Open Access
ARTICLE
RWNeRF: Robust Watermarking Scheme for Neural Radiance Fields Based on Invertible Neural Networks
1 Department of Cryptographic Engineering, Engineering University of PAP, Xi’an, 710086, China
2 Department of Cryptographic Engineering, Key Laboratory of PAP for Cryptology and Information Security, Xi’an, 710086, China
* Corresponding Author: Jia Liu. Email:
(This article belongs to the Special Issue: Multimedia Security in Deep Learning)
Computers, Materials & Continua 2024, 80(3), 4065-4083. https://doi.org/10.32604/cmc.2024.053115
Received 24 April 2024; Accepted 11 July 2024; Issue published 12 September 2024
Abstract
As neural radiance fields continue to advance in 3D content representation, the copyright issues surrounding 3D models oriented towards implicit representation become increasingly pressing. In response to this challenge, this paper treats the embedding and extraction of neural radiance field watermarks as inverse problems of image transformations and proposes a scheme for protecting neural radiance field copyrights using invertible neural network watermarking. Leveraging 2D image watermarking technology for 3D scene protection, the scheme embeds watermarks within the training images of neural radiance fields through the forward process in invertible neural networks and extracts them from images rendered by neural radiance fields through the reverse process, thereby ensuring copyright protection for both the neural radiance fields and associated 3D scenes. However, challenges such as information loss during rendering processes and deliberate tampering necessitate the design of an image quality enhancement module to increase the scheme’s robustness. This module restores distorted images through neural network processing before watermark extraction. Additionally, embedding watermarks in each training image enables watermark information extraction from multiple viewpoints. Our proposed watermarking method achieves a PSNR (Peak Signal-to-Noise Ratio) value exceeding 37 dB for images containing watermarks and 22 dB for recovered watermarked images, as evaluated on the Lego, Hotdog, and Chair datasets, respectively. These results demonstrate the efficacy of our scheme in enhancing copyright protection.Keywords
Cite This Article
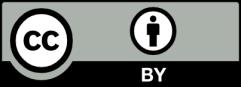
This work is licensed under a Creative Commons Attribution 4.0 International License , which permits unrestricted use, distribution, and reproduction in any medium, provided the original work is properly cited.