authors [31] proposed a hybrid encryption technique for securing video conferencing. Combining classical networks and quantum keys helps protect the transmitted data, making it difficult for hackers to decrypt the information even if intercepted. The encrypted scheme can also efficiently resist high-performance computational threats such as eavesdropping.
Hijacking Attack: The hijacking attack occurs when a hacker tricks a user into clicking on a hidden or disguised link or button, often leading to unintended actions such as downloading malware or making fraudulent purchases [32]. There are several types of hijacking attacks, such as session hijacking, where the attacker steals the user’s session ID (session identifier) or cookies, and DNS (Domain Name System) hijacking, where the DNS settings are modified to redirect traffic to a malicious website. In [33], a radio frequency identification technique is presented to prevent unauthorised access.
Man-in-the-Middle Attack: In a man-in-the-middle (MITM) attack, the hacker positions themselves between two parties’ transmissions and can easily read messages that are being exchanged [34]. The middleman can inject or modify new messages into the conversation to impersonate one of the parties. To reduce the MITM attack, an enhanced version of the Discrete-Logarithm Problem (DLP) was proposed to secure the communication channel [35]. The attacker cannot manipulate or impersonate the exchanged information, nor can they obtain the encryption key. Fig. 5 illustrates the implementation of an MITM attack.
Figure 5: Man-in-the-middle attack
Interference Attack: In an interference attack [36], the hacker disrupts the normal functioning of communication by injecting noise or other unwanted signals. A radio frequency jammer can be used to transmit signals at the same frequency as the communication channel, disrupting the transmission. These attacks pose a significant problem in wireless communication, where communication signals are vulnerable to interference from other sources.
4.2 Attacks on Integrity of Videos
Integrity ensures that video data remains accurate, consistent, authentic, and reliable in video processing. Below are the attacks that can compromise the trustworthiness and reliability of video content.
Replays Attack: In a replay attack [37], the attacker captures a valid data transmission, such as a login request or a video transaction, then resends it to the server later. If the server accepts the replayed data transmission, the attacker can conduct malicious actions such as accessing sensitive information or transferring illegal video data. To prevent replay attacks, a modified high-frequency descriptor technique was used for video streams [38], thus ensuring the integrity of the data being transmitted.
Packet Injection Attack: Packet injection attacks can be executed through various methods, including the use of network sniffers, physical wiretaps, or malware [39]. In this attack, hackers send packets that mimic legitimate ones to gain illegal access to a network or compromise a device’s security. The hacker can modify or delete frames from a video sequence. In [40], the authors discussed encryption schemes that can protect data from interception, make it challenging for attackers to modify information, and restrict access only to authorised users.
Fabrication Attack: The fabrication attack involves injecting false data, such as fake video credentials, to manipulate the originality of a video sequence. The attack can be carried out in various ways, such as using social engineering tactics to trick users into providing sensitive information or exploiting vulnerabilities in software or hardware. To prevent fabrication attacks, the paper utilised YOLO (You Only Look Once) and SSD (Single Shot multibox Detector) algorithms [41]. The proposed method was effective against fabrication attacks and ensured the integrity of the video data.
Message Deletion Attack: A message deletion attack may involve the manipulation or deletion of video frames or metadata within a video stream [42]. A hacker might delete metadata within a video file, such as time codes or camera information, to conceal evidence of malicious activity or hinder the viewer’s ability to interpret the video accurately. Therefore, an efficient detection scheme was introduced by [43] that can prevent message deletion attacks.
Payload Attack: These attacks target the integrity of the video system, as discussed in [44]. Hackers can embed unwanted scripts or executable code within video files. When users open or play the injected video, the malware payload is executed, resulting in the installation of malicious software, data breaches, and system compromise. Hackers may leverage vulnerabilities to deliver payloads that exploit weaknesses and gain unauthorised access, compromising user privacy. The following measures can be implemented to mitigate payload attacks: patch management, secure coding practices, anti-malware protection, user awareness and education, sandboxing and virtualisation, and content verification.
Masquerade Attack: The hacker embedded the masquerade attack to impersonate or deceive users as trusted video sources by spoofing their identities [45]. They may forge video stream metadata like addresses, timestamps, source IP (Internet Protocol), and digital signatures to trick the video processing system into processing and accepting the video from an unauthorised source. This attack can be secured through secure authentication, access control, digital signatures, encryption and secure protocols, user training, content integrity verification, system monitoring, and anomaly detection.
4.3 Attacks on Availability of Videos
Availability ensures that videos are available for authorised users and can compromise in the following ways:
Denial-of-Service Attack: A denial-of-service (DoS) attack targeted at a video sequence can result in significant disruption to video streaming [46]. There are several ways in which a DoS attack can impact video processing: (1) Network congestion: flooding a large number of requests to the network can cause congestion, making the video unwatchable; (2) Resource depletion: consuming resources like CPU (Central Processing Unit) and memory can cause the system to crash, rendering the video unresponsive; (3) Packet fragmentation attack: sending a huge number of fragmented packets to the video system can cause the system to become unstable.
Jamming Attack: A jamming attack refers to the intentional disruption of wireless video transmission by transmitting radio signals at the same frequency as the video signal [47]. Through this attack, video streams may be disrupted, resulting in pixelation, delays, prevention of monitoring critical events or areas, complete loss of signal, and loss of frames in live video streaming. Ashourian et al. proposed the Karhunen–Loeve transform (KLT) technique, based on the spiked matrix model and wigner matrix, which can help minimise the impact of jamming attacks, as discussed in [48].
Routing and Command and Control (C&C) Attack: In a routing and C&C attack, an attacker can manipulate the destination route, reroute video traffic to a malicious destination, and gain control of the video system [49]. This allows them to execute commands to access, manipulate, or steal video data, potentially obtaining sensitive information or using it for blackmail.
4.4 Attacks on Authentication of Videos
It refers to the illegal attempt to gain access to a service or systems by exploiting vulnerabilities using the following methods.
GCS (Ground Control Station) Spoofing Attack: In a GCS spoofing attack, a hacker impersonates a Ground Control Station in a video-based unmanned aerial vehicle (UAV) system. The GCS controls the UAV and receives video data from it. In such an attack, the hacker sends false signals to the UAV, causing it to follow commands from the hacker instead of the legitimate GCS. Thus, the hacker can take control of the UAV and gain access to the video data being transmitted from the UAV’s camera. Wei et al. presented a semantic-based method that can detect the spoofing attack from the UAV [50].
4.5 Machine Learning Attacks on Videos
Machine learning attacks refer to utilising vulnerabilities in the system using various ML algorithms and techniques, as discussed below.
Spam Filtering Attack: Evasion attacks, or spam filtering attacks, are not typically delivered directly to the user’s inbox [51]. Hackers upload videos containing offensive or harmful content. They spam a large volume of data to make it difficult for users to find legitimate content, as discussed in [52]. These videos may be created using editing techniques or deepfake technology to appear trustworthy. Therefore, these attacks on video platforms and streaming services can be handled through collaborative filtering, content moderation, video analysis and classification, user reporting and feedback, and enhanced video metadata.
Intrusion Detection Attack: An intrusion attack involves uploading and streaming videos that contain malicious material or unauthorised content [53]. In such an attack, the hacker can modify or tamper with the video content by altering the video frames and metadata, deceiving the video processing system, and manipulating the recorded videos. The attack can be detected by system monitoring, video authentication, real-time video stream analysis, access control and encryption, and metadata analysis.
Fraud Detection Attack: This attack can be employed for fraudulent purposes by creating realistic videos that mislead users or manipulate information [20]. Hackers may create or distribute videos that promote scams, illegal activities, or counterfeit products. To combat these attacks, it is necessary to update security measures regularly and stay informed about emerging fraud techniques. Measures such as real-time monitoring, video authenticity verification, facial recognition and biometrics, user feedback and reporting, and behavior analysis can help in this regard.
Network Traffic Detection Attack: In this particular attack, hackers can intercept video traffic between the source and destination or modify the video content [54]. They can use packet sniffing tools to analyse traffic patterns associated with video systems to gain insights into system vulnerabilities or sensitive information. Therefore, these attacks on video platforms and streaming services can be handled through encryption and secure protocols, traffic monitoring and analysis, access control and authentication, intrusion detection/prevention (ID/IP), and network segmentation.
Table 1 highlights some of the attacks, their impact, and detection techniques while details are provided above.
This section provides a detailed overview of contemporary approaches to protecting video data. These techniques encompass diverse measures, from well-established methods to cutting-edge advancements. Some of these video security techniques are implemented on publicly available datasets such as PETS2009 [55], Urban Tracker [56], MOT17 [57], Derf’s Test Media Collection [58], NBI-InfFrames [59], YUV Video Sequence [60], 3DV+D [61], UHD test sequences [62], USC-SIPI [63], and ABODA [64]. These datasets contain multiple features that can help researchers evaluate their security algorithms. Some scholars have also implemented security techniques on self-created datasets, such as in [38], where a spoofed replay attack is detected using a self-created video dataset, and in [65], different camera devices are used to obtain a three-dimensional video (3DV) output. Whereas synthetic datasets were taken from the BeamNG drive [66] in [67], VGGface2 [68], and Deepfake Detection Challenge (DFDC) Dataset [69], as utilised by [70].
5.1 Privacy-Protection Strategies
To safeguard video data, researchers have developed various methods that have been utilised for decades, and yet they continue to evolve to achieve data protection and privacy preservation. These techniques include blurring, pixelisation, morphing, encryption, and others, as described in Table 2, primarily focused on offline videos. However, with significant growth in live streaming, communities have discussions about implementing similar for live video. The paper [71], introduced a privacy protection method named Face Pixelation in Video Live Streaming (FPVLS) to safeguard personal privacy rights and foster the healthy growth of the video live streaming industry. The related work also demonstrates that some of these approaches do not pay attention to intelligibility and reversibility, memory-constrained hardware architectures, and low network bandwidths, not to mention privacy aspects. The weaknesses of some methods other than encryption, such as pixelation and blurring, are highlighted in [72].
5.2 Conventional Encryption Techniques
Nowadays, when media manipulation and deepfake are prevalent, encryption is vital for safeguarding video content’s integrity and authenticity. Cryptographic algorithms such as Data Encryption Standard (DES), Advanced Encryption Standard (AES), Rivest-Shamir-Adleman (RSA), etc., have been used to provide security since 1970. To mitigate the current challenges, an improved version of AES was introduced by [80], featuring a mix-row operation replacing sub-byte and shift-row operations to reduce runtime. While this scheme fully encrypts input video, its encryption time complexity is notably higher. A technique for securing video content and verifying receiver identity during online video streaming was proposed using a hybrid cryptography approach integrating AES, RSA, and Elliptic-curve cryptography (ECC) algorithms [81]. This hybrid model, combined with dynamically generated keys, significantly enhances security.
Blowfish, known for its efficiency in symmetric cipher algorithms, was employed by utilising key lengths ranging from 32 to 448 bits [82]. However, its susceptibility to weak key problems introduced complexity. To address this, the Blowfish algorithm was modified by altering the F-function structure, reducing the number of S-boxes to two, which enhanced efficiency and lowered computational costs [83]. In another study [84], the authors utilised the Blowfish algorithm to encrypt sensitive information, concealing it within steganographic images using the Least Significant Bit (LSB) technique. In [85], employed AES, the Vernam encryption algorithm, and LSB schemes to convert information into encrypted, incomprehensible forms. To solve the problem of key distribution in shared secret keys, a secure system based on cryptography and authentication was introduced by utilising elliptic-curve Diffie-Hellman (ECDH) [86]. The authors explored three systems aimed at securing the integration of encryption and authentication to ensure the authenticity and confidentiality of information exchanged between IoT (Internet of Things) devices over untrusted communication channels [86].
Chaotic techniques leverage mathematical functions to generate randomness or scramble data, providing promising solutions for video security. However, their effectiveness is constrained by limitations arising from their inherently less dynamic behavior. Nevertheless, they have been employed in video security with varying success. An intertwining logistic map scheme based on the cosine transformation of video was introduced by [87]. Permutation is applied to each frame to reduce pixel correlation; subsequently, the frames are rotated 90 degrees counterclockwise. Finally, a random substitution scheme is applied to make changes column-wise and row-wise, with frames bounced according to a frame selection key. In contrast, the authors [88] utilised improved chaotic maps and parallel compressive sensing techniques to efficiently encrypt/decrypt videos without compromising quality. A logistic Tent Infinite Collapse Map (LT-ICM) provides unpredictability, sensitivity, complex behavior, and a broader chaotic range for controlling parameters. Additionally, they proposed a substitution method consisting of exclusive-OR (XOR), modular addition operations, and circular shifts to substitute frames using a two-dimensional LT-ICM sequence. Recently, Hadjadj et al. [89] introduced a chaotic-based approach that relies on a unified Lorenz chaotic generator and the One-Time Pad (OTP) technique, altering the behavior of chaotic systems. In case of an attack, this technique provides dynamic reconfiguration and resilience against adversaries.
In [90], a data compression and mapping algorithm was introduced to implement data encryption via video processing. Initially, moving objects and backgrounds were extracted through a video composition model. Then, a data encryption model was applied to facilitate effective data transmission, safeguarding against the extraction of background and moving target information. In another endeavor to secure video communication over network environments, Lin et al. [91] presented a synchronisation controller scheme. This scheme facilitates the transmission of both slave and master chaotic systems, enabling dynamic chaotic and synchronised random number generation simultaneously. While this approach efficiently secures video communication, it does not fully address robustness and reversibility concerns. To overcome these challenges, a multi-domain characteristic and Logistic-chaotic scrambling have been utilised to embed elements in encrypted videos, denoted as element-I [92]. The videos were further encoded using Bose–Chaudhuri–Hocquenghem code. Subsequently, element-I, containing robustly embedded label information, modified the amplitude of element-I, enabling the recovery of lossless auxiliary information represented as element-II. The auxiliary information was reversely embedded into element-II using traditional histogram shifting.
