Open Access
ARTICLE
Improving Transferable Targeted Adversarial Attack for Object Detection Using RCEN Framework and Logit Loss Optimization
School of Cryptography Engineering, Information Engineering University, Zhengzhou, 450000, China
* Corresponding Author: Lei Sun. Email:
Computers, Materials & Continua 2024, 80(3), 4387-4412. https://doi.org/10.32604/cmc.2024.052196
Received 26 March 2024; Accepted 02 August 2024; Issue published 12 September 2024
Abstract
Object detection finds wide application in various sectors, including autonomous driving, industry, and healthcare. Recent studies have highlighted the vulnerability of object detection models built using deep neural networks when confronted with carefully crafted adversarial examples. This not only reveals their shortcomings in defending against malicious attacks but also raises widespread concerns about the security of existing systems. Most existing adversarial attack strategies focus primarily on image classification problems, failing to fully exploit the unique characteristics of object detection models, thus resulting in widespread deficiencies in their transferability. Furthermore, previous research has predominantly concentrated on the transferability issues of non-targeted attacks, whereas enhancing the transferability of targeted adversarial examples presents even greater challenges. Traditional attack techniques typically employ cross-entropy as a loss measure, iteratively adjusting adversarial examples to match target categories. However, their inherent limitations restrict their broad applicability and transferability across different models. To address the aforementioned challenges, this study proposes a novel targeted adversarial attack method aimed at enhancing the transferability of adversarial samples across object detection models. Within the framework of iterative attacks, we devise a new objective function designed to mitigate consistency issues arising from cumulative noise and to enhance the separation between target and non-target categories (logit margin). Secondly, a data augmentation framework incorporating random erasing and color transformations is introduced into targeted adversarial attacks. This enhances the diversity of gradients, preventing overfitting to white-box models. Lastly, perturbations are applied only within the specified object’s bounding box to reduce the perturbation range, enhancing attack stealthiness. Experiments were conducted on the Microsoft Common Objects in Context (MS COCO) dataset using You Only Look Once version 3 (YOLOv3), You Only Look Once version 8 (YOLOv8), Faster Region-based Convolutional Neural Networks (Faster R-CNN), and RetinaNet. The results demonstrate a significant advantage of the proposed method in black-box settings. Among these, the success rate of RetinaNet transfer attacks reached a maximum of 82.59%.Keywords
Cite This Article
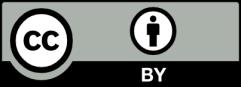
This work is licensed under a Creative Commons Attribution 4.0 International License , which permits unrestricted use, distribution, and reproduction in any medium, provided the original work is properly cited.