Open Access
ARTICLE
An Efficient and Secure Privacy-Preserving Federated Learning Framework Based on Multiplicative Double Privacy Masking
1 College of Cryptography Engineering, Engineering University of PAP, Xi’an, 710086, China
2 Key Laboratory of People’s Armed Police for Cryptology and Information Security, Xi’an, 710086, China
3 College of Information Engineering, Engineering University of PAP, Xi’an, 710086, China
* Corresponding Authors: Cong Shen. Email: ; Wei Zhang. Email:
Computers, Materials & Continua 2024, 80(3), 4729-4748. https://doi.org/10.32604/cmc.2024.054434
Received 28 May 2024; Accepted 16 August 2024; Issue published 12 September 2024
Abstract
With the increasing awareness of privacy protection and the improvement of relevant laws, federal learning has gradually become a new choice for cross-agency and cross-device machine learning. In order to solve the problems of privacy leakage, high computational overhead and high traffic in some federated learning schemes, this paper proposes a multiplicative double privacy mask algorithm which is convenient for homomorphic addition aggregation. The combination of homomorphic encryption and secret sharing ensures that the server cannot compromise user privacy from the private gradient uploaded by the participants. At the same time, the proposed TQRR (Top-Q-Random-R) gradient selection algorithm is used to filter the gradient of encryption and upload efficiently, which reduces the computing overhead of 51.78% and the traffic of 64.87% on the premise of ensuring the accuracy of the model, which makes the framework of privacy protection federated learning lighter to adapt to more miniaturized federated learning terminals.Keywords
Cite This Article
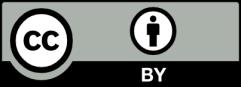
This work is licensed under a Creative Commons Attribution 4.0 International License , which permits unrestricted use, distribution, and reproduction in any medium, provided the original work is properly cited.