Open Access
ARTICLE
GDMNet: A Unified Multi-Task Network for Panoptic Driving Perception
School of Computer Science and Information Engineering, Shanghai Institute of Technology, Shanghai, 201418, China
* Corresponding Author: Jianlin Zhu. Email:
(This article belongs to the Special Issue: Metaheuristics, Soft Computing, and Machine Learning in Image Processing and Computer Vision)
Computers, Materials & Continua 2024, 80(2), 2963-2978. https://doi.org/10.32604/cmc.2024.053710
Received 08 May 2024; Accepted 08 July 2024; Issue published 15 August 2024
Abstract
To enhance the efficiency and accuracy of environmental perception for autonomous vehicles, we propose GDMNet, a unified multi-task perception network for autonomous driving, capable of performing drivable area segmentation, lane detection, and traffic object detection. Firstly, in the encoding stage, features are extracted, and Generalized Efficient Layer Aggregation Network (GELAN) is utilized to enhance feature extraction and gradient flow. Secondly, in the decoding stage, specialized detection heads are designed; the drivable area segmentation head employs DySample to expand feature maps, the lane detection head merges early-stage features and processes the output through the Focal Modulation Network (FMN). Lastly, the Minimum Point Distance IoU (MPDIoU) loss function is employed to compute the matching degree between traffic object detection boxes and predicted boxes, facilitating model training adjustments. Experimental results on the BDD100K dataset demonstrate that the proposed network achieves a drivable area segmentation mean intersection over union (mIoU) of 92.2%, lane detection accuracy and intersection over union (IoU) of 75.3% and 26.4%, respectively, and traffic object detection recall and mAP of 89.7% and 78.2%, respectively. The detection performance surpasses that of other single-task or multi-task algorithm models.Keywords
Cite This Article
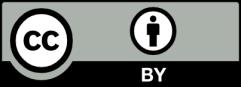
This work is licensed under a Creative Commons Attribution 4.0 International License , which permits unrestricted use, distribution, and reproduction in any medium, provided the original work is properly cited.