Open Access
ARTICLE
ConvNeXt-UperNet-Based Deep Learning Model for Road Extraction from High-Resolution Remote Sensing Images
1 Geographic Information and Tourism College, Chuzhou University, Chuzhou, 239000, China
2 Anhui Province Key Laboratory of Physical Geographic Environment, Chuzhou University, Chuzhou, 239000, China
* Corresponding Author: Jing Wang. Email:
Computers, Materials & Continua 2024, 80(2), 1907-1925. https://doi.org/10.32604/cmc.2024.052597
Received 08 April 2024; Accepted 12 June 2024; Issue published 15 August 2024
Abstract
When existing deep learning models are used for road extraction tasks from high-resolution images, they are easily affected by noise factors such as tree and building occlusion and complex backgrounds, resulting in incomplete road extraction and low accuracy. We propose the introduction of spatial and channel attention modules to the convolutional neural network ConvNeXt. Then, ConvNeXt is used as the backbone network, which cooperates with the perceptual analysis network UPerNet, retains the detection head of the semantic segmentation, and builds a new model ConvNeXt-UPerNet to suppress noise interference. Training on the open-source DeepGlobe and CHN6-CUG datasets and introducing the DiceLoss on the basis of CrossEntropyLoss solves the problem of positive and negative sample imbalance. Experimental results show that the new network model can achieve the following performance on the DeepGlobe dataset: 79.40% for precision (Pre), 97.93% for accuracy (Acc), 69.28% for intersection over union (IoU), and 83.56% for mean intersection over union (MIoU). On the CHN6-CUG dataset, the model achieves the respective values of 78.17% for Pre, 97.63% for Acc, 65.4% for IoU, and 81.46% for MIoU. Compared with other network models, the fused ConvNeXt-UPerNet model can extract road information better when faced with the influence of noise contained in high-resolution remote sensing images. It also achieves multiscale image feature information with unified perception, ultimately improving the generalization ability of deep learning technology in extracting complex roads from high-resolution remote sensing images.Keywords
Cite This Article
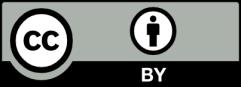
This work is licensed under a Creative Commons Attribution 4.0 International License , which permits unrestricted use, distribution, and reproduction in any medium, provided the original work is properly cited.