Open Access
ARTICLE
Explainable AI-Based DDoS Attacks Classification Using Deep Transfer Learning
1 Department of Computer Science, Jordan University of Science and Technology, Irbid, 22110, Jordan
2 Department of Computer Science, The University of Jordan, Amman, 11942, Jordan
3 Cardiff School of Technologies, Cardiff Metropolitan University, Cardiff, CF5 2YB, UK
4 Prince Abdullah bin Ghazi Faculty of Information and Communication Technology, Al-Balqa Applied University, Salt, 19117, Jordan
* Corresponding Author: Ahmad Alzu’bi. Email:
(This article belongs to the Special Issue: Artificial Intelligence for Addressing Security and Communications Challenges of Internet-connected Critical Infrastructures)
Computers, Materials & Continua 2024, 80(3), 3785-3802. https://doi.org/10.32604/cmc.2024.052599
Received 08 April 2024; Accepted 24 June 2024; Issue published 12 September 2024
Abstract
In the era of the Internet of Things (IoT), the proliferation of connected devices has raised security concerns, increasing the risk of intrusions into diverse systems. Despite the convenience and efficiency offered by IoT technology, the growing number of IoT devices escalates the likelihood of attacks, emphasizing the need for robust security tools to automatically detect and explain threats. This paper introduces a deep learning methodology for detecting and classifying distributed denial of service (DDoS) attacks, addressing a significant security concern within IoT environments. An effective procedure of deep transfer learning is applied to utilize deep learning backbones, which is then evaluated on two benchmarking datasets of DDoS attacks in terms of accuracy and time complexity. By leveraging several deep architectures, the study conducts thorough binary and multiclass experiments, each varying in the complexity of classifying attack types and demonstrating real-world scenarios. Additionally, this study employs an explainable artificial intelligence (XAI) AI technique to elucidate the contribution of extracted features in the process of attack detection. The experimental results demonstrate the effectiveness of the proposed method, achieving a recall of 99.39% by the XAI bidirectional long short-term memory (XAI-BiLSTM) model.Keywords
Cite This Article
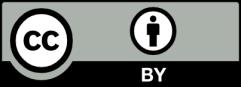
This work is licensed under a Creative Commons Attribution 4.0 International License , which permits unrestricted use, distribution, and reproduction in any medium, provided the original work is properly cited.