Open Access
ARTICLE
Enhancing Log Anomaly Detection with Semantic Embedding and Integrated Neural Network Innovations
School of Computer and Software, Nanjing University of Information Science and Technology, Nanjing, 210044, China
* Corresponding Author: Zhanyang Xu. Email:
(This article belongs to the Special Issue: Advanced Data Mining Techniques: Security, Intelligent Systems and Applications)
Computers, Materials & Continua 2024, 80(3), 3991-4015. https://doi.org/10.32604/cmc.2024.051620
Received 11 March 2024; Accepted 14 July 2024; Issue published 12 September 2024
Abstract
System logs, serving as a pivotal data source for performance monitoring and anomaly detection, play an indispensable role in assuring service stability and reliability. Despite this, the majority of existing log-based anomaly detection methodologies predominantly depend on the sequence or quantity attributes of logs, utilizing solely a single Recurrent Neural Network (RNN) and its variant sequence models for detection. These approaches have not thoroughly exploited the semantic information embedded in logs, exhibit limited adaptability to novel logs, and a single model struggles to fully unearth the potential features within the log sequence. Addressing these challenges, this article proposes a hybrid architecture based on a multiscale convolutional neural network, efficient channel attention and mogrifier gated recurrent unit networks (LogCEM), which amalgamates multiple neural network technologies. Capitalizing on the superior performance of robustly optimized BERT approach (RoBERTa) in the realm of natural language processing, we employ RoBERTa to extract the original word vectors from each word in the log template. In conjunction with the enhanced Smooth Inverse Frequency (SIF) algorithm, we generate more precise log sentence vectors, thereby achieving an in-depth representation of log semantics. Subsequently, these log vector sequences are fed into a hybrid neural network, which fuses 1D Multi-Scale Convolutional Neural Network (MSCNN), Efficient Channel Attention Mechanism (ECA), and Mogrifier Gated Recurrent Unit (GRU). This amalgamation enables the model to concurrently capture the local and global dependencies of the log sequence and autonomously learn the significance of different log sequences, thereby markedly enhancing the efficacy of log anomaly detection. To validate the effectiveness of the LogCEM model, we conducted evaluations on two authoritative open-source datasets. The experimental results demonstrate that LogCEM not only exhibits excellent accuracy and robustness, but also outperforms the current mainstream log anomaly detection methods.Keywords
Cite This Article
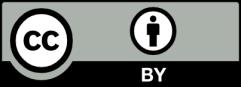
This work is licensed under a Creative Commons Attribution 4.0 International License , which permits unrestricted use, distribution, and reproduction in any medium, provided the original work is properly cited.