Open Access
ARTICLE
Robust and Discriminative Feature Learning via Mutual Information Maximization for Object Detection in Aerial Images
School of Communication Engineering, Jilin University, Changchun, 130012, China
* Corresponding Author: Yinhui Yu. Email:
(This article belongs to the Special Issue: Advanced Artificial Intelligence and Machine Learning Frameworks for Signal and Image Processing Applications)
Computers, Materials & Continua 2024, 80(3), 4149-4171. https://doi.org/10.32604/cmc.2024.052725
Received 12 April 2024; Accepted 06 August 2024; Issue published 12 September 2024
Abstract
Object detection in unmanned aerial vehicle (UAV) aerial images has become increasingly important in military and civil applications. General object detection models are not robust enough against interclass similarity and intraclass variability of small objects, and UAV-specific nuisances such as uncontrolled weather conditions. Unlike previous approaches focusing on high-level semantic information, we report the importance of underlying features to improve detection accuracy and robustness from the information-theoretic perspective. Specifically, we propose a robust and discriminative feature learning approach through mutual information maximization (RD-MIM), which can be integrated into numerous object detection methods for aerial images. Firstly, we present the rank sample mining method to reduce underlying feature differences between the natural image domain and the aerial image domain. Then, we design a momentum contrast learning strategy to make object features similar to the same category and dissimilar to different categories. Finally, we construct a transformer-based global attention mechanism to boost object location semantics by leveraging the high interrelation of different receptive fields. We conduct extensive experiments on the VisDrone and Unmanned Aerial Vehicle Benchmark Object Detection and Tracking (UAVDT) datasets to prove the effectiveness of the proposed method. The experimental results show that our approach brings considerable robustness gains to basic detectors and advanced detection methods, achieving relative growth rates of 51.0% and 39.4% in corruption robustness, respectively. Our code is available at (accessed on 2 August 2024).Keywords
Cite This Article
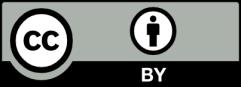
This work is licensed under a Creative Commons Attribution 4.0 International License , which permits unrestricted use, distribution, and reproduction in any medium, provided the original work is properly cited.