In another work [93], a joint operation of two linear chaotic-symmetric maps and one chaotic-tent map has been proposed. This technique permuted each frame’s pixel positions and shuffled linear symmetric-chaotic sequences using a P-box. More recently, Es-Sabry et al. [94] introduced an enhanced method for encrypting 32-bit color images using four 1D (one-dimensional) chaotic maps: Tent, Logistic, Sine, and Chebyshev. These maps carefully filled the four vectors in a cryptographic scheme, assigning unique integers between 0 and 255. They further enhance security by securing each pixel’s digits using the Four-square encryption. Additionally, they introduce an additional layer of complexity during the obfuscation stage by intentionally rearranging all pixel positions using the Arnold Cat Map transformation. An encryption system based on cellular automata (CA) and S-boxes has been proposed [95], which integrates a four-dimensional (4D) memristive hyperchaos with an additional exceptional chaotic variety, thereby enhancing uncertainty and ergodicity.
With the proliferation of the IoT across diverse domains like smart homes, cities, industries, and healthcare, conventional security techniques face inefficiencies when applied to IoT-enabled devices. These challenges arise from the computational intensity, bitrate overhead, and bandwidth utilisation inherent in conventional approaches. As a remedy, lightweight and efficient security solutions become imperative. This section delves into proposed lightweight ciphers and security techniques specifically designed to safeguard video data in the Internet of Multimedia Things (IoMT).
Lightweight Cryptographic Approaches: Recently, researchers and standardisation bodies have shown much interest in designing lightweight algorithms for secure end-to-end communication. Thus, algorithms have been proposed that employ existing SOTA encryption algorithms by reducing the number of rounds and new lightweight cryptographic algorithms. In [96], a dynamic key-dependent LoRCA cipher that comprised two rounds, one for block and another for stream cipher, was presented. Each round was implemented for a single iteration to minimise resource and computational costs. LoRCA cipher demonstrated superior performance compared to Speck by 388%, Simon by 358%, and a 100% improvement over AES. A combined encryption technique that integrates the sigmoid logistic map, Kronecker XOR product, and Hill cipher algorithms was introduced in [97]. The algorithm initially shuffled the matrix rows to the right and then applied the Hill cipher to the resulting image. Subsequently, XOR operations were performed on each value of even or odd columns. Finally, the Kronecker XOR product and sigmoid logistic map were implemented on the resulting image to enhance security and resilience against various attacks. In another work, a SLEPX (Symmetric Cipher for Lightweight Encryption based on Permutation and EXlusive OR) algorithm was proposed to encrypt the Scalable High-Efficiency-Video Coding (HEVC) videos [98]. The authors claim that their scheme offers lower computational costs than XOR and provides security approximately equivalent to AES.
Selective Features-Based Techniques: To address complexity concerns, the study [99] proposed a Selective Encryption (SE) capable of encrypting moving objects using the AES algorithm. This technique achieves high-quality encrypted videos, analyses non-encrypted statistics, and minimises computational costs. In [100], a technique based on chaotic maps and singular-value decomposition (SVD) was proposed for the SE of video feeds. SVD was utilised to select significant parts of video frame feeds containing the most crucial information. The significant frames were encrypted using the PRESENT block cipher, generating an 80-bit key size using the logistic map. In [19], the authors presented a chaotic logistic map-based encryption scheme for encrypting human faces (selected as ROI). Initially, the face is identified using the YOLO v3 detection algorithm. Subsequently, the fast-block scrambling method is applied to scramble the ROI, followed by implementing a cipher to protect the facial representation. In [18], a selective bit-stream encryption based on the chaotic Arnold map (CAM) for scalable HEVC (SHVC) was introduced. This scheme encrypts/decrypts Sample Adaptive Offset, Motion Vector Difference (MVD), and Discrete Cosine Transform (DCT) bits in AES cipher feedback (AES-CFB) operation mode. The CAM-based SHVC-SE scheme minimised encoding time compared to AES-based SHVC-SE. In [101], a message-embedding encryption technique was introduced, allowing the extraction of message bits during video decryption. The embedding of messages is achieved by altering values in reference frames and motion vectors by swapping the values of x and y components, thereby scrambling the video result. Whereas, in [102], SE based on the Rivest Cipher 4 (RC4) was proposed, ensuring correct decryption even in cases of packet loss. The scheme shuffles the non-zero coefficients using a two-round-shifting algorithm, with Quantised Transform Coefficients (QTCs), intra-prediction modes (IPMs), and MVD chosen as selective parameters.
Table 3 provides a comprehensive summary of SOTA lightweight security techniques.
Security for IoMT: IoMT encompasses multimedia devices like cameras and sensors; however, hackers may access the IoMT network or individual devices, allowing them to intercept video streams, manipulate camera settings, or even disable cameras altogether. To address the issues, researchers proposed solutions to safeguard the videos captured by resource-constrained IoMT devices. In [67], the authors apply Fernet encryption to safeguard against unauthorised access and MITM attacks. Additionally, they utilise the Diffie-Hellman protocol for secure key exchange, while the SHA256 (Secure Hash Algorithm 256-bit) verifies the authenticity of video sequences. Another work [105] introduced a lightweight SE to secure videos within the IoMT infrastructure. The proposed SE method can selectively encrypt parameters such as the sign of MVD, UEGO (Unary code and Exp-Golomb code) suffixes, a sign of NZ-TC (non-zero transform coefficient), and dQP (difference of quantisation parameters). In [110], to tackle the space and time complexity constraints of IoT devices, multi-keyed logic and logical operations like AND, XOR, and OR are employed. Additionally, to ensure the security of videos during transmission over IoT-Fog, chaining logic, Op_codes (Operational codes), and HMAC (Hash-based Message Authentication Code) is utilised. In [111], the authors utilised the PRESENT and PRINCE cryptography algorithms within the default architecture of the Reon V-processor-core to establish robust security mechanisms for IoT applications. This approach aimed to achieve high performance and encryption results. Recently, the authors [112] proposed the Elman Neural-based Blowfish-Blockchain mechanism by integrating the features of Blowfish cryptography and the Elman Neural System. The approach involves two phases: firstly, the crypto analysis phase encrypts the dataset, and secondly, the monitoring phase removes attacks from the dataset. The encrypted datasets are then stored in a cloud server. The authors presented a modified DNA-based encryption algorithm tailored for IoT devices [113]. This scheme generates a purely random secret key, enabling efficient transposition, circular shifting operations, and substitution while managing high levels of information security. Modifying the DNA-based encryption scheme enhanced the data block and key space. More recently, a technique named RAFCA (Resource Allocation Functionality with Cluster Aggregation) for securing the transmission of surveillance videos was proposed, which is based on the permutation process of the video sequence over various mobile relays [114]. In contrast, another work on a 2D (two-dimensional) 4-scroll chaotic system that can easily manage the real-time movement of data is proposed [115].
5.5 Machine and Deep Learning Techniques
In recent years, ML has garnered significant attention across various applications, particularly in cybersecurity, for detecting and predicting different types of attacks. However, leveraging its advanced capabilities also holds great potential in protecting video data. In [116], the authors applied unsupervised ML algorithm motion fusion to recognise the motion of objects, with foreground (FG) pixels and background (BG) pixels separated from each other using global thresholding. Encryption is then applied to these pixels with the ChaCha20 algorithm, where the key and nonce value are randomly generated. Another work [117] proposed an ENCVIDC ML technique that employed random forest (RF), K-nearest neighbors (K-NN), and support vector machine (SVM) algorithms to identify encrypted video with peak performance and accuracy of 98.13% in a second. Furthermore, as a subset of ML, Deep learning (DL) also benefits video security, incorporating enhanced accuracy, scalability, and automation. Therefore, researchers continually explore new DL-based methods to ensure video data security. For instance, Geng et al. [118] proposed an encryption algorithm utilising DL to detect target images from videos. This algorithm selects images based on important and non-important regions of interest, encrypting them to generate cipher images. In another study [119] the authors developed a deep convolutional neural networks (DCNN) technique by integrating the Bird-Swarm Algorithm (BSA) and Sine-Cosine Algorithm (SCA) to insert secret information into video frames. Keyframes are identified using Wavelet and Minkowski distances, with regions of interest detected by DCNN. This scheme is both efficient and less time-consuming. On the other hand, Kaczyński et al. [120] introduced watermarking into high-quality videos encoded with the H.265/HEVC codec, employing adjustable subsquares properties technique with deep neural networks (DNN). This approach yields superior results for high-quality videos compared to low-quality ones.
DNA-based encryption refers to a cryptographic approach in which cryptographic algorithms are designed to mimic the behavior of biological processes of DNA (Deoxyribo-Nucleic-Acid) molecules, such as encoding, decoding, replication, and mutation [121]. The idea behind DNA-based security is to leverage the complexity and robustness of data encryption, authentication, and access control. Researchers have explored DNA-based security techniques to assess their efficacy in securing video data. Reference [122] utilised the DNA sequence technique along with a 5D (five-dimensional) hyperchaotic system. The DNA sequence, represented by combinations of 0 and 1 s, is denoted as follows: adenine (A) represented as A = 00, cytosine (C) as C = 01, guanine (G) as G = 10, and thymine (T) as T = 11. These combinations are used to encrypt/decrypt a binary sequence. Instead, the study [123] introduced DNA sequences to generate intricate secrets and encrypted bits, thereby intensifying computation time and encryption capacity. In [124], the authors combined chaotic and DNA sequences. The scheme separated key and non-key video frames from a video sequence, with the DNA sequence employed to extract and encrypt keyframes. The authors [125] implemented DNA sequences in combination with Cellular Automata (CA) and chaotic systems on digital videos to address copyright and confidentiality concerns. The proposed scheme can be parallelly implemented on various CA rules due to one-dimensional chaotic automata based on DNA. The work [111] employed DNA cryptography and chaotic maps by generating three keys using a circle, tent, 3D (three-dimensional) logistic map, and Chebyshev in a multilevel fashion. These keys determine DNA’s encoding–decoding performance on the subblocks, used for row-column rotation of subblocks, and encryption is applied using DNA XOR operation [126] introduced a hybrid method by integrating the Mersenne Twister (MT), DNA, and Chaotic-Dynamical-Rossler (MT-DNA-Chaos), which provides enhanced security and improved efficiency of data randomness.
5.7 Techniques for Drone Videos
Drones, also known as unmanned aerial vehicles (UAVs), equipped with multispectral cameras and sensors, are utilised across various industries and applications, providing valuable insights, enhancing situational awareness [127], and facilitating live video feeds from locations and terrains that are challenging or impossible to access with traditional video-capturing methods [128]. However, they also pose potential security, safety, and privacy risks that must be addressed [129,130]. In [131], a communication of drone-based monitoring is secured through the AI-based Secure Communication and Classification for Drone-Enabled Emergency Monitoring Systems (AISCC-DE2MS). The proposed scheme uses the artificial gorilla troops optimiser (AGTO) algorithm to encrypt images using the ECC-based ElGamal encryption scheme. The AISCC-DE2MS can extract densely connected networks, hyperparameter tuning through penguin search optimisation (PESO), and perform long short-term memory (LSTM)-based classification, making it an effective tool for securing drone-based communication. The authors [132] introduce swarm-aided drone monitoring utilising distributed blockchain technology. In the proposed system, encrypted security protocols are utilised to certify security and ensure the confidentiality of information during transmission and the authenticity of UAV nodes’ identity. To mitigate attacks such as MITM and replay attacks, the authors proposed the ACPBS-IoT (Access Control Protocol for Battlefield Surveillance in drone-assisted Internet of Things) using AVISPA (Automated Validation of Internet Security Protocols and Applications) simulation tool and MIRACL (Multiprecision Integer and Rational Arithmetic Cryptographic Library) to calculate the execution time of Raspberry Pi 3 and the server [133]. In [134], control of electromagnetic fields (CEMF) technology is introduced to secure perimeters, addressing issues such as inflated alarm failure and vulnerability to weather conditions. The proposed system remains powered off until it detects people’s motion or another event. Autonomous drone technology provides much better intruder tracking and detection, overcoming false alarms and allowing cameras to be deactivated most of the time.
Homomorphic encryption (HE) is a cryptographic technique that enables operations to be performed directly on encrypted data, generating results that, when decrypted, are equivalent to those obtained from operations on plaintext [135]. This property makes HE ideal for securing video data, as it allows for secure processing, transmission, storage, and analytics of video data while maintaining its confidentiality and integrity, as presented in [136–138]. In [136], the authors proposed enhanced-fully homomorphic encryption (EFHE), utilising the fully homomorphic encryption (FHE) model. To authenticate videos in the cloud, an integrity-based FHE method was proposed by [139], where the video data was inaugurated to minimise time complexity, thus enhancing cloud services’ authentication and integrity. In [140], an architecture for enabling multimodal inference using homomorphic encryption more lightly and effectively was introduced. The Tensor Fusion Network-based HE technique was initially implemented to encrypt multimodal fusion features. After that, two methods for handling heterogeneous data, pre-expansion, and packaging, were employed, effectively reducing information traffic and temporal lag related to homomorphic computation. In [141], the authors described the Paillier encryption, based on semi-homomorphic encryption (SHE), which can measure encrypted data. While providing security guarantees and strong privacy, the proposed scheme required more energy and time. Priya et al. [142] also addressed video integrity, using the Paillier homomorphic cryptosystem for encrypting/decrypting original data. Although this scheme is secure, it increases computational complexity. In [143], a technique named RACE based on HE was proposed for encrypting/decrypting edge-to-edge communication. While providing sufficient security, it demanded more energy resources. In another study [144], the authors presented a HE scheme with pixel selection based on optimal metaheuristics (OMPS-HEVS). The introduced OMPS-HEVS converted frames and applied 2D-discrete wavelet decomposition to encode secret messages, increasing security and performance using the optimal homomorphic encryption (OHE) with the Jaya optimisation algorithm.
