Open Access
ARTICLE
A Complex Fuzzy LSTM Network for Temporal-Related Forecasting Problems
1 Institute of Information Technology, Vietnam Academy of Science and Technology, Hoang Quoc Viet, Cau Giay, Hanoi, 100000, Vietnam
2 Academic Affairs Department, Thai Nguyen University of Education, Thai Nguyen, 250000, Vietnam
3 Center of Science and Technology Research and Development, Thuongmai University, Ho Tung Mau, Cau Giay, Hanoi, 100000, Vietnam
4 Faculty of Information Technology, Hanoi University of Industry, Bac Tu Liem, Hanoi, 100000, Vietnam
* Corresponding Author: Cu Nguyen Giap. Email:
Computers, Materials & Continua 2024, 80(3), 4173-4196. https://doi.org/10.32604/cmc.2024.054031
Received 16 May 2024; Accepted 01 August 2024; Issue published 12 September 2024
Abstract
Time-stamped data is fast and constantly growing and it contains significant information thanks to the quick development of management platforms and systems based on the Internet and cutting-edge information communication technologies. Mining the time series data including time series prediction has many practical applications. Many new techniques were developed for use with various types of time series data in the prediction problem. Among those, this work suggests a unique strategy to enhance predicting quality on time-series datasets that the time-cycle matters by fusing deep learning methods with fuzzy theory. In order to increase forecasting accuracy on such type of time-series data, this study proposes integrating deep learning approaches with fuzzy logic. Particularly, it combines the long short-term memory network with the complex fuzzy set theory to create an innovative complex fuzzy long short-term memory model (CFLSTM). The proposed model adds a meaningful representation of the time cycle element thanks to a complex fuzzy set to advance the deep learning long short-term memory (LSTM) technique to have greater power for processing time series data. Experiments on standard common data sets and real-world data sets published in the UCI Machine Learning Repository demonstrated the proposed model’s utility compared to other well-known forecasting models. The results of the comparisons supported the applicability of our proposed strategy for forecasting time series data.Keywords
Cite This Article
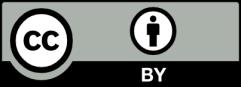
This work is licensed under a Creative Commons Attribution 4.0 International License , which permits unrestricted use, distribution, and reproduction in any medium, provided the original work is properly cited.