Open Access
ARTICLE
Multivariate Time Series Anomaly Detection Based on Spatial-Temporal Network and Transformer in Industrial Internet of Things
1 Information Security Center, State Key Laboratory of Networking and Switching Technology, Beijing University of Posts and Telecommunications, Beijing, 100876, China
2 National Engineering Laboratory for Disaster Backup and Recovery, Beijing University of Posts and Telecommunications, Beijing, 100876, China
3 Department of Information Science and Engineering, Zaozhuang University, Zaozhuang, 277160, China
* Corresponding Author: Haipeng Peng. Email:
Computers, Materials & Continua 2024, 80(2), 2815-2837. https://doi.org/10.32604/cmc.2024.053765
Received 09 May 2024; Accepted 08 July 2024; Issue published 15 August 2024
Abstract
In the Industrial Internet of Things (IIoT), sensors generate time series data to reflect the working state. When the systems are attacked, timely identification of outliers in time series is critical to ensure security. Although many anomaly detection methods have been proposed, the temporal correlation of the time series over the same sensor and the state (spatial) correlation between different sensors are rarely considered simultaneously in these methods. Owing to the superior capability of Transformer in learning time series features. This paper proposes a time series anomaly detection method based on a spatial-temporal network and an improved Transformer. Additionally, the methods based on graph neural networks typically include a graph structure learning module and an anomaly detection module, which are interdependent. However, in the initial phase of training, since neither of the modules has reached an optimal state, their performance may influence each other. This scenario makes the end-to-end training approach hard to effectively direct the learning trajectory of each module. This interdependence between the modules, coupled with the initial instability, may cause the model to find it hard to find the optimal solution during the training process, resulting in unsatisfactory results. We introduce an adaptive graph structure learning method to obtain the optimal model parameters and graph structure. Experiments on two publicly available datasets demonstrate that the proposed method attains higher anomaly detection results than other methods.Keywords
Cite This Article
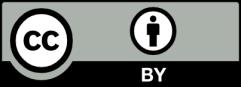
This work is licensed under a Creative Commons Attribution 4.0 International License , which permits unrestricted use, distribution, and reproduction in any medium, provided the original work is properly cited.