Open Access
ARTICLE
Multi-Label Image Classification Based on Object Detection and Dynamic Graph Convolutional Networks
School of Cyber Science and Engineering, Sichuan University, Chengdu, 610207, China
* Corresponding Author: Yong Hu. Email:
Computers, Materials & Continua 2024, 80(3), 4413-4432. https://doi.org/10.32604/cmc.2024.053938
Received 14 May 2024; Accepted 01 August 2024; Issue published 12 September 2024
Abstract
Multi-label image classification is recognized as an important task within the field of computer vision, a discipline that has experienced a significant escalation in research endeavors in recent years. The widespread adoption of convolutional neural networks (CNNs) has catalyzed the remarkable success of architectures such as ResNet-101 within the domain of image classification. However, in multi-label image classification tasks, it is crucial to consider the correlation between labels. In order to improve the accuracy and performance of multi-label classification and fully combine visual and semantic features, many existing studies use graph convolutional networks (GCN) for modeling. Object detection and multi-label image classification exhibit a degree of conceptual overlap; however, the integration of these two tasks within a unified framework has been relatively underexplored in the existing literature. In this paper, we come up with Object-GCN framework, a model combining object detection network YOLOv5 and graph convolutional network, and we carry out a thorough experimental analysis using a range of well-established public datasets. The designed framework Object-GCN achieves significantly better performance than existing studies in public datasets COCO2014, VOC2007, VOC2012. The final results achieved are 86.9%, 96.7%, and 96.3% mean Average Precision (mAP) across the three datasets.Keywords
Cite This Article
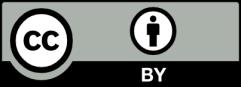
This work is licensed under a Creative Commons Attribution 4.0 International License , which permits unrestricted use, distribution, and reproduction in any medium, provided the original work is properly cited.