Open Access
ARTICLE
Improving Diversity with Multi-Loss Adversarial Training in Personalized News Recommendation
1 School of Computer and Cyber Sciences, Communication University of China, Beijing, 100024, China
2 State Key Laboratory of Media Convergence and Communication, Communication University of China, Beijing, 100024, China
* Corresponding Author: Shuang Feng. Email:
Computers, Materials & Continua 2024, 80(2), 3107-3122. https://doi.org/10.32604/cmc.2024.052600
Received 08 April 2024; Accepted 18 July 2024; Issue published 15 August 2024
Abstract
Users’ interests are often diverse and multi-grained, with their underlying intents even more so. Effectively capturing users’ interests and uncovering the relationships between diverse interests are key to news recommendation. Meanwhile, diversity is an important metric for evaluating news recommendation algorithms, as users tend to reject excessive homogeneous information in their recommendation lists. However, recommendation models themselves lack diversity awareness, making it challenging to achieve a good balance between the accuracy and diversity of news recommendations. In this paper, we propose a news recommendation algorithm that achieves good performance in both accuracy and diversity. Unlike most existing works that solely optimize accuracy or employ more features to meet diversity, the proposed algorithm leverages the diversity-aware capability of the model. First, we introduce an augmented user model to fully capture user intent and the behavioral guidance they might undergo as a result. Specifically, we focus on the relationship between the original clicked news and the augmented clicked news. Moreover, we propose an effective adversarial training method for diversity (AT4D), which is a pluggable component that can enhance both the accuracy and diversity of news recommendation results. Extensive experiments on real-world datasets confirm the efficacy of the proposed algorithm in improving both the accuracy and diversity of news recommendations.Keywords
Cite This Article
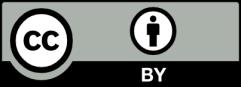
This work is licensed under a Creative Commons Attribution 4.0 International License , which permits unrestricted use, distribution, and reproduction in any medium, provided the original work is properly cited.