Watermarking is used to embed hidden information into digital media, typically a digital signal or pattern, to safeguard content integrity, authenticity, and ownership, along with providing copyright protection and content identification [145]. It can be applied at various video production and distribution stages, including content creation, post-production editing, and distribution. In videos, watermarking subtly inserts metadata such as copyright details or ownership rights into frames to identify the creator. Generally, three methods are used for watermarking video content [146]: (1) Spatial domain watermarking directly modifies the pixel values of the video frames to embed the watermark, typically used for visible watermarks overlaid on top of the video content [147]; (2) Frequency domain watermarking operates on frequency components like DCT coefficients, embedding watermarks in a less perceptible manner while maintaining robustness against signal processing operations [148]; (3) Spread Spectrum Watermarking spreads the watermark signal across the entire video signal using a pseudorandom sequence, with embedded watermarks extracted using a matching correlation process at the receiver [149]. In [150], the authors presented a hybrid compression-based digital watermarking technique that employs dual-tree complex-wavelet transform to decompose the video frame. The ECC encrypts and converts these encrypted images into binary bits and then inserts them at specified positions in the frame. The proposed technique minimises the size of the frame without affecting its quality. Another work [151] used a hybrid technique based on SE and watermarking. The scheme applies singular value decomposition and homomorphic transform in the discrete wavelet transform (DWT) to enhance the functionality of the watermarking method, achieving copyright protection and confidentiality of transmitted information. The study [152] implemented the block-based singular-value decomposition hybrid technique. The infrared and cornea frames are watermarked using SVD watermarking and dispatched through an erroneous wireless channel. The watermark scheme enhances wireless communication channels’ security, robustness, and detectability.
Cao et al. [153] presented a hyperchaotic Lorentz system-based watermarking algorithm to provide robustness and high imperceptibility. In [154], a combined watermarking and encryption based on the homomorphism scheme is proposed. The method can integrate both techniques into the same operand to provide higher security. However, this method is not helpful for Paillier encryption and Patchwork watermarking. The authors [155] proposed a tensor feature map technique based on a watermarking algorithm. The tensor feature map restrains the information of every frame, allowing watermarking to be distributed by inserting the watermark into the tensor feature map. The tensor feature map uses the discrete cosine and wavelet transforms for watermarking. Tian et al. [156] utilised a semi-fragile video watermarking technique based on chromatic-DCT. The scheme can increase bit rate, robustness, and video quality. The authors Zhang et al. [157] presented a watermarked-based time factor matrix adjusting the initial column of the time factor matrix and thus can protect against scaling, rotation, cropping, video compression, and frame deletion. The scheme converts the host video into three-factor matrices and a core tensor, representing the frame in the column, row, and time-axis direction by implementing Tucker decomposition. Du et al. [158] proposed a watermarking technique based on Tensor-QR decomposition (T-QR) and human-visual system (HVS). In [159], videos were protected by implementing a watermarking technique based on a pseudo-three-dimensional cosine transform (pseudo-3D-DCT), non-negative matrix factorisation (NMF), and non-subsampled contourlet transform (NSCT). The proposed technique provides invisibility of the watermark, robustness, and high ability against combined attacks.
5.10 Blockchain-Based Video Protection
Blockchain is an emerging technology that can store or manage a massive amount of data and prevent unauthorised access to the data. In [160], the authors proposed a two-level blockchain system to ensure the integrity of the videos. The proposed systems divide digital evidence into cold and hot blockchains. In the investigation process, the frequently changed information is stored in the hot blockchain, while unchanged information is stored in the cold blockchain. Another blockchain-based technique was proposed to distinguish the original videos from fake ones, thus ensuring authenticity [161]. To address issues related to centralised security systems, the authors [162] proposed a distributed-video surveillance method based on blockchain. The technique protects privacy, consumes fewer resources, maintains integrity, and manages blurring keys. The study [163] utilised blockchain based on the Merkle-Tree method, which can efficiently transmit video data, reduce required bandwidth, minimise storage costs, and safely synchronise CCTV (Closed-Circuit Television) video. In another work, the authors [164] proposed an algorithm based on the secure multiparty computation (SMC) blockchain that can accurately verify and authenticate video data token records. In [165], the authors provide design guidelines for blockchain-based DRM solutions that enable visible licensing of music frameworks, consistent and comprehensive rights metadata, and efficient and visible royalty distribution. Three methods are used to accomplish the solution: (1) putting rights metadata on a publicly distributed ledger, (2) using a consensus process on a blockchain with permission to validate metadata, and (3) using a smart contract to enforce royalty payouts using stablecoin. The study [166] authenticate the integrity of VVC (Versatile Video Coding) by implementing the concept of an ON and OFF chain. First, the video hash is calculated through HMAC; afterward, the calculated hash and the encryption key are stored on the ON chain. Second, the selective features of the video are encrypted through SLEPX and then stored on the OFF chain.
5.11 Digital Right Management-Based Video Security
Digital Rights Management (DRM) refers to techniques for addressing intellectual property rights issues. It can prevent unauthorised copying, distribution, and modification of digital content [167]. Researchers have employed DRM for video data in a few ways beyond simply applying commercial DRM solutions. In [168], the authors proposed a SE for H.264/AVC videos integrated with DRM techniques. This method encrypts the video texture during compression using DCT coefficients, ensuring high encryption speed, robust security, and suitability for DRM and industrial applications. In [169], the authors introduced DRM by implementing Secure Digital Camera (SDC) rights at the sender end for video data. The scheme utilises digital watermarking and encryption to embed binary images into the video framework and authenticate integrity using a unique key. In [170], a DRM is presented to address copyright issues in video systems by employing the AES for encryption/decryption. In another study [171], a streaming-based DRM solution is proposed using the JSON Web Token (JWT) technique to verify individual identification. The server’s RSA secret key encrypts query variables, while the client provides a set of public and private keys. The video employs adaptive protection with AES-128, generating a new key with each performance to enhance security.
5.12 Generative AI-Based Security
More recently, generative adversarial networks (GANs) offer a range of applications in video security, from detecting deepfake [70] for enhancing video quality, detecting anomalies [172], generating synthetic data [67], preserving privacy [173,174], and authenticating video content. By leveraging the capabilities of GANs, video security can become more robust, accurate, and reliable, addressing many challenges posed by modern security threats. For instance, in [70], the authors proposed a lightweight deepfake detection model for video conference applications to detect fake faces. It employs the MTCNN (Multi-task Cascaded Convolutional Networks) method for deepfake detection, demonstrating that the model can detect faces quickly and with acceptable accuracy, outperforming traditional methods. Additionally, the use of an Inception-Resnet model enhances performance results. In [67], the authors created synthetic data to comply with privacy regulations for objects/individuals and generate a large dataset that is difficult to collect in a real-time environment. In [174], the authors introduced a framework called Privacy-Protective-GAN (PP-GAN) for face de-identification that incorporates verification and regulator modules, addressing the limitations of traditional methods like the k-same framework, which suffers from low effectiveness and poor visual quality. The verification ensures that face recognition models cannot recognise the generated faces, while the regulator ensures that the generated faces retain the same structure as the input faces. This means that the output faces, while looking similar to the input, cannot be used to identify the original individual, thereby preserving privacy. Balancing these two aspects makes it a powerful tool for applications where privacy is a concern, such as in public surveillance or social media platforms. Whereas, in [175], a GAN-based technique is employed to train a synthetic dataset for deepfake videos, using 132,000 video frames extracted from John Oliver’s YouTube videos. In [176], the authors proposed a recurrent neural network-based technique to detect video manipulations, utilising 300 deepfake films from various video-hosting websites. An additional 300 videos randomly chosen from the HOHA dataset were also incorporated, resulting in a final dataset comprising 600 videos.
We evaluated the performance of the security approaches presented above by considering the structure of the security system, the Encryption Space Ratio (ESR), which indicates the robustness of the encryption, the attacks the approach is designed to counter, and the computational efficiency in terms of speed, which significantly impacts the proposed methods. Table 4 presents a comparative analysis of these security schemes.
Table 5 presents the summary of some quality metrics considered for evaluating the proposed approaches, whereas the detailed descriptions of these metrics can be found in [19,195]. Click or tap here to enter text.-Click or tap here to enter text. These metrics include SSIM (Structural Similarity Index), PSNR (Peak Signal-to-Noise Ratio), VMAF (Video Multi-Method Assessment Fusion), MSE (Mean Squared Error), PESQ (Perceptual Evaluation of Speech Quality, NPCR (Number of Pixels Change Rate), UACI (Unified Average Changing Intensity), FSIM (Feature Similarity Index).
Table 6 presents a quantitative evaluation using video quality metrics. These metrics collectively provide a robust framework for evaluating the performance of security algorithms, ensuring that the integrity and confidentiality of video data are maintained without compromising quality.
The findings of this survey indicate that even though a significant effort has been made to address the security and privacy challenges inherent in video data, none have proven entirely proficient in ensuring end-to-end security. Each method discussed in this paper presents its own set of strengths and weaknesses. For instance, video encryption employs cryptographic algorithms to prevent unauthorised access and maintain confidentiality, yet it also amplifies computational costs, consumes considerable CPU/GPU (Graphic Processing Unit) resources for processing, and demands higher bandwidth requirements. In response, SE-based schemes have been developed to encrypt portions of videos selectively, but they do not provide sufficient security. Alternatively, chaotic-based encryption offers a solution to enhance video system security, albeit with the drawback of complex key management. The research indicates that as data volumes grow, processing complexity correspondingly increases [15]. Additionally, the constraints of key space present significant concerns. For instance, many XOR-based encryption schemes depend on pseudo-random generators (PRGs) to generate randomness [203]. While ML and DL provide substantial advantages for video security, they also introduce hurdles, such as the need for extensive data for training, which can be challenging to obtain. Moreover, the inherent opacity of these algorithms complicates understanding their decision-making processes. Table 7 presents the advantages and limitations of various video security techniques.
Despite numerous efforts to strengthen video data security using emerging technologies against evolving threats, securing videos remains a persistent challenge. In this section, we discuss some of the open problems to proactively address emerging and protected videos, as illustrated in Fig. 6. By embracing these advancements, video data producers and service providers can navigate the balance between data utility and privacy, tailored to specific use cases, legal requirements, and privacy thresholds.
Figure 6: Future trends for video security
7.1 AI-Driven Threat Detection
The use of AI and ML for video security is an actively growing research area, as discussed earlier. However, AI-driven detection, such as forgery detection, identifying real-time attacks, predictive threat modeling, and automatically responding to security incidents, remains complex and still lacks accuracy. Moreover, in cases where malicious actors manipulate or perturb input video data to deceive ML models, defenses against these adversarial attacks [204], particularly those resistant to multi-perturbation are still in their nascency.
Edge-based security refers to securing data by leveraging the edge-computing paradigm [205] to perform tasks such as encryption, decryption, content filtering, anonymisation, and threat intelligence directly at edge devices where the videos are generated. This ensures that sensitive video content remains secure throughout the video delivery process (End-to-end), thereby protecting individual privacy and ensuring regulatory compliance. For example, in [206], privacy concerns are addressed while enabling effective surveillance at the edge. However, edge devices typically have limited processing power and storage capacity compared to centralised servers. This can restrict the complexity and scale of security operations that can be performed at the edge due to computational and bandwidth requirements. These challenges necessitate the need for efficient, equally robust security measures and careful consideration of the specific requirements and constraints tailored to the edge computing paradigm while dealing with the videos.
7.3 Explainable Security (XSec)
Inspired by Explainable Artificial Intelligence (XAI) [207], XSec represents a novel paradigm in security research, aiming to enhance transparency, understanding, and trust in security mechanisms. It achieves this by providing clear explanations for their behavior, decisions, and vulnerabilities [208]. XSec’s application in video security systems marks a promising research area that integrates cutting-edge technologies to boost privacy and trust significantly. For instance, an XSec approach could be incorporated into security systems to explain the reasons behind the encryption of specific video feeds and control decisions and the effects of various security measures on the integrity of video data, thereby achieving the balance between security and transparency. However, XSec must be seamlessly integrated into current security workflows without causing disruptions or exposing sensitive data through explanations.
7.4 Privacy-Enhancing Technologies (PETs)
Video data often contains sensitive contextual information beyond faces, such as location, activities, or interactions. Preserving privacy while retaining this contextual information presents an ongoing research challenge. Simply blurring faces or other identifying features may prove insufficient to protect privacy, particularly with advancements in Facial Recognition Technology (FRT) [209]. Therefore, further research is necessary to explore more robust methods, such as Privacy-Enhancing Technologies (PETs), that maintain privacy while retaining the usefulness of the video data. PETs encompass various strategies, including homomorphic encryption, Secure Multiparty Computation (SMPC), Federated Learning, Trusted Execution Environments (TEEs), and Differential Privacy (DP), which minimise the collection, processing, and storage of personal data while reducing the risk of privacy breaches. Integrating these technologies will enable organisations to implement data protection measures by design and default, aligning with recommendations from General Data Protection Regulation (GDPR) while respecting privacy rights.
7.5 Quantum-Safe Cryptography (QSC)
QSC, also known as Post-Quantum Cryptography (PQC) [210], involves designing and implementing cryptographic algorithms that are resistant to attacks from quantum computers. While some quantum-safe algorithms, such as lattice-based cryptography and hash-based signatures have been proposed [211]. Their practicality and efficiency in securing video data require a thorough investigation. Research is needed to explore the impact of quantum computing on video data and develop and optimise quantum-safe algorithms and protocols to protect videos against future quantum attacks.
The evolution of technologies like AI and IoT necessitates human-centric security approaches to safeguard video data. Users should ideally maintain good cyber hygiene [212] to protect data from hackers, yet many exhibit poor habits, such as sharing passwords and personal information on social networks [213]. Hackers exploit this vulnerability, making user information their easiest target. Thus, there is a need to recognise the importance of human-centric security in organisational culture, fostering collective responsibility. Traditional cybersecurity training focuses on dos and don’ts. In contrast, the human-centric approach delves into behaviour-based training, helping users understand the rationale behind security measures. Strategies like user authentication and security awareness programs are vital for building resilience against cyberattacks.
7.7 Regulatory Compliance and Standards
Increasing regulatory obligations and government controls over privacy and data localisation can significantly impact video data security. Adherence to regulations like GDPR (General Data Protection Regulation) [214], AI Act [215], CCPA (California Consumer Privacy Act) [216], HIPAA (Health Insurance Portability and Accountability Act) [217], and ISO (International Organisation for Standardisation)/IEC (International Electrotechnical Commission) 27001 [218] are crucial to avoid legal issues and maintain trust, as they impose requirements for transparency, consent, data protection, and accountability. Thus, there is a pressing need to investigate the evolving impact of regulations and industry standards on video data security and to develop compliance frameworks tailored to the unique challenges of securing video data. By addressing these research problems, organisations can effectively navigate the evolving regulatory landscape and implement robust security measures to safeguard videos while ensuring compliance with regulations and industry standards.
