Open Access
ARTICLE
Research on Restoration of Murals Based on Diffusion Model and Transformer
1 School of Computing, Hunan University of Technology, Zhuzhou, 412000, China
2 School of Computing, Hunan Software Vocational and Technical University, Xiangtan, 411100, China
* Corresponding Author: Mansheng Xiao. Email:
Computers, Materials & Continua 2024, 80(3), 4433-4449. https://doi.org/10.32604/cmc.2024.053232
Received 28 April 2024; Accepted 03 August 2024; Issue published 12 September 2024
Abstract
Due to the limitations of a priori knowledge and convolution operation, the existing image restoration techniques cannot be directly applied to the cultural relics mural restoration, in order to more accurately restore the original appearance of the cultural relics mural images, an image restoration based on the denoising diffusion probability model (Denoising Diffusion Probability Model (DDPM)) and the Transformer method. The process involves two steps: in the first step, the damaged mural image is firstly utilized as the condition to generate the noise image, using the time, condition and noise image patch as the inputs to the noise prediction network, capturing the global dependencies in the input sequence through the multi-attention mechanism of the input sequence and feed-forward neural network processing, and designing a long skip connection between the shallow and deep layers in the Transformer blocks between the shallow and deep layers using long skip connections to fuse the feature information of global and local outputs to maintain the overall consistency of the restoration results; In the second step, taking the noisy image as a condition to direct the diffusion model to back sample to generate the restored image. Experiment results show that the PSNR and SSIM of the proposed method are improved by 2% to 9% and 1% to 3.3%, respectively, which are compared to the comparison methods. This study proposed synthesizes the advantages of the diffusion model and deep learning model to make the mural restoration results more accurate.Keywords
Cite This Article
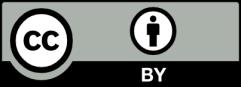
This work is licensed under a Creative Commons Attribution 4.0 International License , which permits unrestricted use, distribution, and reproduction in any medium, provided the original work is properly cited.