Open Access
ARTICLE
Rail-PillarNet: A 3D Detection Network for Railway Foreign Object Based on LiDAR
1 School of Electrical Engineering and Automation, Jiangxi University of Science and Technology, Ganzhou, 341000, China
2 Jiangxi Provincial Key Laboratory of Maglev Technology, Ganzhou, 341000, China
3 School of Information Engineering, Jiangxi University of Science and Technology, Ganzhou, 341000, China
* Corresponding Author: Jie Yang. Email:
(This article belongs to the Special Issue: Artificial Intelligence Driven Innovations in Integrating Communications, Image and Signal Processing Applications)
Computers, Materials & Continua 2024, 80(3), 3819-3833. https://doi.org/10.32604/cmc.2024.054525
Received 30 May 2024; Accepted 01 August 2024; Issue published 12 September 2024
Abstract
Aiming at the limitations of the existing railway foreign object detection methods based on two-dimensional (2D) images, such as short detection distance, strong influence of environment and lack of distance information, we propose Rail-PillarNet, a three-dimensional (3D) LIDAR (Light Detection and Ranging) railway foreign object detection method based on the improvement of PointPillars. Firstly, the parallel attention pillar encoder (PAPE) is designed to fully extract the features of the pillars and alleviate the problem of local fine-grained information loss in PointPillars pillars encoder. Secondly, a fine backbone network is designed to improve the feature extraction capability of the network by combining the coding characteristics of LIDAR point cloud feature and residual structure. Finally, the initial weight parameters of the model were optimised by the transfer learning training method to further improve accuracy. The experimental results on the OSDaR23 dataset show that the average accuracy of Rail-PillarNet reaches 58.51%, which is higher than most mainstream models, and the number of parameters is 5.49 M. Compared with PointPillars, the accuracy of each target is improved by 10.94%, 3.53%, 16.96% and 19.90%, respectively, and the number of parameters only increases by 0.64 M, which achieves a balance between the number of parameters and accuracy.Keywords
Cite This Article
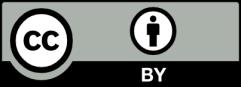
This work is licensed under a Creative Commons Attribution 4.0 International License , which permits unrestricted use, distribution, and reproduction in any medium, provided the original work is properly cited.