Open Access
ARTICLE
Internet of Things Enabled DDoS Attack Detection Using Pigeon Inspired Optimization Algorithm with Deep Learning Approach
Department of Computer Science and Artificial Intelligence, College of Computing, Umm Al-Qura University, Makkah, 21955, Saudi Arabia
* Corresponding Author: Saud S. Alotaibi. Email:
Computers, Materials & Continua 2024, 80(3), 4047-4064. https://doi.org/10.32604/cmc.2024.052796
Received 15 April 2024; Accepted 11 July 2024; Issue published 12 September 2024
Abstract
Internet of Things (IoTs) provides better solutions in various fields, namely healthcare, smart transportation, home, etc. Recognizing Denial of Service (DoS) outbreaks in IoT platforms is significant in certifying the accessibility and integrity of IoT systems. Deep learning (DL) models outperform in detecting complex, non-linear relationships, allowing them to effectually severe slight deviations from normal IoT activities that may designate a DoS outbreak. The uninterrupted observation and real-time detection actions of DL participate in accurate and rapid detection, permitting proactive reduction events to be executed, hence securing the IoT network’s safety and functionality. Subsequently, this study presents pigeon-inspired optimization with a DL-based attack detection and classification (PIODL-ADC) approach in an IoT environment. The PIODL-ADC approach implements a hyperparameter-tuned DL method for Distributed Denial-of-Service (DDoS) attack detection in an IoT platform. Initially, the PIODL-ADC model utilizes Z-score normalization to scale input data into a uniform format. For handling the convolutional and adaptive behaviors of IoT, the PIODL-ADC model employs the pigeon-inspired optimization (PIO) method for feature selection to detect the related features, considerably enhancing the recognition’s accuracy. Also, the Elman Recurrent Neural Network (ERNN) model is utilized to recognize and classify DDoS attacks. Moreover, reptile search algorithm (RSA) based hyperparameter tuning is employed to improve the precision and robustness of the ERNN method. A series of investigational validations is made to ensure the accomplishment of the PIODL-ADC method. The experimental outcome exhibited that the PIODL-ADC method shows greater accomplishment when related to existing models, with a maximum accuracy of 99.81%.Keywords
Cite This Article
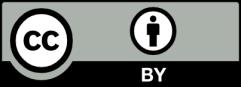
This work is licensed under a Creative Commons Attribution 4.0 International License , which permits unrestricted use, distribution, and reproduction in any medium, provided the original work is properly cited.