Security threats to video data encompass a wide range of risks, including unauthorised access, data breaches, content manipulation, piracy, surveillance, network attacks, insider threats, machine learning attacks, and vulnerabilities in cloud security. Addressing these threats effectively requires a multifaceted approach, integrating technological countermeasures such as encryption, access controls, authentication mechanisms, secure transmission protocols, blockchain, watermarking, DRM, and robust cybersecurity policies. This survey distinguishes itself from other works through its distinct approach, offering a dual focus by examining both, i.e., how video data can be attacked and which cutting-edge technology can safeguard it. Initially, this work meticulously reviews manipulation techniques and attacks on video data and their impacts while thoroughly examining prevalent security challenges for safeguarding videos, encompassing both continuous (e.g., surveillance videos) and noncontinuous (e.g., VoD) formats. Secondly, it provides insights into cutting-edge technological advancements, specifically emphasising the enhancement of video security. The study analyses these security approaches and identifies several challenges, including computational complexity, efficient key management, limitations in parallel processing, intensive resource requirements, and transparency issues. Moreover, it outlines open research challenges for videos, introducing fresh perspectives and innovative solutions such as AI-driven threat detection, edge computing security, and XSec. By focusing on these future directions, researchers can contribute to the development of innovative solutions and best practices for securing video data in an increasingly complex and interconnected world.
Acknowledgement: The authors are grateful to all the editors and anonymous reviewers for their comments and suggestions.
Funding Statement: This research is conducted under the project funded by the European Union’s Horizon 2020 Research and Innovation Programme under the Marie Skłodowska-Curie Action (MSCA) grant agreement No. 101109961.
Author Contributions: The authors confirm their contribution to the paper as follows: Study conception and design: Amna Shifa, Ali Asghar; Data collection: Ali Asghar, Amna Shifa; Analysis and interpretation of methods: Amna Shifa, Ali Asghar; Draft manuscript preparation: Ali Asghar, Amna Shifa, Mamoona Naveed Asghar; Review and editing: Amna Shifa, Mamoona Naveed Asghar; Funding acquisition: Mamoona Naveed Asghar, Amna Shifa. All authors reviewed the results and approved the final version of the manuscript.
Availability of Data and Materials: Not applicable.
Ethics Approval: Not applicable.
Conflicts of Interest: The authors declare that they have no conflicts of interest to report regarding the present study.
References
1. C. Liu, T. Zhu, J. Zhang, and W. Zhou, “Privacy intelligence: A survey on image privacy in online social networks,” ACM Comput. Surv., vol. 55, no. 8, pp. 1–35, 2022. doi: 10.1145/3547299. [Google Scholar] [CrossRef]
2. L. Weinberger, C. Eichenmüller, and Z. Benenson, “Interplay of security, privacy and usability in videoconferencing,” in Ext. Abstr. 2023 CHI Conf. Human Factors Comput. Syst. (CHI EA ’23), Hamburg, Germany, Apr. 23–28, 2023, pp. 1–10. doi: 10.1145/3544549.3585683. [Google Scholar] [CrossRef]
3. P. Bump, “How video consumption is changing in 2023,” Hubspot. Accessed: Jul. 25, 2024. [Online]. Available: https://blog.hubspot.com/marketing/how-video-consumption-is-changing [Google Scholar]
4. CISCO, “Cisco Annual Internet Report (2018–2023) White Paper,” Cisco. Accessed: Jul. 25, 2024. [Online]. Available: https://www.cisco.com/c/en/us/solutions/collateral/executive-perspectives/annual-internet-report/white-paper-c11-741490.html [Google Scholar]
5. S. M. Gillani, M. N. Asghar, A. Shifa, S. Abdullah, N. Kanwal and M. Fleury, “VQProtect: Lightweight visual quality protection for error-prone selectively encrypted video streaming,” Entropy, vol. 24, no. 6, 2022, Art. no. 755. doi: 10.3390/e24060755. [Google Scholar] [CrossRef]
6. T. Winkler and B. Rinner, “Privacy and security in video surveillance,” in Intelligent Multimedia Surveillance: Current Trends and Research, Berlin, Germany: Springer-Verlag, 2013, pp. 37–66. [Google Scholar]
7. J. Coker, “Russia spies on Kyiv defenses via hacked cameras before missile strike,” Infosecurity magazine. Accessed: Apr. 16, 2024. [Online]. Available: https://www.infosecurity-magazine.com/news/russia-spies-kyiv-hacked-cameras/ [Google Scholar]
8. Y. Kelli, “Cyber attack case study: Deepfake scammers con company,” CoverLink Insurance. Accessed: Jul. 14, 2024. [Online]. Available: https://coverlink.com/case-study/cyber-attack-case-study-deepfake-scammers-con-company/ [Google Scholar]
9. Google, “Blur your videos,” YouTube Help. Accessed: Apr. 14, 2024. [Online]. Available: https://support.google.com/youtube/answer/9057652?hl=en [Google Scholar]
10. A. Bencherqui et al., “Optimal algorithm for color medical encryption and compression images based on DNA coding and a hyperchaotic system in the moments,” Eng. Sci. Technol., Int. J., vol. 50, 2024, Art. no. 101612. doi: 10.1016/j.jestch.2023.101612. [Google Scholar] [CrossRef]
11. F. Liu and H. Koenig, “A survey of video encryption algorithms,” Comput. Secur., vol. 29, no. 1, pp. 3–15, 2010. doi: 10.1016/j.cose.2009.06.004. [Google Scholar] [CrossRef]
12. J. R. Padilla-López, A. A. Chaaraoui, and F. Flórez-Revuelta, “Visual privacy protection methods: A survey,” Expert. Syst. Appl., vol. 42, no. 9, pp. 4177–4195, 2015. doi: 10.1016/j.eswa.2015.01.041. [Google Scholar] [CrossRef]
13. Z. Lv, L. Qiao, and H. Song, “Analysis of the security of Internet of Multimedia Things,” ACM Trans. Multimed. Comput., Commun., Appl. (TOMM), vol. 16, no. 3s, pp. 1–16, 2020. doi: 10.1145/3398201. [Google Scholar] [CrossRef]
14. M. S. Alsayfi, M. Y. Dahab, F. E. Eassa, R. Salama, S. Haridi and A. S. Al-Ghamdi, “Securing real-time video surveillance data in vehicular cloud computing: A survey,” IEEE Access, vol. 10, pp. 51525–51547, 2022. doi: 10.1109/ACCESS.2022.3174554. [Google Scholar] [CrossRef]
15. K. M. Hosny, M. A. Zaki, N. A. Lashin, M. M. Fouda, and H. M. Hamza, “Multimedia security using encryption: A survey,” IEEE Access, vol. 11, pp. 63027–63056, 2023. doi: 10.1109/ACCESS.2023.3287858. [Google Scholar] [CrossRef]
16. J. Y. Yu, Y. Kim, and Y. G. Kim, “Intelligent video data security: A survey and open challenges,” IEEE Access, vol. 9, pp. 26948–26967, 2021. doi: 10.1109/ACCESS.2021.3057605. [Google Scholar] [CrossRef]
17. A. Tekalp, Digital Video Processing, 2nd ed., USA: Prentice Hall Press, 2015. Accessed: Apr. 16, 2024. [Online]. Available: https://dl.acm.org/doi/abs/10.5555/2843012 [Google Scholar]
18. O. S. Faragallah, A. I. Sallam, M. Alajmi, and H. S. El-Sayed, “Efficient selective chaotic video stream cipher for SHVC bitstream,” Multimed. Tools Appl., vol. 82, no. 20, pp. 30689–30708, 2023. doi: 10.1007/s11042-023-14517-8. [Google Scholar] [CrossRef]
19. K. M. Hosny, M. A. Zaki, H. M. Hamza, M. M. Fouda, and N. A. Lashin, “Privacy protection in surveillance videos using block scrambling-based encryption and DCNN-based face detection,” IEEE Access, vol. 10, pp. 106750–106769, 2022. doi: 10.1109/ACCESS.2022.3211657. [Google Scholar] [CrossRef]
20. W. El-Shafai, M. A. Fouda, E. S. M. El-Rabaie, and N. A. El-Salam, “A comprehensive taxonomy on multimedia video forgery detection techniques: Challenges and novel trends,” Multimed. Tools Appl., vol. 83, no. 2, pp. 4241–4307, 2024. doi: 10.1007/s11042-023-15609-1. [Google Scholar] [PubMed] [CrossRef]
21. A. Rehman, Z. Jalil, W. Zehra, T. Reddy, D. Young and J. Piran, “A comprehensive survey on digital video forensics: Taxonomy, challenges, and future directions,” Eng. Appl. Artif. Intell., vol. 106, pp. 1–14, 2021, Art. no. 104456. doi: 10.1016/j.engappai.2021.104456. [Google Scholar] [CrossRef]
22. P. Aberna and L. Agilandeeswari, “Digital image and video watermarking: Methodologies, attacks, applications, and future directions,” Multimed. Tools Appl., vol. 83, no. 2, pp. 5531–5591, 2024. doi: 10.1007/s11042-023-15806-y. [Google Scholar] [CrossRef]
23. E. Hu, J. E. S. Grønbæk, W. Ying, R. Du, and S. Heo, “ThingShare: Ad-Hoc digital copies of physical objects for sharing things in video meetings,” in Proc. 2023 CHI Conf. Human Factors Comput. Syst. (CHI ’23), Hamburg, Germany, Apr. 23–28, 2023, pp. 1–22. doi: 10.1145/3544548.3581148. [Google Scholar] [CrossRef]
24. Y. Akbari, A. L. A. Najeeb, S. A. L. Maadeed, S. Member, O. Elharrouss and F. Khelifi, “A new dataset for forged smartphone videos detection: Description and analysis,” IEEE Access, vol. 11, pp. 70387–70395, 2023. doi: 10.1109/ACCESS.2023.3267743. [Google Scholar] [CrossRef]
25. Z. Geradts and Q. Riphagen, “Interpol review of forensic video analysis, 2019–2022,” Forensic Sci. Int., vol. 6, 2023, Art no. 100309. doi: 10.1016/j.fsisyn.2022.100309. [Google Scholar] [PubMed] [CrossRef]
26. S. Karnouskos, “Artificial Intelligence in digital media: The era of deepfakes,” IEEE Trans. Technol. Soc., vol. 1, no. 3, pp. 138–147, 2020. doi: 10.1109/TTS.2020.3001312. [Google Scholar] [CrossRef]
27. K. Patel, H. Han, A. K. Jain, and G. Ott, “Live face video vs. spoof face video: Use of moiré patterns to detect replay video attacks,” in 2015 Int. Conf. Biom. (ICB), Phuket, Thailand, May 19–22, 2015, pp. 98–105. doi: 10.1109/ICB.2015.7139082. [Google Scholar] [CrossRef]
28. M. Conti, E. Losiouk, and A. Visintin, “What you see is not what you get: A man-in-the-middle attack applied to video channels,” in Proc. 37th ACM/SIGAPP Symp. Appl. Comput. (SAC ’22), New York, NY, USA, Apr. 25–29, 2022, pp. 1723–1726. doi: 10.1145/3477314.3507233. [Google Scholar] [CrossRef]
29. A. Fitwi, Y. Chen, S. Zhu, E. Blasch, and G. Chen, “Privacy-preserving surveillance as an edge service based on lightweight video protection schemes using face de-identification and window masking,” Electronics, vol. 10, no. 3, pp. 1–36, 2021. doi: 10.3390/electronics10030236. [Google Scholar] [CrossRef]
30. X. Xu, H. Hu, Y. Liu, J. Tan, H. Zhang and H. Song, “Moving target defense of routing randomization with deep reinforcement learning against eavesdropping attack,” Digit. Commun. Netw., vol. 8, no. 3, pp. 373–387, 2022. doi: 10.1016/j.dcan.2022.01.003. [Google Scholar] [CrossRef]
31. D. Zhu, J. Zheng, H. Zhou, J. Wu, N. Li and L. Song, “A hybrid encryption scheme for quantum secure video conferencing combined with blockchain,” Mathematics, vol. 10, no. 17, pp. 1–24, 2022. doi: 10.3390/math10173037. [Google Scholar] [CrossRef]
32. X. Yan, X. Chen, Y. Jiang, S. T. Xia, Y. Zhao and F. Zheng, “Hijacking tracker: A powerful adversarial attack on visual tracking,” in 2020 IEEE Int. Conf. Acoust., Speech Signal Process. (ICASSP), Barcelona, Spain, May 4–8, 2020, pp. 2897–2901. doi: 10.1109/ICASSP40776.2020.9053574. [Google Scholar] [CrossRef]
33. J. Kim and N. Park, “Lightweight knowledge-based authentication model for intelligent closed circuit television in mobile personal computing,” Pers Ubiquitous Comput., vol. 26, no. 2, pp. 345–353, 2022. doi: 10.1007/s00779-019-01299-w. [Google Scholar] [CrossRef]
34. F. Tommasi, C. Catalano, and I. Taurino, “Browser-in-the-Middle (BitM) attack,” Int. J. Inf. Secur., vol. 21, no. 2, pp. 179–189, 2022. doi: 10.1007/s10207-021-00548-5. [Google Scholar] [CrossRef]
