Open Access
ARTICLE
GATiT: An Intelligent Diagnosis Model Based on Graph Attention Network Incorporating Text Representation in Knowledge Reasoning
School of Computer and Artificial Intelligence, Zhengzhou University, Zhengzhou, 450001, China
* Corresponding Author: Kunli Zhang. Email:
(This article belongs to the Special Issue: Graph Neural Networks: Methods and Applications in Graph-related Problems)
Computers, Materials & Continua 2024, 80(3), 4767-4790. https://doi.org/10.32604/cmc.2024.053506
Received 02 May 2024; Accepted 16 August 2024; Issue published 12 September 2024
Abstract
The growing prevalence of knowledge reasoning using knowledge graphs (KGs) has substantially improved the accuracy and efficiency of intelligent medical diagnosis. However, current models primarily integrate electronic medical records (EMRs) and KGs into the knowledge reasoning process, ignoring the differing significance of various types of knowledge in EMRs and the diverse data types present in the text. To better integrate EMR text information, we propose a novel intelligent diagnostic model named the Graph ATtention network incorporating Text representation in knowledge reasoning (GATiT), which comprises text representation, subgraph construction, knowledge reasoning, and diagnostic classification. In the text representation process, GATiT uses a pre-trained model to obtain text representations of the EMRs and additionally enhances embeddings by including chief complaint information and numerical information in the input. In the subgraph construction process, GATiT constructs text subgraphs and disease subgraphs from the KG, utilizing EMR text and the disease to be diagnosed. To differentiate the varying importance of nodes within the subgraphs features such as node categories, relevance scores, and other relevant factors are introduced into the text subgraph. The message-passing strategy and attention weight calculation of the graph attention network are adjusted to learn these features in the knowledge reasoning process. Finally, in the diagnostic classification process, the interactive attention-based fusion method integrates the results of knowledge reasoning with text representations to produce the final diagnosis results. Experimental results on multi-label and single-label EMR datasets demonstrate the model’s superiority over several state-of-the-art methods.Keywords
Cite This Article
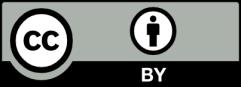
This work is licensed under a Creative Commons Attribution 4.0 International License , which permits unrestricted use, distribution, and reproduction in any medium, provided the original work is properly cited.