Open Access
ARTICLE
Leveraging Uncertainty for Depth-Aware Hierarchical Text Classification
1 Chongqing Key Laboratory of Computational Intelligence, Chongqing University of Posts and Telecommunications, Chongqing, 400000, China
2 Division of Emerging Interdisciplinary Areas, Hong Kong University of Science and Technology, Hong Kong, 999077, China
* Corresponding Authors: Ye Wang. Email: ; Hong Yu. Email:
(This article belongs to the Special Issue: Advancements in Natural Language Processing (NLP) and Fuzzy Logic)
Computers, Materials & Continua 2024, 80(3), 4111-4127. https://doi.org/10.32604/cmc.2024.054581
Received 01 June 2024; Accepted 04 August 2024; Issue published 12 September 2024
Abstract
Hierarchical Text Classification (HTC) aims to match text to hierarchical labels. Existing methods overlook two critical issues: first, some texts cannot be fully matched to leaf node labels and need to be classified to the correct parent node instead of treating leaf nodes as the final classification target. Second, error propagation occurs when a misclassification at a parent node propagates down the hierarchy, ultimately leading to inaccurate predictions at the leaf nodes. To address these limitations, we propose an uncertainty-guided HTC depth-aware model called DepthMatch. Specifically, we design an early stopping strategy with uncertainty to identify incomplete matching between text and labels, classifying them into the corresponding parent node labels. This approach allows us to dynamically determine the classification depth by leveraging evidence to quantify and accumulate uncertainty. Experimental results show that the proposed DepthMatch outperforms recent strong baselines on four commonly used public datasets: WOS (Web of Science), RCV1-V2 (Reuters Corpus Volume I), AAPD (Arxiv Academic Paper Dataset), and BGC. Notably, on the BGC dataset, it improves Micro-F1 and Macro-F1 scores by at least 1.09% and 1.74%, respectively.Keywords
Cite This Article
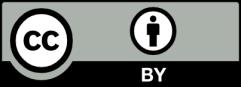
This work is licensed under a Creative Commons Attribution 4.0 International License , which permits unrestricted use, distribution, and reproduction in any medium, provided the original work is properly cited.