35. K. Mostefa, M. Kara, A. Laouid, M. Alshaikh, A. Bounceur and M. Hammoudeh, “Secure key exchange against man-in-the-middle attack: Modified diffie-hellman protocol,” Jurnal Ilmiah Teknik Elektro Komputer dan Informatika, vol. 7, no. 3, pp. 380–387, 2021. doi: 10.26555/jiteki.v7i3.22210. [Google Scholar] [CrossRef]
36. N. Pan, J. Qin, Y. Tan, X. Xiang, and G. Hou, “A video coverless information hiding algorithm based on semantic segmentation,” EURASIP J. Image Vide., vol. 2020, no. 1, pp. 1–18, 2020. doi: 10.1186/s13640-020-00512-8. [Google Scholar] [CrossRef]
37. A. G. Yılmaz, U. Turhal, and V. Nabiyev, “Face presentation attack detection performances of facial regions with multi-block LBP features,” Multimed. Tools Appl., vol. 82, no. 26, pp. 40039–40063, 2023. doi: 10.1007/s11042-023-14453-7. [Google Scholar] [PubMed] [CrossRef]
38. D. Karmakar, R. Sarkar, and M. Datta, “Spoofed replay attack detection by multidimensional Fourier transform on facial micro-expression regions,” Signal Process Image Commun., vol. 93, 2021, Art no. 116164. doi: 10.1016/j.image.2021.116164. [Google Scholar] [CrossRef]
39. J. Obermaier and M. Hutle, “Analyzing the security and privacy of cloud-based video surveillance systems,” in Proc. 2nd ACM Int. Workshop IoT Priv., Trust, Secur. (IoTPTS’16), Xi’an, China, May 30, 2016, pp. 22–28. doi: 10.1145/2899007.2899008. [Google Scholar] [CrossRef]
40. N. Kalbo, Y. Mirsky, A. Shabtai, and Y. Elovici, “The security of IP-based video surveillance systems,” Sensors, vol. 20, no. 17, pp. 1–27, 2020. doi: 10.3390/s20174806. [Google Scholar] [PubMed] [CrossRef]
41. K. H. Chow et al., “Adversarial objectness gradient attacks in real-time object detection systems,” in 2020 Second IEEE Int. Conf. Trust, Priv. Secur. Intell. Syst. Appl. (TPS-ISA), Atlanta, GA, USA, Oct. 28–31, 2020, pp. 263–272. doi: 10.1109/TPS-ISA50397.2020.00042. [Google Scholar] [CrossRef]
42. P. Kong and S. Member, “A survey of cyberattack countermeasures for unmanned aerial vehicles,” IEEE Access, vol. 9, pp. 148244–148263, 2021. doi: 10.1109/ACCESS.2021.3124996. [Google Scholar] [CrossRef]
43. F. Arab and S. M. Abdullah, “A robust video watermarking technique for the tamper detection of surveillance systems,” Multimed. Tools Appl., vol. 5, pp. 1–31, 2015. doi: 10.1007/s11042-015-2800-5. [Google Scholar] [CrossRef]
44. D. R. dos Santos, M. Dagrada, and E. Costante, “Leveraging operational technology and the internet of things to attack smart buildings,” J. Comput. Virol. Hacking Tech., vol. 17, no. 1, pp. 1–20, 2021. doi: 10.1007/s11416-020-00358-8. [Google Scholar] [CrossRef]
45. A. A. Azeezat, O. S. Adebukola, A. A. Adebayo, and O. B. Olushola, “A conceptual hybrid model of Deep Convolutional Neural Network (DCNN) and Long Short-Term Memory (LSTM) for masquerade attack detection,” Commun. Comput. Inform. Sci., vol. 1350, pp. 170–184, 2021. doi: 10.1007/978-3-030-69143-1. [Google Scholar] [CrossRef]
46. S. Ramesh, C. Yaashuwanth, K. Prathibanandhi, A. R. Basha, and T. Jayasankar, “An optimized deep neural network based DoS attack detection in wireless video sensor network,” J. Ambient Intell. Humaniz. Comput., vol. 1, pp. 1–14, 2021. doi: 10.1007/s12652-020-02763-9. [Google Scholar] [CrossRef]
47. N. Sufyan, N. A. Saqib, and M. Zia, “Detection of jamming attacks in 802.11b wireless networks,” EURASIP J. Wirel. Commun. Netw., vol. 2013, no. 1, pp. 1–18, 2013. doi: 10.1186/1687-1499-2013-208. [Google Scholar] [CrossRef]
48. M. Ashourian and O. Sharifi-Tehrani, “Application of semi-circle law and Wigner spiked-model in GPS jamming confronting,” Signal Image Video P., vol. 17, no. 3, pp. 687–694, 2022. doi: 10.1007/s11760-022-02276-2. [Google Scholar] [CrossRef]
49. P. Vennam, T. C. Pramod, B. M. Thippeswamy, Y. G. Kim, and B. N. Pavan Kumar, “Attacks and preventive measures on video surveillance systems: A review,” Appl. Sci., vol. 11, no. 12, 2021, Art no. 5571. doi: 10.3390/app11125571. [Google Scholar] [CrossRef]
50. X. Wei, C. Sun, M. Lyu, Q. Song, and Y. Li, “ConstDet: Control semantics-based detection for GPS spoofing attacks on UAVs,” Remote Sens., vol. 14, no. 21, pp. 1–23, 2022. doi: 10.3390/rs14215587. [Google Scholar] [CrossRef]
51. S. G. Nam, Y. Jang, D. G. Lee, and Y. S. Seo, “Hybrid features by combining visual and text information to improve spam filtering performance,” Electronics, vol. 11, no. 13, 2022, Art no. 2053. doi: 10.3390/electronics11132053. [Google Scholar] [CrossRef]
52. N. Pitropakis, E. Panaousis, T. Giannetsos, E. Anastasiadis, and G. Loukas, “A taxonomy and survey of attacks against machine learning,” Comput. Sci. Rev., vol. 34, 2019, Art no. 100199. doi: 10.1016/j.cosrev.2019.100199. [Google Scholar] [CrossRef]
53. M. U. Ilyas and S. A. Alharbi, “Machine learning approaches to network intrusion detection for contemporary internet traffic,” Computing, vol. 104, no. 5, pp. 1061–1076, 2022. doi: 10.1007/s00607-021-01050-5. [Google Scholar] [CrossRef]
54. O. Salman, I. H. Elhajj, A. Chehab, and A. Kayssi, “A machine learning based framework for IoT device identification and abnormal traffic detection,” Trans. Emerg. Telecomm. Technol., vol. 33, no. 3, pp. 1–15, 2022. doi: 10.1002/ett.3743. [Google Scholar] [CrossRef]
55. J. Ferryman and A. Ellis, “PETS2010: Dataset and challenge,” in 2010 7th IEEE Int. Conf. Adv. Video Signal Based Surveill., Boston, MA, USA, Aug. 29–Sep. 1, 2010, pp. 143–150. doi: 10.1109/AVSS.2010.90. [Google Scholar] [CrossRef]
56. J. Jodoin, G. Bilodeau, and N. Saunier, “Urban tracker: Multiple object tracking in Urban mixed traffic,” in IEEE Winter Conf. Appl. Comput. Vis., Steamboat Springs, CO, USA, Mar. 24–26, 2014, pp. 885–892. doi: 10.1109/WACV.2014.6836010. [Google Scholar] [CrossRef]
57. A. Milan, L. Leal-Taixe, I. Reid, S. Roth, and K. Schindler, “MOT17: A benchmark for multi-object tracking,” 2016, arXiv:1603.00831. [Google Scholar]
58. Xiph, “Derf’s Test Media Collection,” Xiph.org. Accessed: Jul. 14, 2024. [Online]. Available: https://media.xiph.org/video/derf/ [Google Scholar]
59. S. Moccia et al, “NBI-InfFrames Datasets,” NEARLab. Accessed: Jul. 14, 2024. [Online]. Available: https://nearlab.polimi.it/medical/dataset/ [Google Scholar]
60. ASU, “YUV Video Sequences,” Video Trace Library. Accessed: Jul. 14, 2024. [Online]. Available: http://trace.eas.asu.edu/yuv/index.html [Google Scholar]
61. K. Müller and V. Anthony, “Common test conditions of 3D-MVV core experiments,” in Joint Collab. Team 3D Video Coding Ext. (JCT3V-G1100), 7th Meeting, San Jose, USA, 2014. [Google Scholar]
62. European Broadcasting Union (EBU) “UHD-1,” EBU Technology & Innovation. Accessed: Jul. 14, 2024. [Online]. Available: https://tech.ebu.ch/testsequences/uhd-1 [Google Scholar]
63. USC-SIPI, “SIPI Image Database,” University of Southern California (USC). Accessed: Jul. 14, 2024. [Online]. Available: https://sipi.usc.edu/database/ [Google Scholar]
64. K. Lin et al., “ABODA: Abandoned Object Dataset,” Github. Accessed: Jul. 14, 2024. [Online]. Available: https://github.com/kevinlin311tw/ABODA [Google Scholar]
65. A. Alarifi, S. Sankar, T. Altameem, K. C. Jithin, M. Amoon and W. El-Shafai, “A novel hybrid cryptosystem for secure streaming of high efficiency H.265 compressed videos in IoT multimedia applications,” IEEE Access, vol. 8, pp. 128548–128573, 2020. doi: 10.1109/ACCESS.2020.3008644. [Google Scholar] [CrossRef]
66. BeamNG.drive, “Home” BeamNG drive. Accessed: Jul. 14, 2024. [Online]. Available: https://www.beamng.com/game/ [Google Scholar]
67. M. Tahir, Y. Qiao, N. Kanwal, B. Lee, and M. N. Asghar, “Privacy preserved video summarization of road traffic events for IoT smart cities,” Cryptography, vol. 7, no. 1, 2023, Art. no. 7. doi: 10.3390/cryptography7010007. [Google Scholar] [CrossRef]
68. Q. Cao, L. Shen, W. Xie, O. M. Parkhi, and A. Zisserman, “VGGFace2: A dataset for recognising faces across pose and age,” in 2018 13th IEEE Int. Conf. Automatic Face Gesture Recognit. (FG 2018), Xi’an, China, May 15–19, 2018, pp. 67–74. doi: 10.1109/FG.2018.00020. [Google Scholar] [CrossRef]
69. B. Dolhansky et al., “The Deepfake Detection Challenge (DFDC) preview dataset,” 2019, arXiv:1910.08854. [Google Scholar]
70. A. S. Ucan, F. Mustafa, M. A. H. Tutuk, I. H. Aydin, E. Semiz and S. Bahtiyar, “Deepfake and security of video conferences,” in 2021 6th Int. Conf. Comput. Sci. Eng. (UBMK), Ankara, Turkey, Sep. 15–17, 2021, pp. 36–41. doi: 10.1109/UBMK52708.2021.9558963. [Google Scholar] [CrossRef]
71. J. Zhou and C. M. Pun, “Personal privacy protection via irrelevant faces tracking and pixelation in video live streaming,” IEEE Trans. Inf. Forensics Secur., vol. 16, pp. 1088–1103, 2021. doi: 10.1109/TIFS.2020.3029913. [Google Scholar] [CrossRef]
72. T. Winkler and B. Rinner, “Privacy protection vs. utility in visual data: An objective evaluation framework,” Multimed. Tools Appl., vol. 77, pp. 2285–2312, 2018. doi: 10.1007/s11042-016-4337-7. [Google Scholar] [CrossRef]
73. M. Boyle, C. Edwards, and S. Greenberg, “The effects of filtered video on awareness and privacy,” in Proc. ACM Conf. Comput. Support. Coop. Work (CSCW’00), Philadelphia, PA, USA, Dec. 2000, pp. 1–10. doi: 10.1145/358916.358935. [Google Scholar] [CrossRef]
74. J. Zhou, C. M. Pun, and Y. Tong, “Privacy-sensitive objects pixelation for live video streaming,” in Proc. 28th ACM Int. Conf. Multimed. (MM 2020), Seattle, WA, USA, Oct. 12–16, 2020, pp. 3025–3033. doi: 10.1145/3394171.3413972. [Google Scholar] [CrossRef]
75. P. Korshunov and T. Ebrahimi, “Using face morphing to protect privacy,” in 2013 10th IEEE Int. Conf. Adv. Video Signal Based Surveill. (AVSS 2013), Krakow, Poland, Aug. 27–30, 2013, pp. 208–213. doi: 10.1109/AVSS.2013.6636641. [Google Scholar] [CrossRef]
76. M. Yang, N. Bourbakis, and S. Li, “Data-image-video encryption,” IEEE Potentials, vol. 23, no. 3, pp. 28–34, 2004. doi: 10.1109/MP.2004.1341784. [Google Scholar] [CrossRef]
77. S. A. Khan et al., “Visual user-generated content verification in journalism: An overview,” IEEE Access, vol. 11, pp. 6748–6769, 2023. doi: 10.1109/ACCESS.2023.3236993. [Google Scholar] [CrossRef]
78. F. Dufaux and T. Ebrahimi, “H.264/AVC video scrambling for privacy protection,” in 2008 15th IEEE Int. Conf. Image Process. (ICIP), San Diego, CA, USA, Oct. 12–15, 2008, pp. 1688–1691. doi: 10.1109/ICIP.2008.4712098. [Google Scholar] [CrossRef]
79. K. Martin and K. N. Plataniotis, “Privacy protected surveillance using secure visual object coding,” IEEE Trans. Circuits Syst. Video Technol., vol. 18, no. 8, pp. 1152–1162, 2008. doi: 10.1109/TCSVT.2008.927110. [Google Scholar] [CrossRef]
80. A. Hafsa, M. Fradi, A. Sghaier, J. Malek, and M. Machhout, “Real-time video security system using chaos- improved advanced encryption standard (IAES),” Multimed. Tools Appl., vol. 81, no. 2, pp. 2275–2298, 2022. doi: 10.1007/s11042-021-11668-4. [Google Scholar] [CrossRef]
81. Y. Fouzar, A. Lakhssassi, S. Member, and M. Ramakrishna, “A novel hybrid multikey cryptography technique for video communication,” IEEE Access, vol. 11, pp. 15693–15700, 2023, Art. no. 3242616. doi: 10.1109/ACCESS.2023.3242616. [Google Scholar] [CrossRef]
82. B. A. Buhari, A. A. Obiniyi, K. Sunday, and S. Shehu, “Performance evaluation of symmetric data encryption algorithms: AES and Blowfish,” Saudi J. Eng. Technol., vol. 4, no. 10, pp. 407–414, 2019. doi: 10.36348/SJEAT.2019.v04i10.002. [Google Scholar] [CrossRef]
