Open Access
ARTICLE
Spatial Attention Integrated EfficientNet Architecture for Breast Cancer Classification with Explainable AI
1 Department of Electronics and Communication Engineering, Bannari Amman Institute of Technology, Sathyamangalam, 638402, India
2 School of Computer Science Engineering and Information Systems (SCORE), Vellore Institute of Technology, Vellore, 632014, India
3 School of Science, Engineering and Environment, University of Salford, Manchester, M54WT, UK
4 Department of Computer Science & Engineering, Faculty of Engineering and Technology, JAIN (Deemed-to-be University), Bengaluru, 562112, India
5 Department of Management, College of Business Administration, Princess Nourah Bint Abdulrahman University, P. O. Box 84428, Riyadh, 11671, Saudi Arabia
6 Department of Computer Engineering, College of Computer and Information Sciences, King Saud University, P. O. Box 51178, Riyadh, 11543, Saudi Arabia
7 Adjunct Research Faculty, Centre for Research Impact & Outcome, Chitkara University, Rajpura, 140401, India
* Corresponding Author: Surbhi Bhatia Khan. Email:
(This article belongs to the Special Issue: Privacy-Aware AI-based Models for Cancer Diagnosis)
Computers, Materials & Continua 2024, 80(3), 5029-5045. https://doi.org/10.32604/cmc.2024.052531
Received 04 April 2024; Accepted 11 July 2024; Issue published 12 September 2024
Abstract
Breast cancer is a type of cancer responsible for higher mortality rates among women. The cruelty of breast cancer always requires a promising approach for its earlier detection. In light of this, the proposed research leverages the representation ability of pretrained EfficientNet-B0 model and the classification ability of the XGBoost model for the binary classification of breast tumors. In addition, the above transfer learning model is modified in such a way that it will focus more on tumor cells in the input mammogram. Accordingly, the work proposed an EfficientNet-B0 having a Spatial Attention Layer with XGBoost (ESA-XGBNet) for binary classification of mammograms. For this, the work is trained, tested, and validated using original and augmented mammogram images of three public datasets namely CBIS-DDSM, INbreast, and MIAS databases. Maximum classification accuracy of 97.585% (CBIS-DDSM), 98.255% (INbreast), and 98.91% (MIAS) is obtained using the proposed ESA-XGBNet architecture as compared with the existing models. Furthermore, the decision-making of the proposed ESA-XGBNet architecture is visualized and validated using the Attention Guided GradCAM-based Explainable AI technique.Keywords
Cite This Article
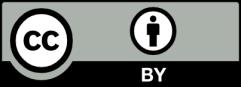
This work is licensed under a Creative Commons Attribution 4.0 International License , which permits unrestricted use, distribution, and reproduction in any medium, provided the original work is properly cited.