Open Access
ARTICLE
Colorectal Cancer Segmentation Algorithm Based on Deep Features from Enhanced CT Images
1 School of Biomedical Engineering, Fourth Military Medical University, Xi’an, 710119, China
2 Department of Radiology, Xijing Hospital, Fourth Military Medical University, Xi’an, 710032, China
3 Department of Radiology, The First Affiliated Hospital of Xi’an Jiaotong University, Xi’an, 710061, China
4 School of Computer Science and Engineering, North Minzu University, Yinchuan, 750030, China
* Corresponding Author: Hongbing Lu. Email:
(This article belongs to the Special Issue: Deep Learning in Computer-Aided Diagnosis Based on Medical Image)
Computers, Materials & Continua 2024, 80(2), 2495-2510. https://doi.org/10.32604/cmc.2024.052476
Received 02 April 2024; Accepted 03 July 2024; Issue published 15 August 2024
Abstract
Colorectal cancer, a malignant lesion of the intestines, significantly affects human health and life, emphasizing the necessity of early detection and treatment. Accurate segmentation of colorectal cancer regions directly impacts subsequent staging, treatment methods, and prognostic outcomes. While colonoscopy is an effective method for detecting colorectal cancer, its data collection approach can cause patient discomfort. To address this, current research utilizes Computed Tomography (CT) imaging; however, conventional CT images only capture transient states, lacking sufficient representational capability to precisely locate colorectal cancer. This study utilizes enhanced CT images, constructing a deep feature network from the arterial, portal venous, and delay phases to simulate the physician’s diagnostic process and achieve accurate cancer segmentation. The innovations include: 1) Utilizing portal venous phase CT images to introduce a context-aware multi-scale aggregation module for preliminary shape extraction of colorectal cancer. 2) Building an image sequence based on arterial and delay phases, transforming the cancer segmentation issue into an anomaly detection problem, establishing a pixel-pairing strategy, and proposing a colorectal cancer segmentation algorithm using a Siamese network. Experiments with 84 clinical cases of colorectal cancer enhanced CT data demonstrated an Area Overlap Measure of 0.90, significantly better than Fully Convolutional Networks (FCNs) at 0.20. Future research will explore the relationship between conventional and enhanced CT to further reduce segmentation time and improve accuracy.Keywords
Cite This Article
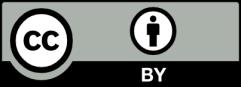
This work is licensed under a Creative Commons Attribution 4.0 International License , which permits unrestricted use, distribution, and reproduction in any medium, provided the original work is properly cited.