83. A. E. Adeniyi, S. Misra, E. Daniel, and A. Bokolo, “Computational complexity of modified blowfish cryptographic algorithm on video data,” Algorithms, vol. 15, no. 10, 2022, Art. no. 373. doi: 10.3390/a15100373. [Google Scholar] [CrossRef]
84. S. K. Salim, M. M. Msallam, and H. I. Olewi, “Hide text in an image using Blowfish algorithm and development of least significant bit technique,” Indones. J. Electr. Eng. Comput. Sci., vol. 29, no. 1, pp. 339–347, 2023. doi: 10.11591/ijeecs.v29.i1. [Google Scholar] [CrossRef]
85. M. K. Hussein and H. Amintoosi, “Protection of images by combination of vernam stream cipher, AES and LSB steganography in a video clip,” Bull. Electr. Eng. Inform., vol. 12, no. 3, pp. 1578–1585, 2023. doi: 10.11591/eei.v12i3.4039. [Google Scholar] [CrossRef]
86. A. A. Ahmed, S. J. Malebary, W. Ali, and A. A. Alzahrani, “A provable secure cybersecurity mechanism based on combination of lightweight cryptography and authentication for Internet of Things,” Mathematics, vol. 11, no. 1, 2023, Art. no. 220. doi: 10.3390/math11010220. [Google Scholar] [CrossRef]
87. M. Dua, D. Makhija, P. Y. L. Manasa, and P. Mishra, “3D chaotic map-cosine transformation based approach to video encryption and decryption,” Open Comput. Sci., vol. 12, no. 1, pp. 37–56, 2022. doi: 10.1515/comp-2020-0225. [Google Scholar] [CrossRef]
88. J. Sethi, J. Bhaumik, and A. S. Chowdhury, “Joint video compression and encryption using parallel compressive sensing and improved chaotic maps,” Digit. Signal Process., vol. 130, 2022, Art no. 103746. doi: 10.1016/j.dsp.2022.103746. [Google Scholar] [CrossRef]
89. M. A. Hadjadj, S. Sadoudi, M. S. Azzaz, H. Bendecheche, and R. Kaibou, “A new hardware architecture of lightweight and efficient real-time video chaos-based encryption algorithm,” J. Real Time Image Process, vol. 19, no. 6, pp. 1049–1062, 2022. doi: 10.1007/s11554-022-01244-w. [Google Scholar] [CrossRef]
90. S. Qiu, Y. Cui, and X. Meng, “A data encryption and fast transmission algorithm based on surveillance video,” Wirel Commun. Mob. Comput., vol. 2020, no. 1, 2020, Art. no. 8842412. doi: 10.1155/2020/8842412. [Google Scholar] [CrossRef]
91. C. H. Lin, G. H. Hu, J. S. Chen, J. J. Yan, and K. H. Tang, “Novel design of cryptosystems for video/audio streaming via dynamic synchronized chaos-based random keys,” Multimed. Syst., vol. 28, no. 5, pp. 1793–1808, 2022. doi: 10.1007/s00530-022-00950-6. [Google Scholar] [PubMed] [CrossRef]
92. P. Chen, Z. Zhang, Y. Lei, K. Niu, and X. Yang, “A multi-domain embedding framework for robust reversible data hiding scheme in encrypted videos,” Electronics, vol. 11, no. 16, pp. 1–21, 2022. doi: 10.3390/electronics11010001. [Google Scholar] [CrossRef]
93. B. M. El Den, W. A. Raslan, and A. A. Abdullah, “Even symmetric chaotic and skewed maps as a technique in video encryption,” EURASIP J. Adv. Signal Process, vol. 4, pp. 1–22, 2023. doi: 10.1186/s13634-023-01003-4. [Google Scholar] [CrossRef]
94. M. Es-Sabry et al., “An efficient 32-bit color image encryption technique using multiple chaotic maps and advanced ciphers,” Egypt. Inform. J., vol. 25, 2024, Art. no. 100449. doi: 10.1016/j.eij.2024.100449. [Google Scholar] [CrossRef]
95. M. Vijayakumar and A. Ahilan, “An optimized chaotic S-box for real-time image encryption scheme based on 4-dimensional memristive hyperchaotic map,” Ain Shams Eng. J., vol. 15, no. 4, 2024, Art. no. 102620. doi: 10.1016/j.asej.2023.102620. [Google Scholar] [CrossRef]
96. H. N. Noura, O. Salman, R. Couturier, and A. Chehab, “LoRCA: Lightweight round block and stream cipher algorithms for IoV systems,” Veh. Commun., vol. 34, 2022, Art. no. 100416. doi: 10.1016/j.vehcom.2021.100416. [Google Scholar] [CrossRef]
97. D. E. Mfungo, X. Fu, X. Wang, and Y. Xian, “Enhancing image encryption with the Kronecker xor product, the hill cipher, and the sigmoid logistic map,” Appl. Sci., vol. 13, no. 6, 2023, Art. no. 4034. doi: 10.3390/app13064034. [Google Scholar] [CrossRef]
98. R. A. Shah, M. N. Asghar, S. Abdullah, N. Kanwal, and M. Fleury, “SlEPX: An efficient lightweight cipher for visual protection of scalable HEVC extension,” IEEE Access, vol. 8, pp. 187784–187807, 2020. doi: 10.1109/ACCESS.2020.3030608. [Google Scholar] [CrossRef]
99. M. A. Saleh, N. M. Tahir, and H. Hashim, “Moving objects encryption of high efficiency video coding (HEVC) using AES algorithm,” J. Telecommun., Electron. Comput. Eng., vol. 8, no. 3, pp. 31–36, 2016. [Google Scholar]
100. O. Benrhouma, A. B. Alkhodre, A. Alzahrani, A. Namoun, and W. A. Bhat, “Using singular value decomposition and chaotic maps for selective encryption of video feeds in smart traffic management,” Appl. Sci. (Switzerland), vol. 12, no. 8, 2022, Art. no. 3917. doi: 10.3390/app12083917. [Google Scholar] [CrossRef]
101. T. Shanableh, “HEVC video encryption with high capacity message embedding by altering picture reference indices and motion vectors,” IEEE Access, vol. 10, pp. 22320–22329, 2022. doi: 10.1109/ACCESS.2022.3152548. [Google Scholar] [CrossRef]
102. C. Chen, X. Wang, G. Liu, and G. Huang, “A robust selective encryption scheme for H.265/HEVC video,” IEEE Access, vol. 11, pp. 17252–17264, 2023. doi: 10.1109/ACCESS.2022.3210132. [Google Scholar] [CrossRef]
103. F. Peng, X. Gong, and M. Long, “A selective encryption scheme for protecting H.264/AVC video in multimedia social network,” Multimed. Tools Appl., vol. 76, no. 3, pp. 3235–3253, 2017. doi: 10.1007/s11042-016-3710-x. [Google Scholar] [CrossRef]
104. A. I. Sallam, E. S. M. El-Rabaie, and O. S. Faragallah, “Efficient HEVC selective stream encryption using chaotic logistic map,” Multimed. Syst., vol. 24, no. 4, pp. 419–437, 2018. doi: 10.1007/s00530-017-0568-3. [Google Scholar] [CrossRef]
105. A. Shifa, M. N. Asghar, S. Noor, N. Gohar, and M. Fleury, “Lightweight cipher for H.264 videos in the internet of multimedia things with encryption space ratio diagnostics,” Sensors, vol. 19, no. 5, 2019, Art. no. 1228. doi: 10.3390/s19051228. [Google Scholar] [PubMed] [CrossRef]
106. J. Yun and M. Kim, “JLVEA: Lightweight real-time video stream encryption algorithm for internet of things,” Sensors, vol. 20, no. 13, pp. 1–14, 2020. doi: 10.3390/s20133627. [Google Scholar] [PubMed] [CrossRef]
107. A. Fitwi, Y. Chen, and S. Zhu, “Lightweight frame scrambling mechanisms for end-to-end privacy in edge smart surveillance,” IET Smart Cities, vol. 1049, pp. 17–35, 2021. doi: 10.1049/smc2.12019. [Google Scholar] [CrossRef]
108. A. Fitwi, Y. Chen, and S. Zhu, “Enforcing privacy preservation on edge cameras using lightweight video frame scrambling,” IEEE Trans. Serv. Comput., vol. 28, pp. 1–12, 2021. doi: 10.1109/TSC.2021.3135352. [Google Scholar] [CrossRef]
109. M. Farajallah, G. Gautier, W. Hamidouche, O. Déforges, and S. E. L. Assad, “Selective encryption of the versatile video coding standard,” IEEE Access, vol. 10, pp. 21821–21835, 2022. doi: 10.1109/ACCESS.2022.3149599. [Google Scholar] [CrossRef]
110. P. Kanani et al., “Lightweight multi-level authentication scheme for secured data transmission in IoT-Fog context,” J. Comb. Optim., vol. 45, no. 2, 2023, Art. no. 46. doi: 10.1007/s10878-023-00987-x. [Google Scholar] [CrossRef]
111. W. El Hadj Youssef, A. Abdelli, F. Dridi, R. Brahim, and M. Machhout, “An efficient lightweight cryptographic instructions set extension for IoT device security,” Secur. Commun. Netw., vol. 2022, pp. 1–17, 2022. doi: 10.1155/2022/9709601. [Google Scholar] [CrossRef]
112. G. M. Karthik, “Deep intelligent blockchain technology for securing IoT-based healthcare multimedia data,” Wirel. Netw., vol. 29, no. 6, pp. 2481–2493, 2023. doi: 10.1007/s11276-023-03333-5. [Google Scholar] [CrossRef]
113. B. Al-shargabi and A. Dar Assi, “A modified lightweight DNA-based cryptography method for internet of things devices,” Expert. Syst., vol. 40, no. 6, pp. 1–12, 2023. doi: 10.1111/exsy.13270. [Google Scholar] [CrossRef]
114. E. Yaacoub, “Resource allocation functionality with cluster aggregation (RAFCA) for secure HST video transmission,” Multimed. Tools Appl., vol. 83, no. 3, pp. 7583–7607, 2024. doi: 10.1007/s11042-023-15957-y. [Google Scholar] [CrossRef]
115. D. Clemente-Lopez, J. J. De Rangel-Magdaleno, and J. M. Muñoz-Pacheco, “A lightweight chaos-based encryption scheme for IoT healthcare systems,” Internet of Things, vol. 25, 2024, Art. no. 101032. doi: 10.1016/j.iot.2023.101032. [Google Scholar] [CrossRef]
116. I. Aribilola, M. N. Asghar, N. Kanwal, M. Fleury, and B. Lee, “SecureCam: Selective detection and encryption enabled application for dynamic camera surveillance videos,” IEEE Trans. Consum. Electron., vol. 69, no. 2, pp. 156–169, 2023. doi: 10.1109/TCE.2022.3228679. [Google Scholar] [CrossRef]
117. F. Amjad, F. Khan, S. Tahir, T. Yaqoob, and H. Abbas, “ENCVIDC: An innovative approach for encoded video content classification,” Neural Comput. Appl., vol. 34, no. 21, pp. 18685–18702, 2022. doi: 10.1007/s00521-022-07480-2. [Google Scholar] [CrossRef]
118. Q. Geng, H. Yan, and X. Lu, “Optimization of a deep learning algorithm for security protection of big data from video images,” Comput Intell. Neurosci, vol. 2022, pp. 1–17, 2022. doi: 10.1155/2022/3394475. [Google Scholar] [PubMed] [CrossRef]
119. S. S. Ingaleshwar, D. Jayadevappa, and N. V. Dharwadkar, “Sine cosine bird swarm algorithm-based deep convolution neural network for reversible medical video watermarking,” Multimed. Tools Appl., vol. 82, pp. 36687–36712, 2023. doi: 10.1007/s11042-023-14495-x. [Google Scholar] [CrossRef]
120. M. Kaczyński and Z. Piotrowski, “High-quality video watermarking based on deep neural networks and adjustable subsquares properties algorithm,” Sensors, vol. 22, no. 14, 2022, Art. no. 5376. doi: 10.3390/s22145376. [Google Scholar] [PubMed] [CrossRef]
121. G. Bhoi, R. Bhavsar, P. Prajapati, and P. Shah, “A review of recent trends on DNA based cryptography,” in 2020 3rd Int. Conf. Intell. Sustainable Syst. (ICISS), Thoothukudi, India, Dec. 3–5, 2020, pp. 815–822. doi: 10.1109/ICISS49785.2020.9316013. [Google Scholar] [CrossRef]
122. J. Karmakar, A. Pathak, D. Nandi, and M. K. Mandal, “Sparse representation based compressive video encryption using hyper-chaos and DNA coding,” Digit. Signal Process., vol. 117, 2021, Art. no. 103143. doi: 10.1016/j.dsp.2021.103143. [Google Scholar] [CrossRef]
123. R. Ettiyan and V. Geetha, “A hybrid logistic DNA-based encryption system for securing the Internet of Things patient monitoring systems,” Healthcare Anal., vol. 3, 2023, Art. no. 100149. doi: 10.1016/j.health.2023.100149. [Google Scholar] [CrossRef]
124. W. Wen, R. Tu, and K. Wei, “Video frames encryption based on DNA sequences and chaos,” in Eleventh Int. Conf. Digit. Image Process. (ICDIP 2019), Guangzhou, China, SPIE, May 10–13, 2019, pp. 756–760. doi: 10.1117/12.2540057. [Google Scholar] [CrossRef]
125. E. Farri and P. Ayubi, “A robust digital video watermarking based on CT-SVD domain & chaotic DNA sequences for copyright protection,” J. Ambient Intell. Humaniz Comput., vol. 14, no. 10, pp. 13113–13137, 2022. doi: 10.1007/s12652-022-03771-7. [Google Scholar] [CrossRef]
126. F. Masood et al., “A novel privacy approach of digital aerial images based on mersenne twister method with DNA genetic encoding and chaos,” Remote Sens., vol. 12, no. 11, pp. 1–25, 2020. doi: 10.3390/rs12111893. [Google Scholar] [CrossRef]
