Open Access
ARTICLE
ED-Ged: Nighttime Image Semantic Segmentation Based on Enhanced Detail and Bidirectional Guidance
College of Computer Science and Engineering, Chongqing University of Technology, Chongqing, 400054, China
* Corresponding Author: Jianxun Zhang. Email:
(This article belongs to the Special Issue: Metaheuristics, Soft Computing, and Machine Learning in Image Processing and Computer Vision)
Computers, Materials & Continua 2024, 80(2), 2443-2462. https://doi.org/10.32604/cmc.2024.052285
Received 28 March 2024; Accepted 27 June 2024; Issue published 15 August 2024
Abstract
Semantic segmentation of driving scene images is crucial for autonomous driving. While deep learning technology has significantly improved daytime image semantic segmentation, nighttime images pose challenges due to factors like poor lighting and overexposure, making it difficult to recognize small objects. To address this, we propose an Image Adaptive Enhancement (IAEN) module comprising a parameter predictor (Edip), multiple image processing filters (Mdif), and a Detail Processing Module (DPM). Edip combines image processing filters to predict parameters like exposure and hue, optimizing image quality. We adopt a novel image encoder to enhance parameter prediction accuracy by enabling Edip to handle features at different scales. DPM strengthens overlooked image details, extending the IAEN module’s functionality. After the segmentation network, we integrate a Depth Guided Filter (DGF) to refine segmentation outputs. The entire network is trained end-to-end, with segmentation results guiding parameter prediction optimization, promoting self-learning and network improvement. This lightweight and efficient network architecture is particularly suitable for addressing challenges in nighttime image segmentation. Extensive experiments validate significant performance improvements of our approach on the ACDC-night and Nightcity datasets.Keywords
Cite This Article
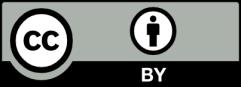
This work is licensed under a Creative Commons Attribution 4.0 International License , which permits unrestricted use, distribution, and reproduction in any medium, provided the original work is properly cited.