Open Access
ARTICLE
Vehicle Head and Tail Recognition Algorithm for Lightweight DCDSNet
1 School of Informatics and Engineering, Suzhou University, Suzhou, 234000, China
2 School of Informatics and Engineering, Suzhou Vocational College of Civil Aviation, Suzhou, 234122, China
3 Institute of Machine Learning and Systems Biology, School of Electronics and Information Engineering, Tongji University, Shanghai, 201804, China
* Corresponding Author: Kaijie Zhang. Email:
(This article belongs to the Special Issue: Metaheuristics, Soft Computing, and Machine Learning in Image Processing and Computer Vision)
Computers, Materials & Continua 2024, 80(3), 4451-4473. https://doi.org/10.32604/cmc.2024.051764
Received 14 March 2024; Accepted 01 July 2024; Issue published 12 September 2024
Abstract
In the model of the vehicle recognition algorithm implemented by the convolutional neural network, the model needs to compute and store a lot of parameters. Too many parameters occupy a lot of computational resources making it difficult to run on computers with poor performance. Therefore, obtaining more efficient feature information of target image or video with better accuracy on computers with limited arithmetic power becomes the main goal of this research. In this paper, a lightweight densely connected, and deeply separable convolutional network (DCDSNet) algorithm is proposed to achieve this goal. Visual Geometry Group (VGG) model is improved by utilizing the convolution instead of the fully connected module, the deeply separable convolution module, and the densely connected network module, with the first two modules reducing the parameters and the third module allowing the algorithm to have more features in a limited number of parameters. The algorithm achieves better results in the mine vehicle recognition dataset. Experiments show that the recognition accuracy is improved by 4.41% compared to VGG19 and the amount of parameters is reduced by 71% compared to VGG19.Keywords
Cite This Article
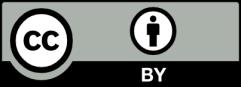
This work is licensed under a Creative Commons Attribution 4.0 International License , which permits unrestricted use, distribution, and reproduction in any medium, provided the original work is properly cited.