Open Access
ARTICLE
Metaheuristic-Driven Two-Stage Ensemble Deep Learning for Lung/Colon Cancer Classification
1 Department of Electrical & Computer Engineering, Valie-e-Asr University of Rafsanjan, Kerman, 7718897111, Iran
2 International Academic Relations Office (IRO), Computer Science Department, University of Halabja, Halabja, 46018, Iraq
3 Department of Computer Engineering, University of Mohaghegh Ardabili, Ardabil, 5619911367, Iran
4 Department of Biomedical Engineering, Islamic Azad University of Mashhad, Mashhad, 9187147578, Iran
5 Department of Electrical & Computer Engineering, Shahid Beheshti University, Tehran, 1983969411, Iran
* Corresponding Author: Mohammad Shokouhifar. Email:
(This article belongs to the Special Issue: Metaheuristic-Driven Optimization Algorithms: Methods and Applications)
Computers, Materials & Continua 2024, 80(3), 3855-3880. https://doi.org/10.32604/cmc.2024.054460
Received 28 May 2024; Accepted 02 August 2024; Issue published 12 September 2024
Abstract
This study investigates the application of deep learning, ensemble learning, metaheuristic optimization, and image processing techniques for detecting lung and colon cancers, aiming to enhance treatment efficacy and improve survival rates. We introduce a metaheuristic-driven two-stage ensemble deep learning model for efficient lung/colon cancer classification. The diagnosis of lung and colon cancers is attempted using several unique indicators by different versions of deep Convolutional Neural Networks (CNNs) in feature extraction and model constructions, and utilizing the power of various Machine Learning (ML) algorithms for final classification. Specifically, we consider different scenarios consisting of two-class colon cancer, three-class lung cancer, and five-class combined lung/colon cancer to conduct feature extraction using four CNNs. These extracted features are then integrated to create a comprehensive feature set. In the next step, the optimization of the feature selection is conducted using a metaheuristic algorithm based on the Electric Eel Foraging Optimization (EEFO). This optimized feature subset is subsequently employed in various ML algorithms to determine the most effective ones through a rigorous evaluation process. The top-performing algorithms are refined using the High-Performance Filter (HPF) and integrated into an ensemble learning framework employing weighted averaging. Our findings indicate that the proposed ensemble learning model significantly surpasses existing methods in classification accuracy across all datasets, achieving accuracies of 99.85% for the two-class, 98.70% for the three-class, and 98.96% for the five-class datasets.Keywords
Cite This Article
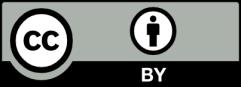
This work is licensed under a Creative Commons Attribution 4.0 International License , which permits unrestricted use, distribution, and reproduction in any medium, provided the original work is properly cited.