Open Access
ARTICLE
Ghost-YOLO v8: An Attention-Guided Enhanced Small Target Detection Algorithm for Floating Litter on Water Surfaces
School of Information Engineering, North China University of Water Resources and Electric Power, Zhengzhou, 450046, China
* Corresponding Author: Shuqing Li. Email:
(This article belongs to the Special Issue: Metaheuristics, Soft Computing, and Machine Learning in Image Processing and Computer Vision)
Computers, Materials & Continua 2024, 80(3), 3713-3731. https://doi.org/10.32604/cmc.2024.054188
Received 21 May 2024; Accepted 27 July 2024; Issue published 12 September 2024
Abstract
Addressing the challenges in detecting surface floating litter in artificial lakes, including complex environments, uneven illumination, and susceptibility to noise and weather, this paper proposes an efficient and lightweight Ghost-YOLO (You Only Look Once) v8 algorithm. The algorithm integrates advanced attention mechanisms and a small-target detection head to significantly enhance detection performance and efficiency. Firstly, an SE (Squeeze-and-Excitation) mechanism is incorporated into the backbone network to fortify the extraction of resilient features and precise target localization. This mechanism models feature channel dependencies, enabling adaptive adjustment of channel importance, thereby improving recognition of floating litter targets. Secondly, a 160 × 160 small-target detection layer is designed in the feature fusion neck to mitigate semantic information loss due to varying target scales. This design enhances the fusion of deep and shallow semantic information, improving small target feature representation and enabling better capture and identification of tiny floating litter. Thirdly, to balance performance and efficiency, the GhostConv module replaces part of the conventional convolutions in the feature fusion neck. Additionally, a novel C2fGhost (CSPDarknet53 to 2-Stage Feature Pyramid Networks Ghost) module is introduced to further reduce network parameters. Lastly, to address the challenge of occlusion, a new loss function, WIoU (Wise Intersection over Union) v3 incorporating a flexible and non-monotonic concentration approach, is adopted to improve detection rates for surface floating litter. The outcomes of the experiments demonstrate that the Ghost-YOLO v8 model proposed in this paper performs well in the dataset Marine, significantly enhances precision and recall by 3.3 and 7.6 percentage points, respectively, in contrast with the base model, mAP@0.5 and mAP@0.5:0.95 improve by 5.3 and 4.4 percentage points and reduces the computational volume by 1.88 MB, the FPS value hardly decreases, and the efficient real-time identification of floating debris on the water’s surface can be achieved cost-effectively.Keywords
Cite This Article
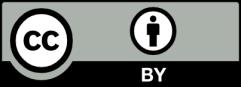
This work is licensed under a Creative Commons Attribution 4.0 International License , which permits unrestricted use, distribution, and reproduction in any medium, provided the original work is properly cited.