Open Access
ARTICLE
Blockchain-Enabled Federated Learning for Privacy-Preserving Non-IID Data Sharing in Industrial Internet
School of Electrical and Information Engineering, Hunan Institute of Technology, Hengyang, 421002, China
* Corresponding Author: Haibing Dong. Email:
(This article belongs to the Special Issue: AI and Data Security for the Industrial Internet)
Computers, Materials & Continua 2024, 80(2), 1967-1983. https://doi.org/10.32604/cmc.2024.052775
Received 15 April 2024; Accepted 19 June 2024; Issue published 15 August 2024
Abstract
Sharing data while protecting privacy in the industrial Internet is a significant challenge. Traditional machine learning methods require a combination of all data for training; however, this approach can be limited by data availability and privacy concerns. Federated learning (FL) has gained considerable attention because it allows for decentralized training on multiple local datasets. However, the training data collected by data providers are often non-independent and identically distributed (non-IID), resulting in poor FL performance. This paper proposes a privacy-preserving approach for sharing non-IID data in the industrial Internet using an FL approach based on blockchain technology. To overcome the problem of non-IID data leading to poor training accuracy, we propose dynamically updating the local model based on the divergence of the global and local models. This approach can significantly improve the accuracy of FL training when there is relatively large dispersion. In addition, we design a dynamic gradient clipping algorithm to alleviate the influence of noise on the model accuracy to reduce potential privacy leakage caused by sharing model parameters. Finally, we evaluate the performance of the proposed scheme using commonly used open-source image datasets. The simulation results demonstrate that our method can significantly enhance the accuracy while protecting privacy and maintaining efficiency, thereby providing a new solution to data-sharing and privacy-protection challenges in the industrial Internet.Keywords
Cite This Article
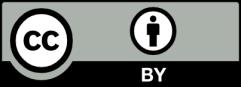
This work is licensed under a Creative Commons Attribution 4.0 International License , which permits unrestricted use, distribution, and reproduction in any medium, provided the original work is properly cited.