Open Access
ARTICLE
A Graph with Adaptive Adjacency Matrix for Relation Extraction
1 Engineering Research Center of Text Computing & Cognitive Intelligence, Ministry of Education, Guizhou University, Guiyang, 550025, China
2 State Key Laboratory of Public Big Data, Guizhou University, Guiyang, 550025, China
3 College of Computer Science and Technology, Guizhou University, Guiyang, 550025, China
* Corresponding Author: Yanping Chen. Email:
Computers, Materials & Continua 2024, 80(3), 4129-4147. https://doi.org/10.32604/cmc.2024.051675
Received 12 March 2024; Accepted 25 July 2024; Issue published 12 September 2024
Abstract
The relation is a semantic expression relevant to two named entities in a sentence. Since a sentence usually contains several named entities, it is essential to learn a structured sentence representation that encodes dependency information specific to the two named entities. In related work, graph convolutional neural networks are widely adopted to learn semantic dependencies, where a dependency tree initializes the adjacency matrix. However, this approach has two main issues. First, parsing a sentence heavily relies on external toolkits, which can be error-prone. Second, the dependency tree only encodes the syntactical structure of a sentence, which may not align with the relational semantic expression. In this paper, we propose an automatic graph learning method to autonomously learn a sentence’s structural information. Instead of using a fixed adjacency matrix initialized by a dependency tree, we introduce an Adaptive Adjacency Matrix to encode the semantic dependency between tokens. The elements of this matrix are dynamically learned during the training process and optimized by task-relevant learning objectives, enabling the construction of task-relevant semantic dependencies within a sentence. Our model demonstrates superior performance on the TACRED and SemEval 2010 datasets, surpassing previous works by 1.3% and 0.8%, respectively. These experimental results show that our model excels in the relation extraction task, outperforming prior models.Keywords
Cite This Article
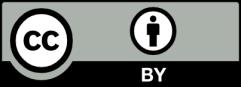
This work is licensed under a Creative Commons Attribution 4.0 International License , which permits unrestricted use, distribution, and reproduction in any medium, provided the original work is properly cited.