Open Access
ARTICLE
IMTNet: Improved Multi-Task Copy-Move Forgery Detection Network with Feature Decoupling and Multi-Feature Pyramid
1 School of Information, Guizhou University of Finance and Economics, Guiyang, 550025, China
2 College of Big Data Statistics, Guizhou University of Finance and Economics, Guiyang, 550025, China
* Corresponding Author: Zhongyuan Jiang. Email:
(This article belongs to the Special Issue: Multimedia Security in Deep Learning)
Computers, Materials & Continua 2024, 80(3), 4603-4620. https://doi.org/10.32604/cmc.2024.053740
Received 09 May 2024; Accepted 13 August 2024; Issue published 12 September 2024
Abstract
Copy-Move Forgery Detection (CMFD) is a technique that is designed to identify image tampering and locate suspicious areas. However, the practicality of the CMFD is impeded by the scarcity of datasets, inadequate quality and quantity, and a narrow range of applicable tasks. These limitations significantly restrict the capacity and applicability of CMFD. To overcome the limitations of existing methods, a novel solution called IMTNet is proposed for CMFD by employing a feature decoupling approach. Firstly, this study formulates the objective task and network relationship as an optimization problem using transfer learning. Furthermore, it thoroughly discusses and analyzes the relationship between CMFD and deep network architecture by employing ResNet-50 during the optimization solving phase. Secondly, a quantitative comparison between fine-tuning and feature decoupling is conducted to evaluate the degree of similarity between the image classification and CMFD domains by the enhanced ResNet-50. Finally, suspicious regions are localized using a feature pyramid network with bottom-up path augmentation. Experimental results demonstrate that IMTNet achieves faster convergence, shorter training times, and favorable generalization performance compared to existing methods. Moreover, it is shown that IMTNet significantly outperforms fine-tuning based approaches in terms of accuracy and F1.Keywords
Cite This Article
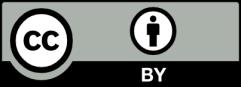
This work is licensed under a Creative Commons Attribution 4.0 International License , which permits unrestricted use, distribution, and reproduction in any medium, provided the original work is properly cited.