127. SVG Staff, “Case Study: City of Westerville Uses Drones + LiveU for Situational Awareness,” Sports Video Group. Accessed: Jul. 27, 2024. [Online]. Available: https://www.sportsvideo.org/2023/11/16/case-study-city-of-westerville-uses-drones-liveu-for-situational-awareness/ [Google Scholar]
128. A. V. Savkin and H. Huang, “Multi-UAV navigation for optimized video surveillance of ground vehicles on uneven terrains,” IEEE Trans. Intell. Transp. Syst., vol. 24, no. 9, pp. 10238–10242, 2023. doi: 10.1109/TITS.2023.3270969. [Google Scholar] [CrossRef]
129. H. Benkraouda, E. Barka, and K. Shuaib, “Cyber-attacks on the data communication of monitoring critical infrastructure,” Comput. Sci. Inform. Technol. (CS&IT), pp. 83–93, 2018. doi: 10.5121/CSIT.2018.81708. [Google Scholar] [CrossRef]
130. A. Fotouhi et al., “Survey on UAV cellular communications: Practical aspects, standardization advancements, regulation, and security challenges,” IEEE Commun. Surv. Tutorials, vol. 21, no. 4, pp. 3417–3442, 2019. doi: 10.1109/COMST.2019.2906228. [Google Scholar] [CrossRef]
131. F. S. Alrayes et al., “Artificial Intelligence-based secure communication and classification for drone-enabled emergency monitoring systems,” Drones, vol. 6, no. 9, 2022, Art. no. 222. doi: 10.3390/drones6090222. [Google Scholar] [CrossRef]
132. W. Xiao, M. Li, B. Alzahrani, R. Alotaibi, A. Barnawi and Q. Ai, “A blockchain-based secure crowd monitoring system using UAV swarm,” IEEE Netw., vol. 35, no. 1, pp. 108–115, 2021. doi: 10.1109/MNET.011.2000210. [Google Scholar] [CrossRef]
133. B. Bera, A. K. Das, S. Garg, M. J. Piran, and M. S. Hossain, “Access control protocol for battlefield surveillance in drone-assisted IoT environment,” IEEE Internet Things, vol. 9, no. 4, pp. 2708–2721, 2022. doi: 10.1109/JIOT.2020.3049003. [Google Scholar] [CrossRef]
134. P. Teixidó et al., “Secured perimeter with electromagnetic detection and tracking with drone embedded and static cameras,” Sensors, vol. 21, no. 21, 2021, Art. no. 7379. doi: 10.3390/s21217379. [Google Scholar] [PubMed] [CrossRef]
135. C. Marcolla et al., “Survey on fully homomorphic encryption, theory, and applications,” Proc. IEEE, vol. 110, no. 10, pp. 1572–1609, 2022. doi: 10.1109/JPROC.2022.3205665. [Google Scholar] [CrossRef]
136. N. Geetha and K. Mahesh, “An efficient enhanced full homomorphic encryption for securing video in cloud environment,” Wirel. Pers. Commun., vol. 123, no. 2, pp. 1553–1571, 2022. doi: 10.1007/s11277-021-09200-w. [Google Scholar] [CrossRef]
137. U. Goswami, K. Wang, G. Nguyen, and B. Lagesse, “Privacy-preserving mobile video sharing using fully homomorphic encryption,” in 2020 IEEE Int. Conf. Pervasive Comput. Commun. Workshops (PerCom Workshops), Austin, TX, USA, Mar. 23–27, 2020, pp. 1–3. doi: 10.1109/PerComWorkshops48775.2020.9156217. [Google Scholar] [CrossRef]
138. B. Lagesse, G. Nguyen, U. Goswami, and K. Wu, “You had to be there: Private video sharing for mobile phones using fully homomorphic encryption,” in 2021 IEEE Int. Conf. Pervasive Comput. Commun. Workshops Other Affiliated Events (PerCom Workshops), Kassel, Germany, Mar. 22–26, 2021, pp. 730–735. doi: 10.1109/PerComWorkshops51409.2021.9431029. [Google Scholar] [CrossRef]
139. H. Yan, M. Chen, L. Hu, and C. Jia, “Secure video retrieval using image query on an untrusted cloud,” Appl. Soft Comput., vol. 97, 2020, Art. no. 106782. doi: 10.1016/j.asoc.2020.106782. [Google Scholar] [CrossRef]
140. Z. Li, Y. Sang, X. Deng, and H. Tian, “Lightweight and efficient privacy-preserving multimodal representation inference via fully homomorphic encryption,” in Intell. Inform. Database Syst.: 15th Asian Conf. (ACIIDS 2023), Phuket, Thailand, Jul. 24–26, 2023, pp. 307–321. doi: 10.1007/978-981-99-5834-4_25. [Google Scholar] [CrossRef]
141. F. Farokhi, I. Shames, and N. Batterham, “Secure and private control using semi-homomorphic encryption,” Control Eng. Pract., vol. 67, pp. 13–20, Oct. 2017. doi: 10.1016/j.conengprac.2017.07.004. [Google Scholar] [CrossRef]
142. S. Priya, R. Varatharajan, G. Manogaran, R. Sundarasekar, and P. M. Kumar, “Paillier homomorphic cryptosystem with poker shuffling transformation based water marking method for the secured transmission of digital medical images,” Pers. Ubiquitous Comput., vol. 22, no. 5–6, pp. 1141–1151, 2018. doi: 10.1007/s00779-018-1131-8. [Google Scholar] [CrossRef]
143. Z. Azad, G. Yang, R. Agrawal, D. Petrisko, M. Taylor and A. Joshi, “RACE: RISC-V SoC for En/decryption acceleration on the edge for homomorphic computation,” in Proc. Int. Symp. Low Power Electron. Design (ISLPED’22), Boston, MA, USA, Aug. 1–3, 2022. doi: 10.1145/3531437.3539725. [Google Scholar] [CrossRef]
144. M. N. Sharath, T. M. Rajesh, and M. Patil, “Design of optimal metaheuristics based pixel selection with homomorphic encryption technique for video steganography,” Int. J. Inform. Technol., vol. 14, no. 5, pp. 2265–2274, 2022. doi: 10.1007/s41870-022-01005-9. [Google Scholar] [CrossRef]
145. F. Hartung and M. Kutter, “Multimedia watermarking techniques,” Proc. IEEE, vol. 87, no. 7, pp. 1079–1107, 1999. doi: 10.1109/5.771066. [Google Scholar] [CrossRef]
146. Z. Yuan, Q. Su, D. Liu, and X. Zhang, “A blind image watermarking scheme combining spatial domain and frequency domain,” Vis. Comput., vol. 37, no. 7, pp. 1867–1881, 2020. doi: 10.1007/s00371-020-01945-y. [Google Scholar] [CrossRef]
147. V. Sharma, M. Gangarde, and S. Oza, “A spatial domain based secure and robust video watermarking technique using modified LSB and secret image sharing processing,” ICTACT J. Image. Video Process., vol. 1, no. 10, pp. 2061–2070, 2019. doi: 10.21917/ijivp.2019.0293. [Google Scholar] [CrossRef]
148. R. Singh, A. Ashok, and M. Saraswat, “Robust video watermarking in frequency domain for copyright protection,” in Proc. 2021 Thirteenth Int. Conf. Contemp. Comput., Noida, India, Aug. 5–7, 2021, pp. 174–178. doi: 10.1145/3474124.3474148. [Google Scholar] [CrossRef]
149. K. Abdelhedi, F. Chaabane, W. Puech, and C. B. Amar, “A novel robust spread spectrum watermarking scheme for 3D video traitor tracing,” IEEE Access, vol. 11, pp. 93487–93499, 2023. doi: 10.1109/ACCESS.2023.3308494. [Google Scholar] [CrossRef]
150. G. Dhevanandhini and G. Yamuna, “An effective and secure video watermarking using hybrid technique,” Multimed. Syst., vol. 27, no. 5, pp. 953–967, 2021. doi: 10.1007/s00530-021-00765-x. [Google Scholar] [CrossRef]
151. O. S. Faragallah et al., “Cybersecurity framework of hybrid watermarking and selective encryption for secure HEVC communication,” J. Ambient Intell. Humaniz. Comput., vol. 13, no. 2, pp. 1215–1239, 2022. doi: 10.1007/s12652-020-02832-z. [Google Scholar] [CrossRef]
152. W. El-Shafai et al., “Efficient framework for video communication in IoT applications,” Wirel. Pers. Commun., vol. 129, no. 1, pp. 1–35, 2023. doi: 10.1007/s11277-022-09491-7. [Google Scholar] [CrossRef]
153. Z. Cao and L. Wang, “A secure video watermarking technique based on hyperchaotic Lorentz system,” Multimed. Tools Appl., vol. 78, no. 18, pp. 26089–26109, 2019. doi: 10.1007/s11042-019-07809-5. [Google Scholar] [CrossRef]
154. L. Jiang, “The identical operands commutative encryption and watermarking based on homomorphism,” Multimed. Tools Appl., vol. 77, no. 23, pp. 30575–30594, 2018. doi: 10.1007/s11042-018-6142-y. [Google Scholar] [CrossRef]
155. S. Zhang, X. Guo, X. Xu, and L. Li, “A video watermark algorithm based on tensor feature map,” Multimed. Tools Appl., vol. 82, no. 13, pp. 19557–19575, 2023. doi: 10.1007/s11042-022-14299-5. [Google Scholar] [CrossRef]
156. L. Tian, H. Dai, and C. Li, “A semi-fragile video watermarking algorithm based on chromatic residual DCT,” Multimed. Tools Appl., vol. 79, no. 3–4, pp. 1759–1779, 2020. doi: 10.1007/s11042-019-08256-y. [Google Scholar] [CrossRef]
157. S. Zhang, H. Li, L. Li, J. Lu, and C. C. Chang, “A video watermarking algorithm based on time factor matrix,” Multimed. Tools Appl., vol. 82, no. 5, pp. 7509–7527, 2023. doi: 10.1007/s11042-022-13609-1. [Google Scholar] [CrossRef]
158. M. Du, T. Luo, H. Xu, Y. Song, C. Wang and L. Li, “Robust HDR video watermarking method based on the HVS model and T-QR,” Multimed. Tools Appl., vol. 81, no. 23, pp. 33375–33395, 2022. doi: 10.1007/s11042-022-13145-y. [Google Scholar] [CrossRef]
159. D. Fan, X. Zhang, W. Kang, H. Zhao, and Y. Lv, “Video watermarking algorithm based on NSCT, Pseudo 3D-DCT and NMF,” Sensors, vol. 22, no. 13, 2022, Art. no. 4752. doi: 10.3390/s22134752. [Google Scholar] [PubMed] [CrossRef]
160. D. Kim, S. Y. Ihm, and Y. Son, “Two-level blockchain system for digital crime evidence management,” Sensors, vol. 21, no. 9, 2021, Art. no. 3051. doi: 10.3390/s21093051. [Google Scholar] [PubMed] [CrossRef]
161. P. W. Khan, Y. C. Byun, and N. Park, “A data verification system for CCTV surveillance cameras using blockchain technology in smart cities,” Electronics, vol. 9, no. 3, 2020, Art. no. 484. doi: 10.3390/electronics9030484. [Google Scholar] [CrossRef]
162. M. Dave, V. Rastogi, M. Miglani, P. Saharan, and N. Goyal, “Smart fog-based video surveillance with privacy preservation based on blockchain,” Wirel. Pers. Commun., vol. 124, no. 2, pp. 1677–1694, 2022. doi: 10.1007/s11277-021-09426-8. [Google Scholar] [CrossRef]
163. S. B. Lee, A. Park, and J. Song, “Blockchain technology and application,” J. Korea Soc. Comput. Inform., vol. 26, no. 2, pp. 89–97, 2021. doi: 10.9708/JKSCI.2021.26.02.089. [Google Scholar] [CrossRef]
164. J. Liu, K. Fan, H. Li, and Y. Yang, “A blockchain-based privacy preservation scheme in multimedia network,” Multimed. Tools Appl., vol. 80, no. 20, pp. 30691–30705, 2021. doi: 10.1007/s11042-021-10513-y. [Google Scholar] [CrossRef]
165. R. F. Ciriello, A. C. G. Torbensen, M. R. P. Hansen, and C. Müller-Bloch, “Blockchain-based digital rights management systems: Design principles for the music industry,” Electronic. Mark., vol. 33, no. 1, pp. 1–21, 2023. doi: 10.1007/s12525-023-00628-5. [Google Scholar] [CrossRef]
166. R. A. Shah, S. A. Nawaz, Q. Shaheen, and A. Asghar, “Collaborative blockchain-based crypto-efficient scheme for protecting visual contents,” J. Comput. Biomed. Inform., vol. 6, no. 3, pp. 1–11, 2024. [Google Scholar]
167. S. R. Subramanya and B. K. Yi, “Digital rights management,” IEEE Potentials, vol. 25, no. 2, pp. 31–34, 2006. doi: 10.1109/MP.2006.1649008. [Google Scholar] [CrossRef]
168. P. Ayubi, M. J. Barani, M. Y. Valandar, and B. Y. Irani, “A new chaotic complex map for robust video watermarking,” Artif. Intell. Rev., vol. 54, no. 2, pp. 1–30, 2021. doi: 10.1007/s10462-020-09877-8. [Google Scholar] [CrossRef]
169. S. P. Mohanty, “A secure digital camera architecture for integrated real-time digital rights management,” J. Syst. Archit., vol. 55, no. 10–12, pp. 468–480, 2017. doi: 10.1016/j.sysarc.2009.09.005. [Google Scholar] [CrossRef]
170. Z. Zhang, Z. Wang, and D. Niu, “A novel approach to rights sharing-enabling digital rights management for mobile multimedia,” Multimed. Tools Appl., vol. 74, no. 16, pp. 6255–6271, 2014. doi: 10.1007/s11042-014-2135-7. [Google Scholar] [CrossRef]
171. M. T. Chen, Y. Y. Chang, and T. J. Wu, “Digital copyright management mechanism based on dynamic encryption for multiplatform browsers,” Int. J. Semant. Web Inf. Syst., vol. 20, no. 1, pp. 1–22, 2023. doi: 10.4018/IJSWIS.334591. [Google Scholar] [CrossRef]
172. Y. Fan, G. Wen, F. Xiao, S. Qiu, and D. Li, “Detecting anomalies in videos using perception generative adversarial network,” Circ. Syst. Signal Process, vol. 41, no. 2, pp. 994–1018, 2022. doi: 10.1007/S00034-021-01820-8. [Google Scholar] [CrossRef]
