Open Access
ARTICLE
Attention Guided Food Recognition via Multi-Stage Local Feature Fusion
School of Electrical and Electronic Engineering, Wuhan Polytechnic University, Wuhan, 430048, China
* Corresponding Author: Weizhen Chen. Email:
(This article belongs to the Special Issue: Metaheuristics, Soft Computing, and Machine Learning in Image Processing and Computer Vision)
Computers, Materials & Continua 2024, 80(2), 1985-2003. https://doi.org/10.32604/cmc.2024.052174
Received 25 March 2024; Accepted 18 June 2024; Issue published 15 August 2024
Abstract
The task of food image recognition, a nuanced subset of fine-grained image recognition, grapples with substantial intra-class variation and minimal inter-class differences. These challenges are compounded by the irregular and multi-scale nature of food images. Addressing these complexities, our study introduces an advanced model that leverages multiple attention mechanisms and multi-stage local fusion, grounded in the ConvNeXt architecture. Our model employs hybrid attention (HA) mechanisms to pinpoint critical discriminative regions within images, substantially mitigating the influence of background noise. Furthermore, it introduces a multi-stage local fusion (MSLF) module, fostering long-distance dependencies between feature maps at varying stages. This approach facilitates the assimilation of complementary features across scales, significantly bolstering the model’s capacity for feature extraction. Furthermore, we constructed a dataset named Roushi60, which consists of 60 different categories of common meat dishes. Empirical evaluation of the ETH Food-101, ChineseFoodNet, and Roushi60 datasets reveals that our model achieves recognition accuracies of 91.12%, 82.86%, and 92.50%, respectively. These figures not only mark an improvement of 1.04%, 3.42%, and 1.36% over the foundational ConvNeXt network but also surpass the performance of most contemporary food image recognition methods. Such advancements underscore the efficacy of our proposed model in navigating the intricate landscape of food image recognition, setting a new benchmark for the field.Keywords
Cite This Article
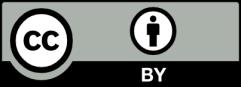
This work is licensed under a Creative Commons Attribution 4.0 International License , which permits unrestricted use, distribution, and reproduction in any medium, provided the original work is properly cited.