Open Access
REVIEW
Deep Transfer Learning Techniques in Intrusion Detection System-Internet of Vehicles: A State-of-the-Art Review
1 School of Information Engineering, Nanchang University, Nanchang, 330031, China
2 Department of Computer Engineering, Technical and Vocational University (TVU), Tehran, 4631964198, Iran
3 Department of Computer Engineering, University of Birjand, Birjand, 9717434765, Iran
4 Department of Computer Engineering, Babol Branch, Islamic Azad University, Babol, 3738147471, Iran
5 Department of Electronics & Communication Engineering, Mepco Schlenk Engineering College, Sivakasi, 626 005, India
6 School of Electronics Engineering, VIT-AP University, Near Vijayawada, 522 237, India
7 Faculty of Mathematics and Informatics, University of Plovdiv Paisii Hilendarski, Plovdiv, 4000, Bulgaria
8 Examination Branch, Dibrugarh University, Dibrugarh, 786004, India
9 Department of Computer Engineering, Faculty of Engineering, University of Birjand, Birjand, 9717434765, Iran
10 Faculty of Electrical and Computer Engineering, University of Birjand, Birjand, 9717434765, Iran
11 Malaysian Institute of Information Technology, Universiti Kuala Lumpur, Kuala Lumpur, 50250, Malaysia
12 Faculty of Physics and Technology, University of Plovdiv Paisii Hilendarski, Plovdiv, 4000, Bulgaria
* Corresponding Author: Silvia Gaftandzhieva. Email:
(This article belongs to the Special Issue: Advanced Communication and Networking Technologies for Internet of Things and Internet of Vehicles)
Computers, Materials & Continua 2024, 80(2), 2785-2813. https://doi.org/10.32604/cmc.2024.053037
Received 23 April 2024; Accepted 09 July 2024; Issue published 15 August 2024
Abstract
The high performance of IoT technology in transportation networks has led to the increasing adoption of Internet of Vehicles (IoV) technology. The functional advantages of IoV include online communication services, accident prevention, cost reduction, and enhanced traffic regularity. Despite these benefits, IoV technology is susceptible to cyber-attacks, which can exploit vulnerabilities in the vehicle network, leading to perturbations, disturbances, non-recognition of traffic signs, accidents, and vehicle immobilization. This paper reviews the state-of-the-art achievements and developments in applying Deep Transfer Learning (DTL) models for Intrusion Detection Systems in the Internet of Vehicles (IDS-IoV) based on anomaly detection. IDS-IoV leverages anomaly detection through machine learning and DTL techniques to mitigate the risks posed by cyber-attacks. These systems can autonomously create specific models based on network data to differentiate between regular traffic and cyber-attacks. Among these techniques, transfer learning models are particularly promising due to their efficacy with tagged data, reduced training time, lower memory usage, and decreased computational complexity. We evaluate DTL models against criteria including the ability to transfer knowledge, detection rate, accurate analysis of complex data, and stability. This review highlights the significant progress made in the field, showcasing how DTL models enhance the performance and reliability of IDS-IoV systems. By examining recent advancements, we provide insights into how DTL can effectively address cyber-attack challenges in IoV environments, ensuring safer and more efficient transportation networks.Keywords
Cite This Article
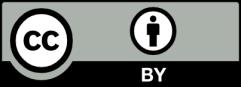
This work is licensed under a Creative Commons Attribution 4.0 International License , which permits unrestricted use, distribution, and reproduction in any medium, provided the original work is properly cited.