Open Access
ARTICLE
Heart-Net: A Multi-Modal Deep Learning Approach for Diagnosing Cardiovascular Diseases
1 Department of Computer Science and Information Technology, Applied College, Princess Nourah bint Abdulrahman University, Riyadh, 84428, Saudi Arabia
2 College of Engineering and Technology, American University of the Middle East, Egaila, 15453, Kuwait
3 Faculty of Computers Science, Misr International University, Cairo, 11800, Egypt
4 University Institute of Information Technology—PMAS Arid Agriculture University, Rawalpjndi, 46000, Pakistan
5 Department of Computer Science, International Islamic University, Islamabad, 04436, Pakistan
6 Jadara Research Center, Jadara University, Irbid, 21110, Jordan
7 Information System Department, Faculty of Computers and Artificial Intelligence, Benha University, Banha, 13511, Egypt
8 MEU Research Unit, Middle East University, Amman, 11831, Jordan
* Corresponding Author: Asif Nawaz. Email:
(This article belongs to the Special Issue: Deep Learning in Medical Imaging-Disease Segmentation and Classification)
Computers, Materials & Continua 2024, 80(3), 3967-3990. https://doi.org/10.32604/cmc.2024.054591
Received 02 June 2024; Accepted 17 July 2024; Issue published 12 September 2024
Abstract
Heart disease remains a leading cause of morbidity and mortality worldwide, highlighting the need for improved diagnostic methods. Traditional diagnostics face limitations such as reliance on single-modality data and vulnerability to apparatus faults, which can reduce accuracy, especially with poor-quality images. Additionally, these methods often require significant time and expertise, making them less accessible in resource-limited settings. Emerging technologies like artificial intelligence and machine learning offer promising solutions by integrating multi-modality data and enhancing diagnostic precision, ultimately improving patient outcomes and reducing healthcare costs. This study introduces Heart-Net, a multi-modal deep learning framework designed to enhance heart disease diagnosis by integrating data from Cardiac Magnetic Resonance Imaging (MRI) and Electrocardiogram (ECG). Heart-Net uses a 3D U-Net for MRI analysis and a Temporal Convolutional Graph Neural Network (TCGN) for ECG feature extraction, combining these through an attention mechanism to emphasize relevant features. Classification is performed using Optimized TCGN. This approach improves early detection, reduces diagnostic errors, and supports personalized risk assessments and continuous health monitoring. The proposed approach results show that Heart-Net significantly outperforms traditional single-modality models, achieving accuracies of 92.56% for Heartnet Dataset I (HNET-DSI), 93.45% for Heartnet Dataset II (HNET-DSII), and 91.89% for Heartnet Dataset III (HNET-DSIII), mitigating the impact of apparatus faults and image quality issues. These findings underscore the potential of Heart-Net to revolutionize heart disease diagnostics and improve clinical outcomes.Keywords
Cite This Article
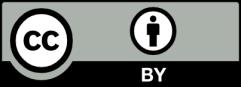
This work is licensed under a Creative Commons Attribution 4.0 International License , which permits unrestricted use, distribution, and reproduction in any medium, provided the original work is properly cited.