Open Access
ARTICLE
CMMCAN: Lightweight Feature Extraction and Matching Network for Endoscopic Images Based on Adaptive Attention
1 School of Electronic and Information Engineering, Hebei University of Technology, Tianjin, 300401, China
2 School of Information and Intelligence Engineering, Tianjin Renai College, Tianjin, 301636, China
* Corresponding Author: Nannan Chong. Email:
(This article belongs to the Special Issue: Multimodal Learning in Image Processing)
Computers, Materials & Continua 2024, 80(2), 2761-2783. https://doi.org/10.32604/cmc.2024.052217
Received 26 March 2024; Accepted 24 June 2024; Issue published 15 August 2024
Abstract
In minimally invasive surgery, endoscopes or laparoscopes equipped with miniature cameras and tools are used to enter the human body for therapeutic purposes through small incisions or natural cavities. However, in clinical operating environments, endoscopic images often suffer from challenges such as low texture, uneven illumination, and non-rigid structures, which affect feature observation and extraction. This can severely impact surgical navigation or clinical diagnosis due to missing feature points in endoscopic images, leading to treatment and postoperative recovery issues for patients. To address these challenges, this paper introduces, for the first time, a Cross-Channel Multi-Modal Adaptive Spatial Feature Fusion (ASFF) module based on the lightweight architecture of EfficientViT. Additionally, a novel lightweight feature extraction and matching network based on attention mechanism is proposed. This network dynamically adjusts attention weights for cross-modal information from grayscale images and optical flow images through a dual-branch Siamese network. It extracts static and dynamic information features ranging from low-level to high-level, and from local to global, ensuring robust feature extraction across different widths, noise levels, and blur scenarios. Global and local matching are performed through a multi-level cascaded attention mechanism, with cross-channel attention introduced to simultaneously extract low-level and high-level features. Extensive ablation experiments and comparative studies are conducted on the HyperKvasir, EAD, M2caiSeg, CVC-ClinicDB, and UCL synthetic datasets. Experimental results demonstrate that the proposed network improves upon the baseline EfficientViT-B3 model by 75.4% in accuracy (Acc), while also enhancing runtime performance and storage efficiency. When compared with the complex DenseDescriptor feature extraction network, the difference in Acc is less than 7.22%, and IoU calculation results on specific datasets outperform complex dense models. Furthermore, this method increases the F1 score by 33.2% and accelerates runtime by 70.2%. It is noteworthy that the speed of CMMCAN surpasses that of comparative lightweight models, with feature extraction and matching performance comparable to existing complex models but with faster speed and higher cost-effectiveness.Keywords
Cite This Article
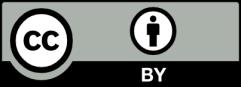
This work is licensed under a Creative Commons Attribution 4.0 International License , which permits unrestricted use, distribution, and reproduction in any medium, provided the original work is properly cited.