Open Access
ARTICLE
A Model for Detecting Fake News by Integrating Domain-Specific Emotional and Semantic Features
1 College of Cryptography Engineering, Engineering University of People’s Armed Police, Xi’an, 710086, China
2 Key Laboratory of Network and Information Security, Engineering University of People’s Armed Police, Xi’an, 710086, China
3 Key Laboratory of CTC & Information Engineering, Engineering University of People’s Armed Police, Ministry of Education, Xi’an, 710086, China
* Corresponding Author: Mingshu Zhang. Email:
(This article belongs to the Special Issue: Security, Privacy, and Robustness for Trustworthy AI Systems)
Computers, Materials & Continua 2024, 80(2), 2161-2179. https://doi.org/10.32604/cmc.2024.053762
Received 09 May 2024; Accepted 17 June 2024; Issue published 15 August 2024
Abstract
With the rapid spread of Internet information and the spread of fake news, the detection of fake news becomes more and more important. Traditional detection methods often rely on a single emotional or semantic feature to identify fake news, but these methods have limitations when dealing with news in specific domains. In order to solve the problem of weak feature correlation between data from different domains, a model for detecting fake news by integrating domain-specific emotional and semantic features is proposed. This method makes full use of the attention mechanism, grasps the correlation between different features, and effectively improves the effect of feature fusion. The algorithm first extracts the semantic features of news text through the Bi-LSTM (Bidirectional Long Short-Term Memory) layer to capture the contextual relevance of news text. Senta-BiLSTM is then used to extract emotional features and predict the probability of positive and negative emotions in the text. It then uses domain features as an enhancement feature and attention mechanism to fully capture more fine-grained emotional features associated with that domain. Finally, the fusion features are taken as the input of the fake news detection classifier, combined with the multi-task representation of information, and the MLP and Softmax functions are used for classification. The experimental results show that on the Chinese dataset Weibo21, the F1 value of this model is 0.958, 4.9% higher than that of the sub-optimal model; on the English dataset FakeNewsNet, the F1 value of the detection result of this model is 0.845, 1.8% higher than that of the sub-optimal model, which is advanced and feasible.Keywords
Cite This Article
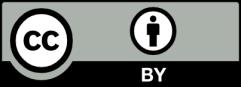
This work is licensed under a Creative Commons Attribution 4.0 International License , which permits unrestricted use, distribution, and reproduction in any medium, provided the original work is properly cited.