Open Access
ARTICLE
CAEFusion: A New Convolutional Autoencoder-Based Infrared and Visible Light Image Fusion Algorithm
1 Key Laboratory of Modern Power System Simulation and Control & Renewable Energy Technology, Ministry of Education, School of Electrical Engineering, Northeast Electric Power University, Jilin, 132012, China
2 School of Electrical Engineering, Northeast Electric Power University, Jilin, 132012, China
3 School of Electronic Information Engineering, Bozhou University, Bozhou, 236800, China
* Corresponding Author: Mei-Ling Ren. Email:
(This article belongs to the Special Issue: Artificial Intelligence Driven Innovations in Integrating Communications, Image and Signal Processing Applications)
Computers, Materials & Continua 2024, 80(2), 2857-2872. https://doi.org/10.32604/cmc.2024.053708
Received 08 May 2024; Accepted 07 July 2024; Issue published 15 August 2024
Abstract
To address the issues of incomplete information, blurred details, loss of details, and insufficient contrast in infrared and visible image fusion, an image fusion algorithm based on a convolutional autoencoder is proposed. The region attention module is meant to extract the background feature map based on the distinct properties of the background feature map and the detail feature map. A multi-scale convolution attention module is suggested to enhance the communication of feature information. At the same time, the feature transformation module is introduced to learn more robust feature representations, aiming to preserve the integrity of image information. This study uses three available datasets from TNO, FLIR, and NIR to perform thorough quantitative and qualitative trials with five additional algorithms. The methods are assessed based on four indicators: information entropy (EN), standard deviation (SD), spatial frequency (SF), and average gradient (AG). Object detection experiments were done on the M3FD dataset to further verify the algorithm’s performance in comparison with five other algorithms. The algorithm’s accuracy was evaluated using the mean average precision at a threshold of 0.5 (mAP@0.5) index. Comprehensive experimental findings show that CAEFusion performs well in subjective visual and objective evaluation criteria and has promising potential in downstream object detection tasks.Keywords
Cite This Article
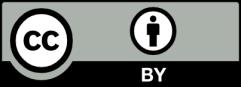
This work is licensed under a Creative Commons Attribution 4.0 International License , which permits unrestricted use, distribution, and reproduction in any medium, provided the original work is properly cited.