Open Access
ARTICLE
A Quarterly High RFM Mining Algorithm for Big Data Management
1 School of International Education, Guangdong University of Technology, Guangzhou, 510006, China
2 School of Computer Science and Technology, Guangdong University of Technology, Guangzhou, 510006, China
3 Business Administration, South China University of Technology, Guangzhou, 510641, China
4 Guangzhou Institute of Technology, Xidian University, Guangzhou, 710071, China
* Corresponding Author: Jiahui Chen. Email:
Computers, Materials & Continua 2024, 80(3), 4341-4360. https://doi.org/10.32604/cmc.2024.054109
Received 19 May 2024; Accepted 09 August 2024; Issue published 12 September 2024
Abstract
In today’s highly competitive retail industry, offline stores face increasing pressure on profitability. They hope to improve their ability in shelf management with the help of big data technology. For this, on-shelf availability is an essential indicator of shelf data management and closely relates to customer purchase behavior. RFM (recency, frequency, and monetary) pattern mining is a powerful tool to evaluate the value of customer behavior. However, the existing RFM pattern mining algorithms do not consider the quarterly nature of goods, resulting in unreasonable shelf availability and difficulty in profit-making. To solve this problem, we propose a quarterly RFM mining algorithm for On-shelf products named OS-RFM. Our algorithm mines the high recency, high frequency, and high monetary patterns and considers the period of the on-shelf goods in quarterly units. We conducted experiments using two real datasets for numerical and graphical analysis to prove the algorithm’s effectiveness. Compared with the state-of-the-art RFM mining algorithm, our algorithm can identify more patterns and performs well in terms of precision, recall, and F1-score, with the recall rate nearing 100%. Also, the novel algorithm operates with significantly shorter running times and more stable memory usage than existing mining algorithms. Additionally, we analyze the sales trends of products in different quarters and seasonal variations. The analysis assists businesses in maintaining reasonable on-shelf availability and achieving greater profitability.Keywords
Cite This Article
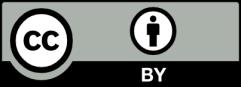
This work is licensed under a Creative Commons Attribution 4.0 International License , which permits unrestricted use, distribution, and reproduction in any medium, provided the original work is properly cited.