173. D. T. Tran, D. T. Phung, D. M. Duong, K. Inoue, J. H. Lee and A. Q. Nguyen, “Privacy-preserving face and hair swapping in real-time with a GAN-generated face image,” IEEE Access, 2024. doi: 10.1109/ACCESS.2024.3420452. [Google Scholar] [CrossRef]
174. Y. Wu, F. Yang, Y. Xu, and H. Ling, “Privacy-protective-GAN for privacy preserving face de-identification,” J. Comput. Sci. Technol., vol. 34, no. 1, pp. 47–60, 2019. doi: 10.1007/S11390-019-1898-8. [Google Scholar] [CrossRef]
175. S. Singh, R. Sharma, and A. F. Smeaton, “Using GANs to synthesise minimum training data for deepfake generation,” in Proc. CEUR Workshop, 2020, vol. 2771, pp. 193–204. Accessed: Jul. 28, 2024. [Online]. Available:https://arxiv.org/abs/2011.05421v1 [Google Scholar]
176. D. Guera and E. J. Delp, “Deepfake video detection using recurrent neural networks,” in 2018 15th IEEE Int. Conf. Adv. Video Signal Based Surveill. (AVSS), Auckland, New Zealand, Nov. 27–30, 2018, pp. 1–6. doi: 10.1109/AVSS.2018.8639163. [Google Scholar] [CrossRef]
177. S. A. Abaas and A. K. Shibeeb, “A new approach for video encryption based on modified AES algorithm,” IOSR J. Comput. Eng. (IOSR-JCE), vol. 17, no. 3, pp. 44–51, 2015. doi: 10.9790/0661-17364451. [Google Scholar] [CrossRef]
178. V. A. Memos and K. E. Psannis, “Encryption algorithm for efficient transmission of HEVC media,” J. Real Time Image Process, vol. 12, no. 2, pp. 473–482, 2016. doi: 10.1007/s11554-015-0509-3. [Google Scholar] [CrossRef]
179. X. Zhou, H. Wang, K. Li, L. Tang, N. Mo and Y. Jin, “A video streaming encryption method and experimental system based on reconfigurable quaternary logic operators,” IEEE Access, vol. 12, pp. 25034–25051, 2024. doi: 10.1109/ACCESS.2024.3365523. [Google Scholar] [CrossRef]
180. X. Li, H. Yu, H. Zhang, X. Jin, H. Sun and J. Liu, “Video encryption based on hyperchaotic system,” Multimed. Tools Appl., vol. 79, pp. 23995–24011, 2020. doi: 10.1007/s11042-020-09200-1. [Google Scholar] [CrossRef]
181. Y. Li, Z. Li, M. Ma, and M. Wang, “Generation of grid multi-wing chaotic attractors and its application in video secure communication system,” Multimed. Tools Appl., vol. 79, pp. 29161–29177, 2020. doi: 10.1007/s11042-020-09448-7. [Google Scholar] [CrossRef]
182. O. El Ogri, H. Karmouni, M. Sayyouri, and H. Qjidaa, “A new image/video encryption scheme based on fractional discrete Tchebichef transform and singular value decomposition,” Multimed. Tools Appl., vol. 82, no. 22, pp. 33465–33497, 2023. doi: 10.1007/s11042-023-14573-0. [Google Scholar] [CrossRef]
183. D. Dhingra and M. Dua, “A chaos-based novel approach to video encryption using dynamic S-box,” Multimed. Tools Appl., vol. 83, no. 1, pp. 1693–1723, 2024. doi: 10.1007/s11042-023-15593-6. [Google Scholar] [CrossRef]
184. H. Li, Z. Gu, L. Deng, Y. Han, C. Yang and Z. Tian, “A fine-grained video encryption service based on the cloud-fog-local architecture for public and private videos,” Sensors, vol. 19, no. 24, pp. 1–21, 2019. doi: 10.3390/s19245366. [Google Scholar] [PubMed] [CrossRef]
185. A. Shifa, M. B. Imtiaz, M. N. Asghar, and M. Fleury, “Skin detection and lightweight encryption for privacy protection in real-time surveillance applications,” Image Vis. Comput., vol. 94, 2020, Art. no. 103859. doi: 10.1016/j.imavis.2019.103859. [Google Scholar] [CrossRef]
186. A. Shifa et al., “MuLViS: Multi-level encryption based security system for surveillance videos,” IEEE Access, vol. 8, pp. 177131–177155, 2020. doi: 10.1109/ACCESS.2020.3024926. [Google Scholar] [CrossRef]
187. I. Aribilola, B. Lee, and M. N. Asghar, “Pixel tampering detection in encrypted surveillance videos on resource-constrained devices,” Internet of Things, vol. 25, 2024, Art. no. 101058. doi: 10.1016/j.iot.2023.101058. [Google Scholar] [CrossRef]
188. Z. Shahid and W. Puech, “Visual protection of HEVC video by selective encryption of CABAC binstrings,” IEEE Trans. Multimed., vol. 16, no. 1, pp. 24–36, 2015. doi: 10.1109/TMM.2013.2281029. [Google Scholar] [CrossRef]
189. R. Hamza, A. Hassan, T. Huang, L. Ke, and H. Yan, “An efficient cryptosystem for video surveillance in the internet of things environment,” Complexity, 2019. doi: 10.1155/2019/1625678. [Google Scholar] [CrossRef]
190. V. Baboolal, K. Akkaya, N. Saputro, and K. Rabieh, “Preserving privacy of drone videos using proxy re-encryption technique,” in Proc. 12th Conf. Secur. Priv. Wireless Mobile Netw. (WiSec ’19), Miami, FL, USA, May 15–17, 2019, pp. 336–337. doi: 10.1145/3317549.3326319. [Google Scholar] [CrossRef]
191. H. M. Ismael, Z. Tariq Mustafa Al-Ta, and A. Emails Mordasshani, “Authentication and encryption drone communication by using HIGHT lightweight algorithm,” Turkish J. Comput. Math. Educ.(TURCOMAT), vol. 12, no. 11, pp. 5891–5908, 2021. doi: 10.17762/TURCOMAT.V12I11.6875. [Google Scholar] [CrossRef]
192. S. Silalahi, T. Ahmad, and H. Studiawan, “Transformer-based named entity recognition on drone flight logs to support forensic investigation,” IEEE Access, vol. 11, pp. 3257–3274, 2023. doi: 10.1109/ACCESS.2023.3234605. [Google Scholar] [CrossRef]
193. C. Sharma, B. Amandeep, R. Sobti, T. K. Lohani, and M. Shabaz, “A secured frame selection based video watermarking technique to address quality loss of data: Combining graph based transform, singular valued decomposition, and hyperchaotic encryption,” Secur. Commun. Netw., vol. 2021, pp. 1–19, 2021. doi: 10.1155/2021/5536170. [Google Scholar] [CrossRef]
194. R. Singh, H. Mittal, and R. Pal, “Optimal keyframe selection-based lossless video-watermarking technique using IGSA in LWT domain for copyright protection,” Complex Intell. Syst., vol. 8, no. 2, pp. 1047–1070, 2022. doi: 10.1007/s40747-021-00569-6. [Google Scholar] [CrossRef]
195. S. Chikkerur, V. Sundaram, M. Reisslein, and L. J. Karam, “Objective video quality assessment methods: A classification, review, and performance comparison,” IEEE Trans. Broadcast., vol. 57, no. 2, pp. 165–182, 2011. doi: 10.1109/TBC.2011.2104671. [Google Scholar] [CrossRef]
196. W. El-Shafai, I. M. Almomani, and A. Alkhayer, “Optical bit-plane-based 3D-JST cryptography algorithm with cascaded 2D-FrFT encryption for efficient and secure HEVC communication,” IEEE Access, vol. 9, pp. 35004–35026, 2021. doi: 10.1109/ACCESS.2021.3062403. [Google Scholar] [CrossRef]
197. D. Xu, “Commutative encryption and data hiding in HEVC video compression,” IEEE Access, vol. 7, pp. 66028–66041, 2019. doi: 10.1109/ACCESS.2019.2916484. [Google Scholar] [CrossRef]
198. N. Dolati, A. Beheshti, and H. Azadegan, “A selective encryption for H.264/AVC videos based on scrambling,” Multimed. Tools Appl., vol. 80, no. 2, pp. 2319–2338, 2021. doi: 10.1007/s11042-020-09654-3. [Google Scholar] [CrossRef]
199. W. El-Shafai, A. K. Mesrega, H. E. Ahmed, N. A. El-Bahnasawy, and F. E. Abd El-Samie, “An efficient multimedia compression-encryption scheme using latin squares for securing Internet-of-things networks,” J. Inf. Secur. Appl., vol. 64, 2022, Art. no. 103039. doi: 10.1016/j.jisa.2021.103039. [Google Scholar] [CrossRef]
200. K. M. Hosny, M. A. Zaki, N. A. Lashin, and H. M. Hamza, “Fast colored video encryption using block scrambling and multi-key generation,” Vis. Comput., vol. 39, no. 12, pp. 6041–6072, 2023. doi: 10.1007/s00371-022-02711-y. [Google Scholar] [CrossRef]
201. W. El-Shafai, S. El-Rabaie, M. M. El-Halawany, and F. E. A. El-Samie, “Security of 3D-HEVC transmission based on fusion and watermarking techniques,” Multimed. Tools Appl., vol. 78, no. 19, pp. 27211–27244, 2019. doi: 10.1007/s11042-019-7448-0. [Google Scholar] [CrossRef]
202. H. Wen, Y. Lin, Z. Xie, and T. Liu, “Chaos-based block permutation and dynamic sequence multiplexing for video encryption,” Sci. Rep., vol. 13, no. 1, pp. 1–22, 2023. doi: 10.1038/s41598-023-41082-9. [Google Scholar] [PubMed] [CrossRef]
203. S. Saha and A. K. Chattopadhyay, “Secret image sharing schemes: A comprehensive survey,” IEEE Access, vol. 11, pp. 98333–98361, 2023. doi: 10.1109/ACCESS.2023.3304055. [Google Scholar] [CrossRef]
204. S. Y. Lo and V. M. Patel, “Defending against multiple and unforeseen adversarial videos,” IEEE Trans. Image Process., vol. 31, pp. 962–973, 2022. doi: 10.1109/TIP.2021.3137648. [Google Scholar] [PubMed] [CrossRef]
205. W. Shi, J. Cao, Q. Zhang, Y. Li, and L. Xu, “Edge computing: Vision and challenges,” IEEE Internet Things J., vol. 3, no. 5, pp. 637–646, 2016. doi: 10.1109/JIOT.2016.2579198. [Google Scholar] [CrossRef]
206. A. Fitwi and Y. Chen, “PriSE: Slenderized privacy-preserving surveillance as an edge service,” in 2020 IEEE 6th Int. Conf. Collab. Internet Comput. (CIC), Atlanta, GA, USA, Dec. 1–3, 2020, pp. 125–134. doi: 10.1109/CIC50333.2020.00024. [Google Scholar] [CrossRef]
207. A. B. Arrieta et al., “Explainable Artificial Intelligence (XAI): Concepts, taxonomies, opportunities and challenges toward responsible AI,” Inf. Fusion, vol. 58, pp. 82–115, 2020. doi: 10.1016/j.inffus.2019.12.012. [Google Scholar] [CrossRef]
208. L. Vigano and D. Magazzeni, “Explainable security,” in 2020 IEEE Eur. Symp. Secur. Priv. Workshops (EuroS&PW), Genoa, Italy, Sep. 7–11, 2020, pp. 293–300. doi: 10.1109/EuroSPW51379.2020.00045. [Google Scholar] [CrossRef]
209. X. Lai and P. P. Rau, “Computers in human behavior has facial recognition technology been misused? A public perception model of facial recognition scenarios,” Comput. Human Behav., vol. 124, 2021, Art. no. 106894. doi: 10.1016/j.chb.2021.106894. [Google Scholar] [CrossRef]
210. M. Strand, “A status update on quantum safe cryptography,” in 2021 Int. Conf. Mil. Commun. Inform. Syst. (ICMCIS), The Hague, Netherlands, May 4–5, 2021, pp. 1–7. doi: 10.1109/ICMCIS52405.2021.9486413. [Google Scholar] [CrossRef]
211. J. Wang et al., “Quantum-safe cryptography: Crossroads of coding theory and cryptography,” Sci. China Inf. Sci., vol. 65, no. 1, pp. 1–21, 2022. doi: 10.1007/s11432-021-3354-7. [Google Scholar] [CrossRef]
212. D. Singh, S. O. Anusandhan, and S. Kumar, “Cyber-hygiene: The key concept for cyber security in cyberspace,” Test Eng. Manage., vol. 83, pp. 8145–8152, 2020. [Google Scholar]
213. A. A. Cain, M. E. Edwards, and J. D. Still, “An exploratory study of cyber hygiene behaviors and knowledge,” J. Inf. Secur. Appl., vol. 42, pp. 36–45, 2018. doi: 10.1016/j.jisa.2018.08.002. [Google Scholar] [CrossRef]
214. GDPR, “General Data Protection Regulation (GDPR)–official legal text,” Accessed: Apr. 20, 2024. [Online]. Available: https://gdpr-info.eu/ [Google Scholar]
215. EUAIA, “AI Act–Legal text,” Accessed: Jul. 14, 2024. [Online]. Available: https://ai-act-law.eu/ [Google Scholar]
216. CCPA, “California Consumer Privacy Act (CCPA),” Department of Justice. Accessed: Apr. 20, 2024. [Online]. Available: https://oag.ca.gov/privacy/ccpa [Google Scholar]
217. HIPAA, “Health insurance portability and accountability act of 1996,” Public Law 104–191, 1996. [Google Scholar]
218. ISO/IEC 27001 Standard, “ISO/IEC 27001: 2022—Information security management systems—Requirements,” ISO. Accessed: Jul. 14, 2024. [Online]. Available: https://www.iso.org/standard/27001 [Google Scholar]
Cite This Article
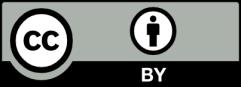
This work is licensed under a Creative Commons Attribution 4.0 International License , which permits unrestricted use, distribution, and reproduction in any medium, provided the original work is properly cited.