Open Access
ARTICLE
Dynamic Forecasting of Traffic Event Duration in Istanbul: A Classification Approach with Real-Time Data Integration
1 Occupational Health and Safety Department, Bandirma Onyedi Eylul University, Balikesir, 10200, Türkiye
2 Department of Industrial Engineering, Istanbul University-Cerrahpasa, Istanbul, 34320, Türkiye
3 Department of Industrial Engineering, Istanbul Technical University, Istanbul, 34467, Türkiye
* Corresponding Author: Mesut Ulu. Email:
Computers, Materials & Continua 2024, 80(2), 2259-2281. https://doi.org/10.32604/cmc.2024.052323
Received 30 March 2024; Accepted 21 June 2024; Issue published 15 August 2024
Abstract
Today, urban traffic, growing populations, and dense transportation networks are contributing to an increase in traffic incidents. These incidents include traffic accidents, vehicle breakdowns, fires, and traffic disputes, resulting in long waiting times, high carbon emissions, and other undesirable situations. It is vital to estimate incident response times quickly and accurately after traffic incidents occur for the success of incident-related planning and response activities. This study presents a model for forecasting the traffic incident duration of traffic events with high precision. The proposed model goes through a 4-stage process using various features to predict the duration of four different traffic events and presents a feature reduction approach to enable real-time data collection and prediction. In the first stage, the dataset consisting of 24,431 data points and 75 variables is prepared by data collection, merging, missing data processing and data cleaning. In the second stage, models such as Decision Trees (DT), K-Nearest Neighbour (KNN), Random Forest (RF) and Support Vector Machines (SVM) are used and hyperparameter optimisation is performed with GridSearchCV. In the third stage, feature selection and reduction are performed and real-time data are used. In the last stage, model performance with 14 variables is evaluated with metrics such as accuracy, precision, recall, F1-score, MCC, confusion matrix and SHAP. The RF model outperforms other models with an accuracy of 98.5%. The study’s prediction results demonstrate that the proposed dynamic prediction model can achieve a high level of success.Keywords
Cite This Article
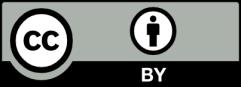
This work is licensed under a Creative Commons Attribution 4.0 International License , which permits unrestricted use, distribution, and reproduction in any medium, provided the original work is properly cited.