Open Access
ARTICLE
Abnormal Action Detection Based on Parameter-Efficient Transfer Learning in Laboratory Scenarios
1 College of Mathematics and Informatics, South China Agricultural University, Guangzhou, 510642, China
2 School of Oceanography, Shanwei Institute of Technology, Shanwei, 516600, China
3 Technical Department, Kerric (Guangdong) Laboratory Equipment Research and Manufacture Co., Ltd., Foshan, 528139, China
* Corresponding Author: Guogang Huang. Email:
Computers, Materials & Continua 2024, 80(3), 4219-4242. https://doi.org/10.32604/cmc.2024.053625
Received 06 May 2024; Accepted 02 August 2024; Issue published 12 September 2024
Abstract
Laboratory safety is a critical area of broad societal concern, particularly in the detection of abnormal actions. To enhance the efficiency and accuracy of detecting such actions, this paper introduces a novel method called TubeRAPT (Tubelet Transformer based on Adapter and Prefix Training Module). This method primarily comprises three key components: the TubeR network, an adaptive clustering attention mechanism, and a prefix training module. These components work in synergy to address the challenge of knowledge preservation in models pre-trained on large datasets while maintaining training efficiency. The TubeR network serves as the backbone for spatio-temporal feature extraction, while the adaptive clustering attention mechanism refines the focus on relevant information. The prefix training module facilitates efficient fine-tuning and knowledge transfer. Experimental results demonstrate the effectiveness of TubeRAPT, achieving a 68.44% mean Average Precision (mAP) on the CLA (Crazy Lab Activity) small-scale dataset, marking a significant improvement of 1.53% over the previous TubeR method. This research not only showcases the potential applications of TubeRAPT in the field of abnormal action detection but also offers innovative ideas and technical support for the future development of laboratory safety monitoring technologies. The proposed method has implications for improving safety management systems in various laboratory environments, potentially reducing accidents and enhancing overall workplace safety.Keywords
Cite This Article
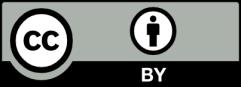
This work is licensed under a Creative Commons Attribution 4.0 International License , which permits unrestricted use, distribution, and reproduction in any medium, provided the original work is properly cited.